LACL: Lesion-Aware Contrastive Learning Framework for Medical Image Classification
2023 IEEE INTERNATIONAL CONFERENCE ON MULTIMEDIA AND EXPO, ICME(2023)
摘要
Recently, contrastive learning has received significant attention in various classification tasks of natural images. However, current contrastive learning frameworks display unsatisfactory performance on medical images due to the inability of obtaining fine-grained visual features. In this paper, we propose a Lesion-Aware Contrastive Learning (LACL) framework to learn more discriminative and comprehensive representations and reinforce the attention of diagnosis regions on medical images. LACL framework includes two phases of training procedures: the comprehensive feature-extracting phase and the contrastive learning enhancement phase. In the first phase, LACL fully captures meaningful deep features related to the training targets to form comprehensive visual representations, by training a novel lesion-aware module we proposed. In the second phase, we introduce the previous representation information into contrastive learning to guide the LACL framework in learning disease- related features. This approach provides more effective guidance than the traditional contrastive learning method of directly comparing features. Extensive experiments on several benchmark datasets demonstrate that our LACL framework significantly improves the performance of medical image classification and highlights the lesion areas for disease diagnosis.
更多查看译文
关键词
contrastive learning, medical image, transfer learning, feature extraction
AI 理解论文
溯源树
样例
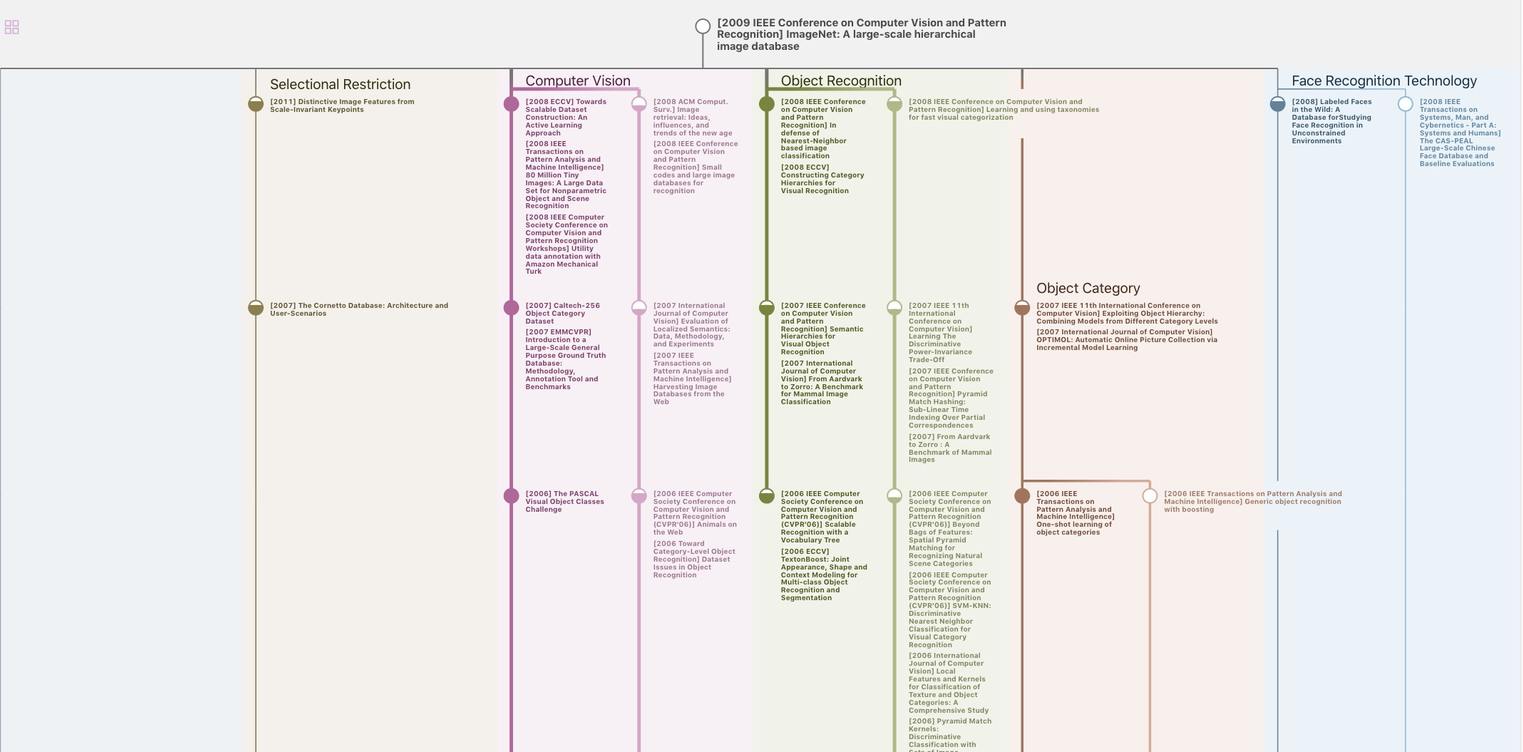
生成溯源树,研究论文发展脉络
Chat Paper
正在生成论文摘要