LWR-Net: Robust and Lightweight Place Recognition Network for Noisy and Low-Density Point Clouds
Sensors(2023)
摘要
Point cloud-based retrieval for place recognition is essential in robotic applications like autonomous driving or simultaneous localization and mapping. However, this remains challenging in complex real-world scenes. Existing methods are sensitive to noisy, low-density point clouds and require extensive storage and computation, posing limitations for hardware-limited scenarios. To overcome these challenges, we propose LWR-Net, a lightweight place recognition network for efficient and robust point cloud retrieval in noisy, low-density conditions. Our approach incorporates a fast dilated sampling and grouping module with a residual MLP structure to learn geometric features from local neighborhoods. We also introduce a lightweight attentional weighting module to enhance global feature representation. By utilizing the Generalized Mean pooling structure, we aggregated the global descriptor for point cloud retrieval. We validated LWR-Net's efficiency and robustness on the Oxford robotcar dataset and three in-house datasets. The results demonstrate that our method efficiently and accurately retrieves matching scenes while being more robust to variations in point density and noise intensity. LWR-Net achieves state-of-the-art accuracy and robustness with a lightweight model size of 0.4M parameters. These efficiency, robustness, and lightweight advantages make our network highly suitable for robotic applications relying on point cloud-based place recognition.
更多查看译文
关键词
lightweight place recognition network,lwr-net,low-density
AI 理解论文
溯源树
样例
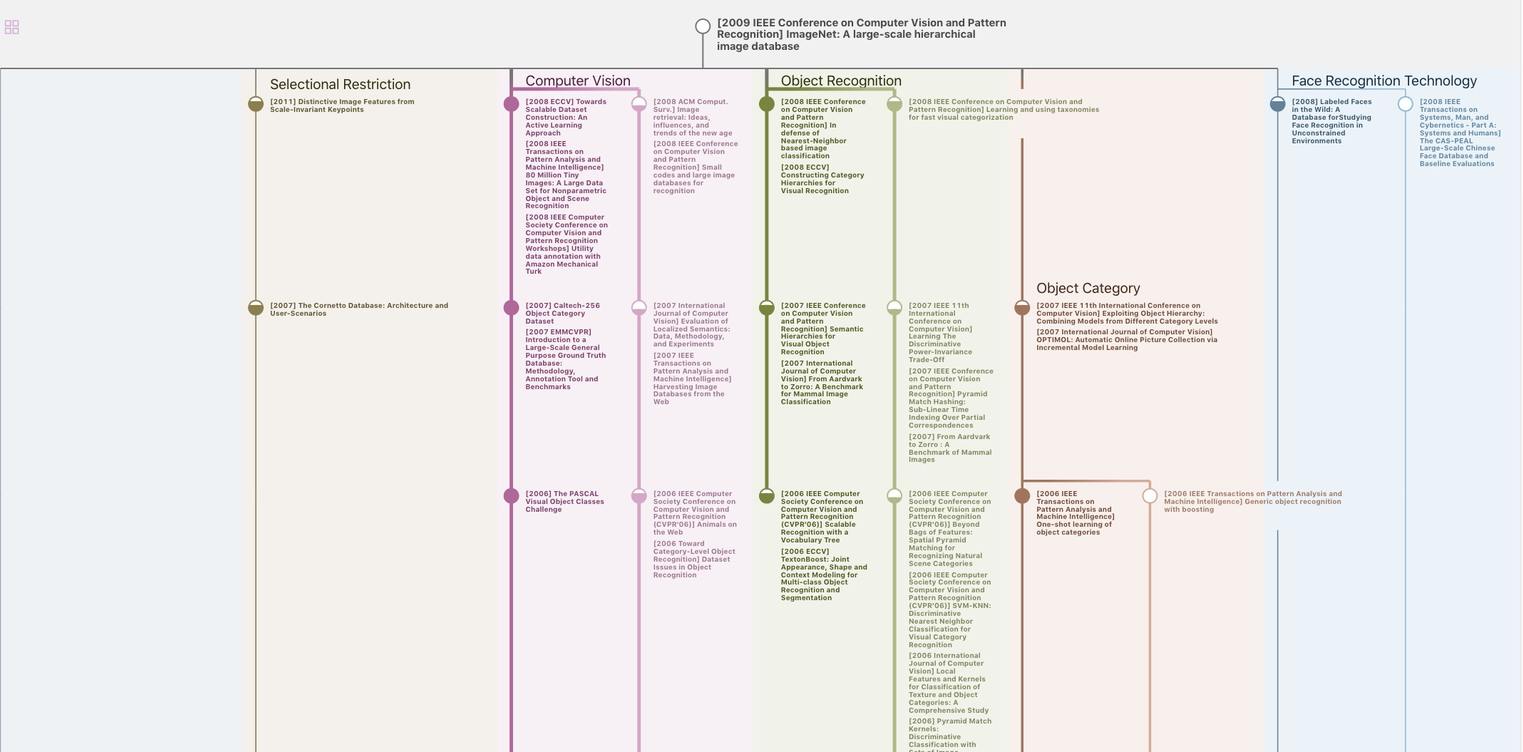
生成溯源树,研究论文发展脉络
Chat Paper
正在生成论文摘要