Learning High Frequency Surface Functions In Shells
2023 IEEE INTERNATIONAL CONFERENCE ON MULTIMEDIA AND EXPO, ICME(2023)
摘要
Recently, coordinate-based MLPs have been shown to be powerful representations for 3D surfaces, where learning high-frequency details is facilitated by modulating surface functions with periodic functions [1], [2]. While shortening the periodicity helps in learning high frequencies, it leads to increasing ambiguity, i.e., more points along the axis directions become similar in the embedded space, so that many points on the surface and outside the surface have similar predictions. In addition, short periodicity increases local geometric variations, leading to unexpected noisy artifacts in untrained regions. Unlike existing methods that learn surface functions in a regular cube, we find surfaces within shells, a coarse form of the target surfaces constructed by a binary classifier. The advantage of build surfaces in shells is that MLPs focus on regions of interest, which inherently reduces ambiguity and also promotes training efficiency and test accuracy. We demonstrate the effectiveness of shells and show significant improvements over baseline methods in 3D surface reconstruction from raw point clouds.
更多查看译文
关键词
surface reconstruction, coordinate-based MLPs, implicit neural representation, Fourier feature embedding, 3D point clouds
AI 理解论文
溯源树
样例
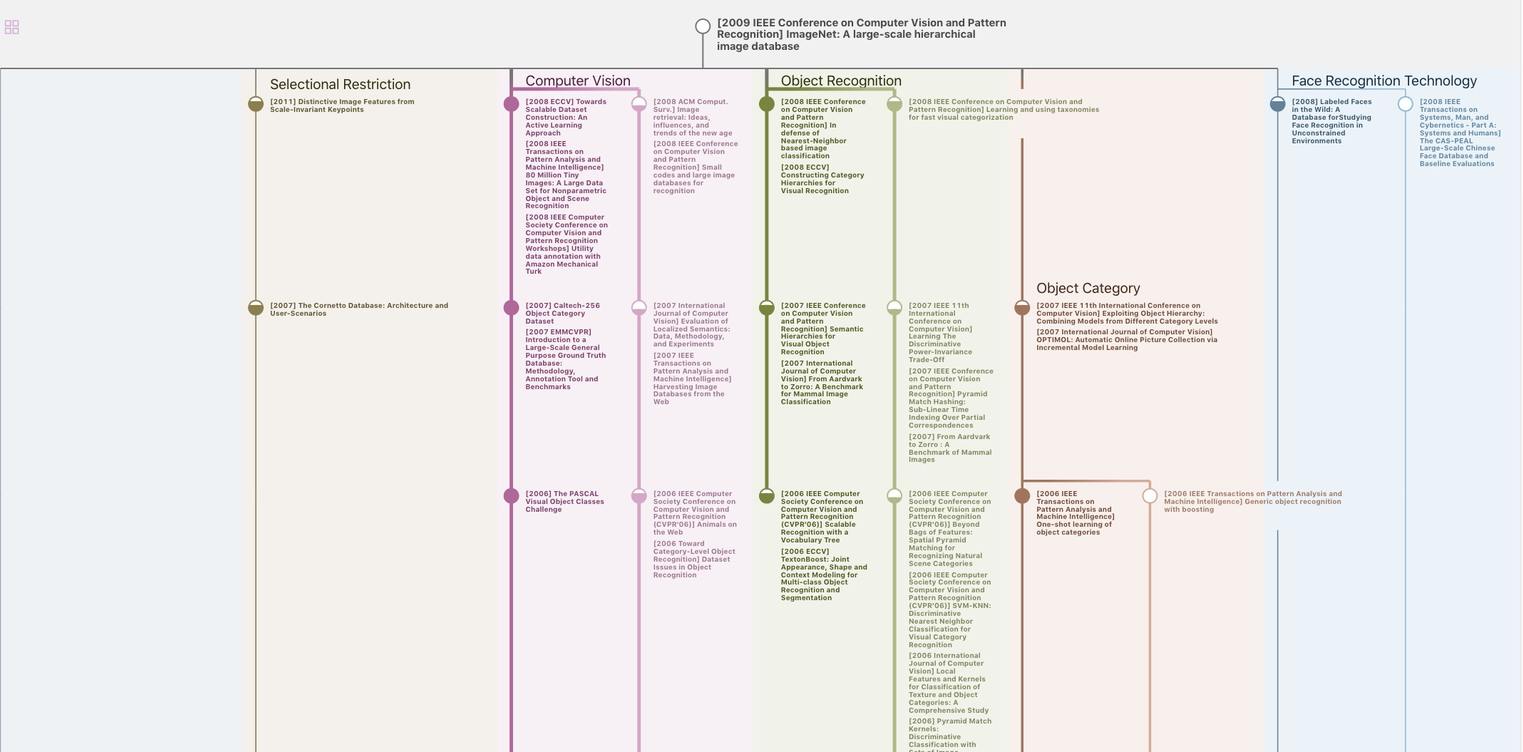
生成溯源树,研究论文发展脉络
Chat Paper
正在生成论文摘要