Path Planning for Unmanned Surface Vehicles with Strong Generalization Ability Based on Improved Proximal Policy Optimization
Sensors(2023)
摘要
To solve the problems of path planning and dynamic obstacle avoidance for an unmanned surface vehicle (USV) in a locally observable non-dynamic ocean environment, a visual perception and decision-making method based on deep reinforcement learning is proposed. This method replaces the full connection layer in the Proximal Policy Optimization (PPO) neural network structure with a convolutional neural network (CNN). In this way, the degree of memorization and forgetting of sample information is controlled. Moreover, this method accumulates reward models faster by preferentially learning samples with high reward values. From the USV-centered radar perception input of the local environment, the output of the action is realized through an end-to-end learning model, and the environment perception and decision are formed as a closed loop. Thus, the proposed algorithm has good adaptability in different marine environments. The simulation results show that, compared with the PPO algorithm, Soft Actor-Critic (SAC) algorithm, and Deep Q Network (DQN) algorithm, the proposed algorithm can accelerate the model convergence speed and improve the path planning performances in partly or fully unknown ocean fields.
更多查看译文
关键词
USV,path planning,deep reinforcement learning,deep neural network,generalization,perception
AI 理解论文
溯源树
样例
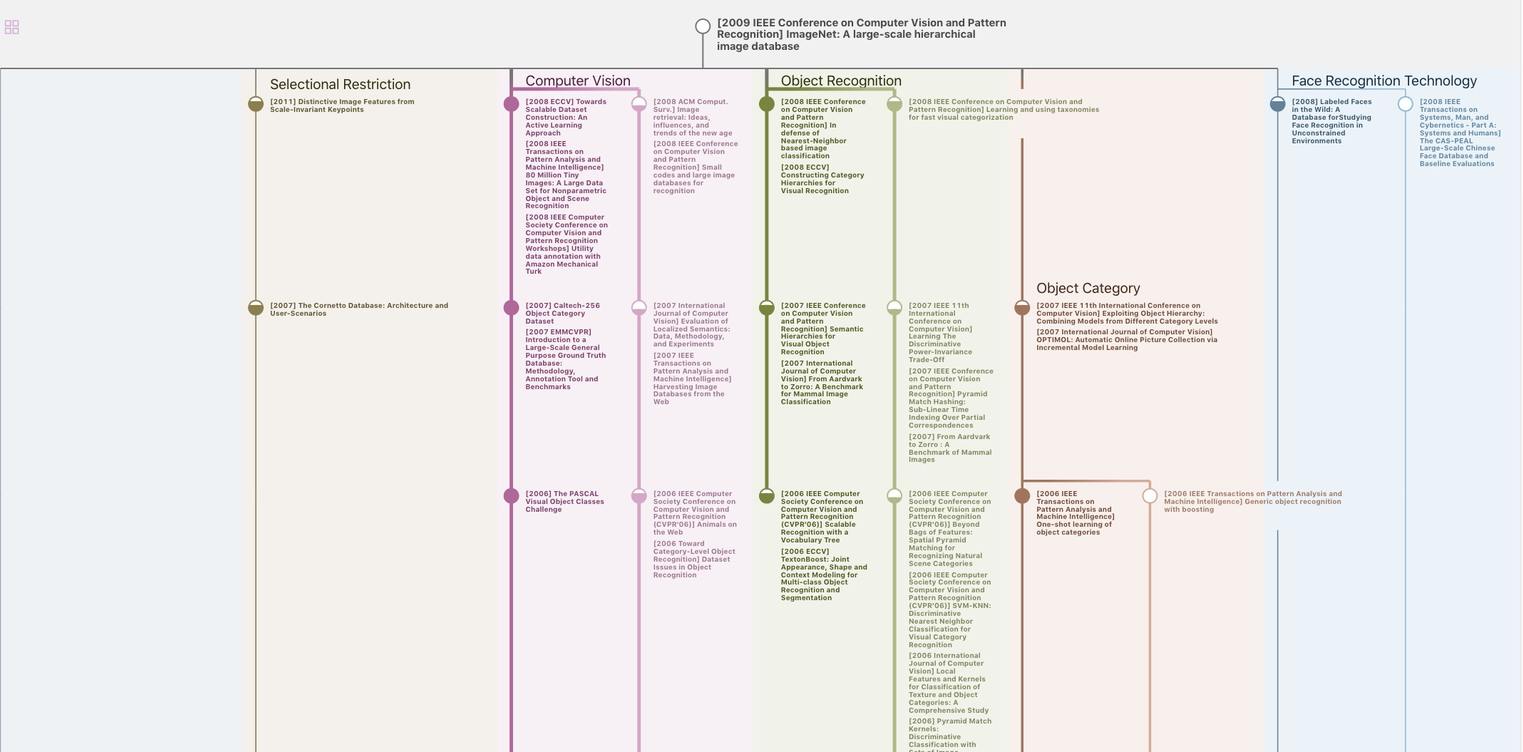
生成溯源树,研究论文发展脉络
Chat Paper
正在生成论文摘要