Improved Active and Reactive Energy Forecasting Using a Stacking Ensemble Approach: Steel Industry Case Study
Energies(2023)
摘要
The prevalence of substantial inductive/capacitive loads within the industrial sectors induces variations in reactive energy levels. The imbalance between active and reactive energy within the network leads to heightened losses, diminished network efficiency, and an associated escalation in operating costs. Therefore, the forecasting of active and reactive energy in the industrial sector confers notable advantages, including cost reduction, heightened operational efficiency, safeguarding of equipment, enhanced energy consumption management, and more effective assimilation of renewable energy sources. Consequently, a range of specialized forecasting methods for different applications have been developed to address these challenges effectively. This research proposes a stacked ensemble methodology, denoted as Stack-XGBoost, leveraging three distinct machine learning (ML) methods: extra trees regressor (ETR), adaptive boosting (AdaBoost), and random forest regressor (RFR), as foundational models. Moreover, the incorporation of an extreme gradient boosting (XGBoost) algorithm as a meta-learner serves to amalgamate the predictions generated by the base models, enhancing the precision of the active/reactive energy consumption forecasting using real time data for steel industry. To assess the efficacy of the proposed model, diverse performance metrics were employed. The results show that the proposed Stack-XGBoost model outperformed other forecasting methods. Additionally, a sensitivity analysis was conducted to assess the robustness of the proposed method against variations in input parameters.
更多查看译文
关键词
active/reactive energy forecasting,industrial energy consumption,short-term forecasting,energy management,machine learning,ensemble model
AI 理解论文
溯源树
样例
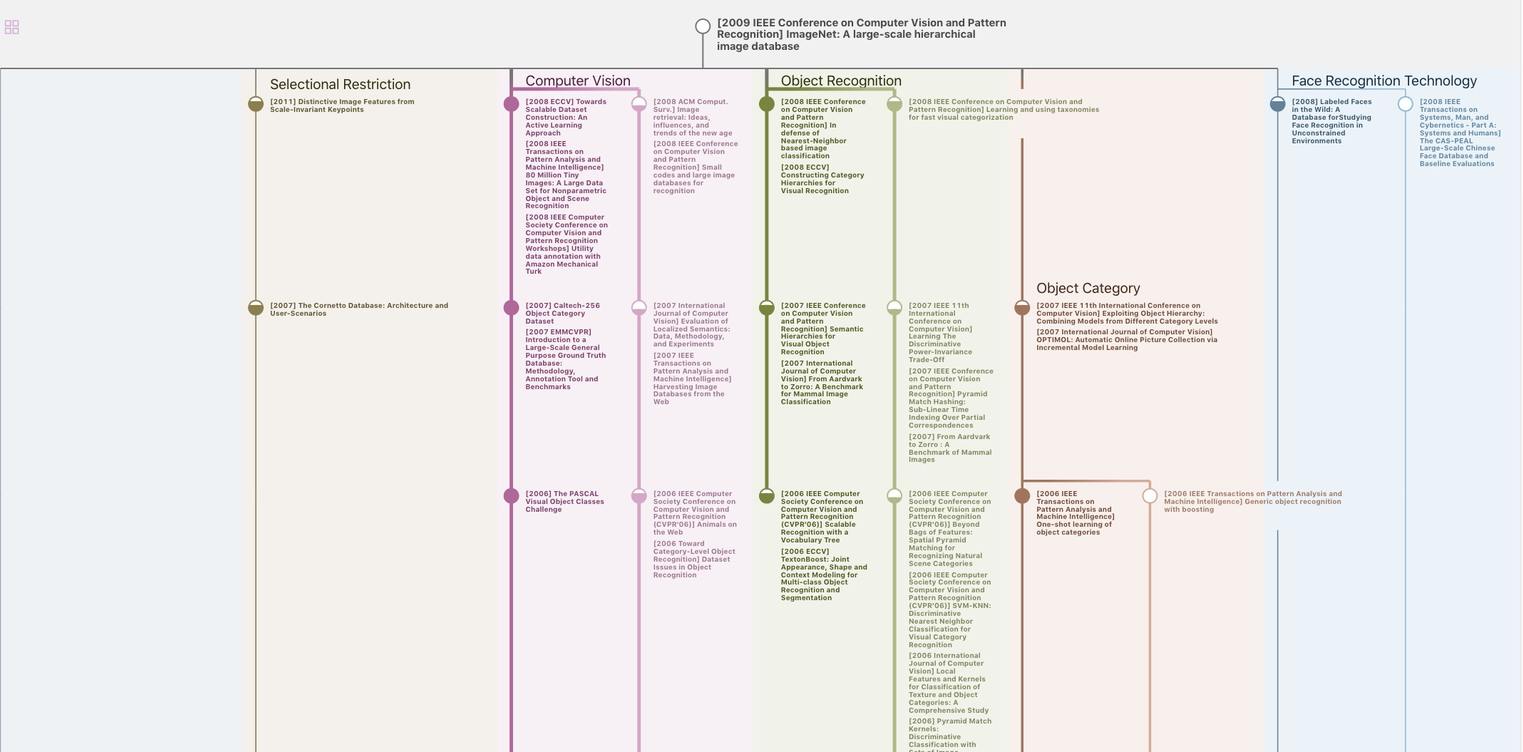
生成溯源树,研究论文发展脉络
Chat Paper
正在生成论文摘要