DR-Net: A Multi-View Face Synthesis Network Driven by Dual Representation
2023 IEEE INTERNATIONAL CONFERENCE ON MULTIMEDIA AND EXPO, ICME(2023)
摘要
With the rapid growth of virtual human techniques and the popularity of 3D face modeling, multi-view face synthesis has received considerable attention in the computer vision community. Though learning-based methods have achieved photorealistic effect, the lack of geometry guidance remains a great hurdle to their results in high fidelity of identity. To relieve it, we propose a novel network named Dual-Representation-Net (DR-Net) driven by both learning and geometry information. In addition, we utilize face parsing to lead the learning of regional attention, in this way, the two synthesizing representations can collaborate and compete dynamically under different face angles, which improves the stability of face synthesis by assimilating the benefits of both kinds of information. What is more, we want to emphasize that DR-Net can serve as a general framework for multi-view face synthesis by jointly exploiting learning and geometry information. That is, our geometry module and learning module are actually substitutable, which can be replaced by any other SOTA methods. This makes our method both flexible and scalable. Extensive experiments verify that our approach is superior to other counterparts in both synthesis quality and identity preservation. Moreover, with the geometric information, we can decouple the synthesis of face and background to obtain more robust results on in-the-wild data.
更多查看译文
关键词
Multi-view face synthesis,3D geometry modeling,deep learning,dual-representation net,attention network
AI 理解论文
溯源树
样例
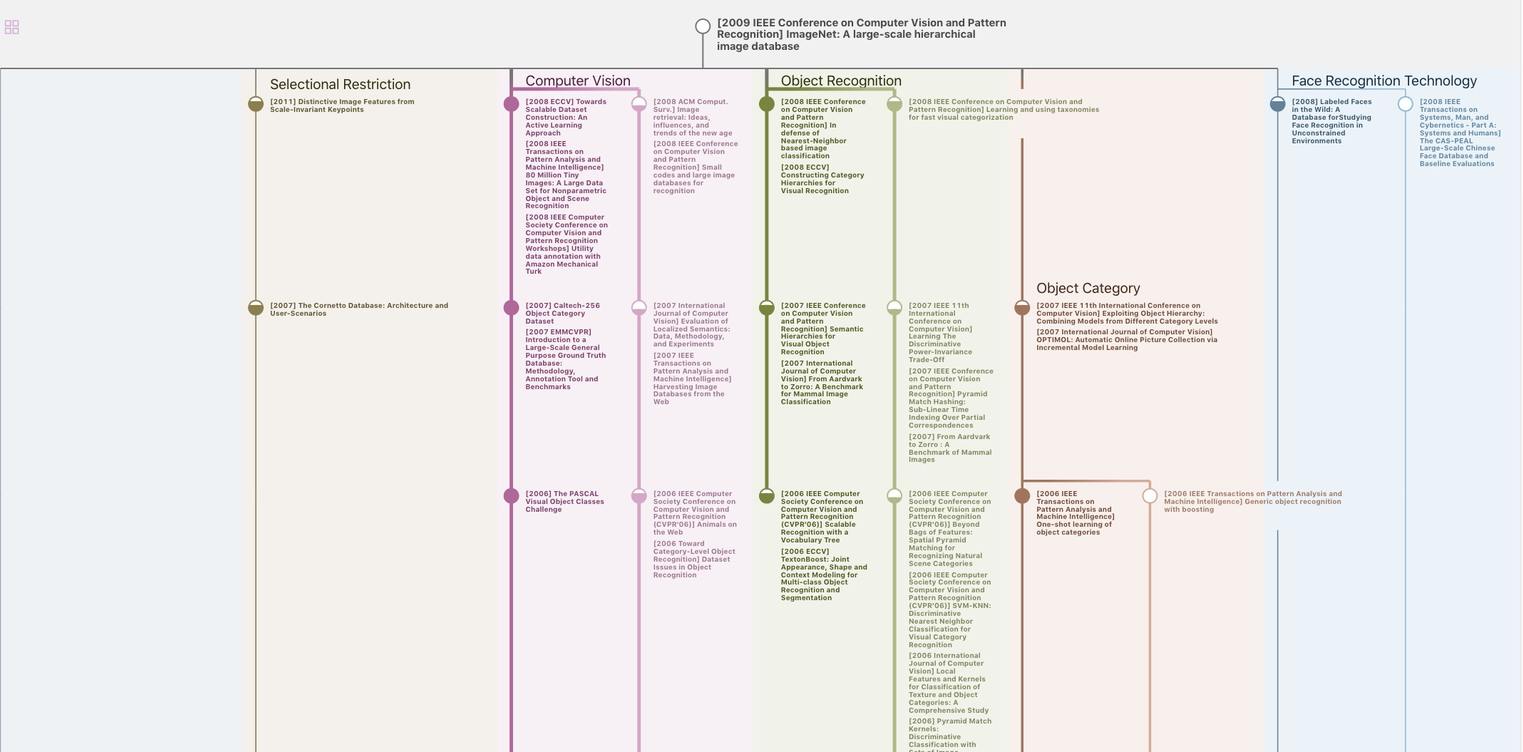
生成溯源树,研究论文发展脉络
Chat Paper
正在生成论文摘要