Feature Mixing and Disentangling for Occluded Person Re-Identification
2023 IEEE INTERNATIONAL CONFERENCE ON MULTIMEDIA AND EXPO, ICME(2023)
摘要
Occluded person re-identification (Re-ID) has recently attracted lots of attention for its applicability in practical scenarios. However, previous pose-based methods always neglect the non-target pedestrian (NTP) problem. In contrast, we propose a feature mixing and disentangling method to train a robust network for occluded person Re-ID without extra data. Based on ViT, we design our network as follows: 1) A multi-target patch mixing (MPM) module is proposed to generate complex multi-target images with refined labels in the training stage. 2) We propose an identity-based patch realignment (IPR) module in the decoder layer to disentangle local features from the multi-target sample. In contrast to pose-guided methods, our approach overcomes the difficulties of NTP. More importantly, our approach does not bring additional computational costs in the training and testing phases. Experimental results show that our method effectively on occluded person Re-ID. For example, our method performs 3.3%/3.2% better than the baseline on Occluded-Duke in terms of mAP/rank-1 and outperforms the previous state-of-the-art.
更多查看译文
关键词
occluded person re-identification,feature disentangling,feature mixing
AI 理解论文
溯源树
样例
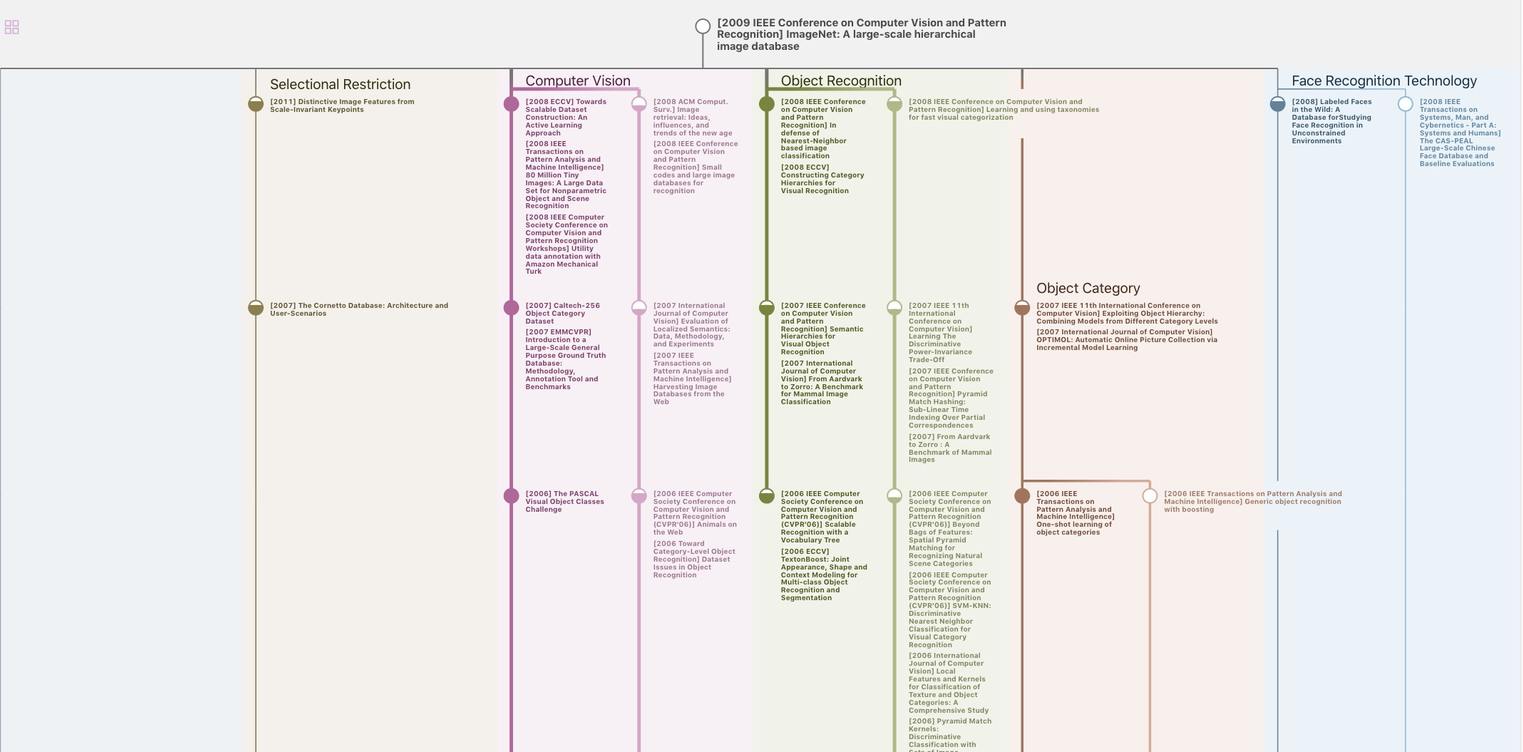
生成溯源树,研究论文发展脉络
Chat Paper
正在生成论文摘要