Image Deraining Transformer with Sparsity and Frequency Guidance
2023 IEEE INTERNATIONAL CONFERENCE ON MULTIMEDIA AND EXPO, ICME(2023)
摘要
In recent years, Transformer has witnessed significant progress in the single image deraining field. However, most existing methods do not consider the latent sparse representation and distinguished frequency information. To this end, this paper proposes an effective Image Deraining Transformer with Sparsity and Frequency Guidance, called SFG-IDT. To achieve such guidance, the proposed method consists two key designs: sparsity-compensated multi-head attention (SCMA) and frequency-enhanced multi-scale operator (FEMO). Specifically, the SCMA enhances the concentration of attention while explicitly retaining non-local connectivity with Locality Sensitive Hashing (LSH), to facilitate rain removal better and help image restoration. Simultaneously, the FEMO integrates the frequency information into the multi-scale convolution operators with Fast Fourier Transform (FFT) to obtain a more accurate representation for achieving high-quality derained results. Extensive experimental results show that our developed SFG-IDT outperforms the state-of-the-art approach (Restormer) by 0.27 dB on average, but saves 50.3% parameters and 46.7% computational cost.
更多查看译文
关键词
Image Restoration,Locality Sensitive Hashing,Fast Fourier Transform,Single Image Deraining,Transformer
AI 理解论文
溯源树
样例
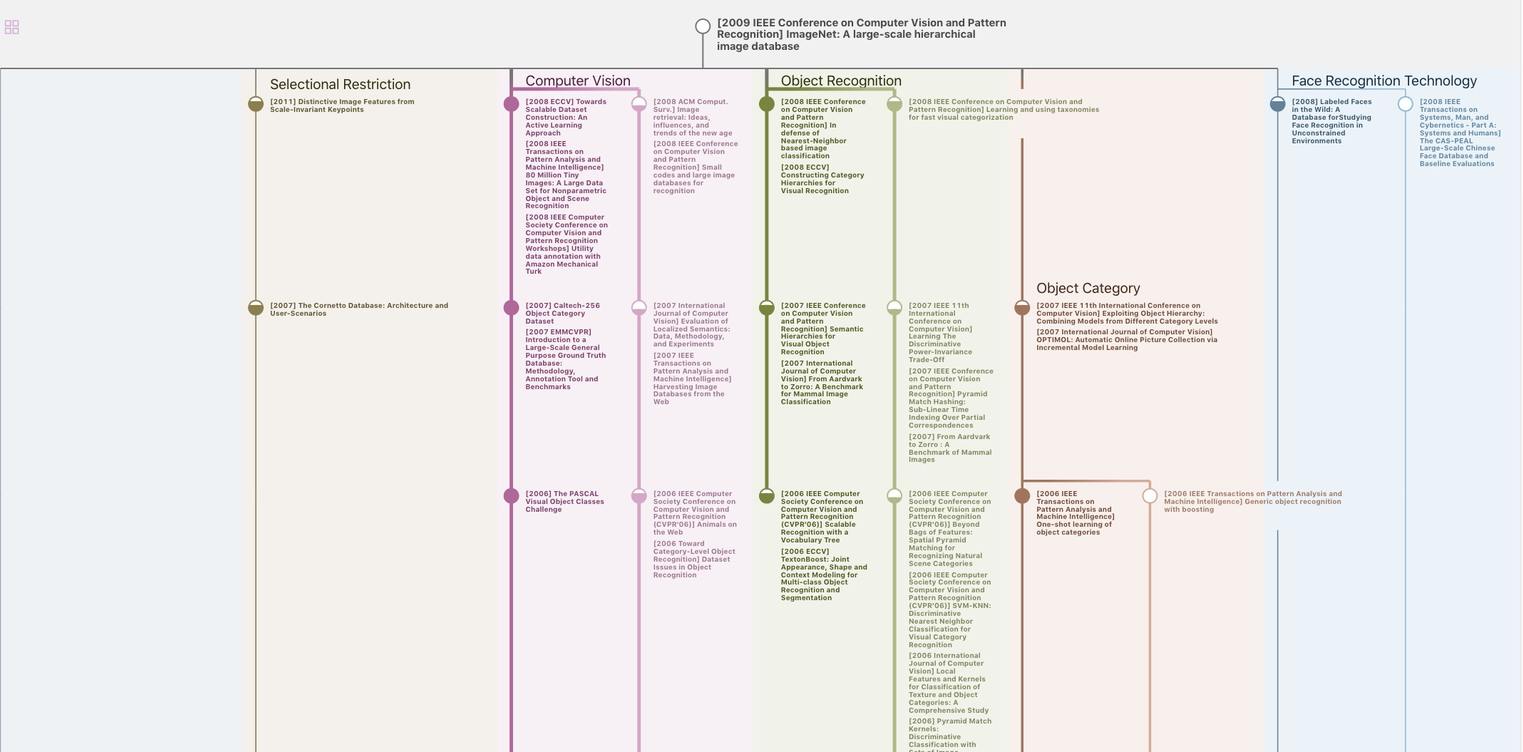
生成溯源树,研究论文发展脉络
Chat Paper
正在生成论文摘要