Discriminative Spatiotemporal Alignment for Self-Supervised Video Correspondence Learning
2023 IEEE INTERNATIONAL CONFERENCE ON MULTIMEDIA AND EXPO, ICME(2023)
摘要
This paper focuses on self-supervised video correspondence learning, which learns effective representations from raw videos without manual annotations and exploits the learned representations for video visual tracking tasks. Previous methods extract temporal correspondence between two frames in fixed geometric structures, which easily leads to mismatches of pixels and overlooks the intra-frame semantic correspondence. To address these issues, we propose a Discriminative Spatio-temporal Alignment (DSA) framework to improve the tracking accuracy in the inference stage. DSA first discriminates representations of different instances for each reference frame through an Instance-Guided Spatial Alignment (IGSA) module. Then, it employs a Focused Temporal Alignment (FTA) module, which samples discriminative pixels from reference frames and propagates the labels of the sampled reference pixels to a target pixel. Experimental results show that DSA possesses flexibility and generalizability and has boosted previous approaches on three tracking tasks, including video object segmentation, human part segmentation, and pose keypoint tracking.
更多查看译文
关键词
self-supervised learning, video correspondence, spatiotemporal alignment
AI 理解论文
溯源树
样例
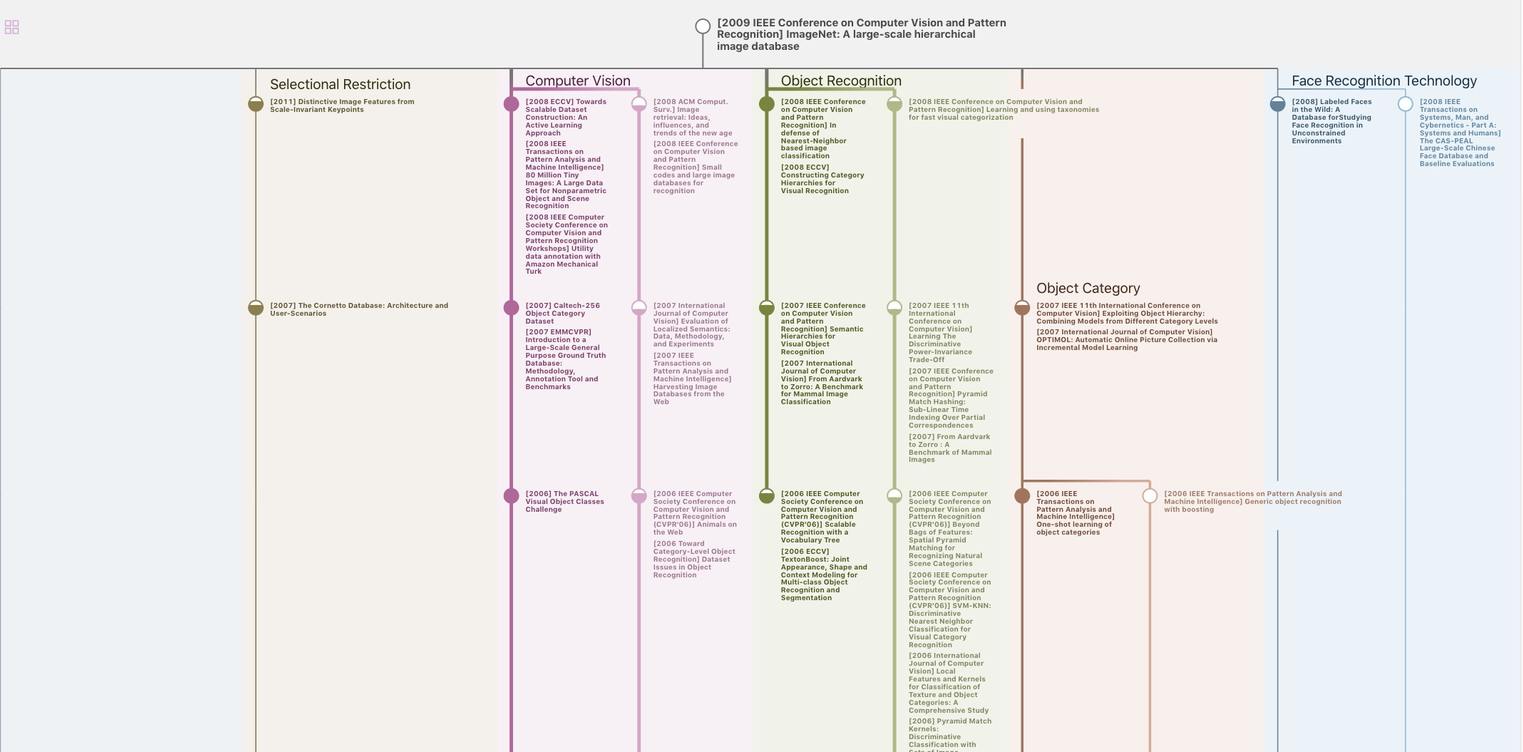
生成溯源树,研究论文发展脉络
Chat Paper
正在生成论文摘要