Intensifying the consistency of pseudo label refinement for unsupervised domain adaptation person re-identification
2023 IEEE INTERNATIONAL CONFERENCE ON MULTIMEDIA AND EXPO, ICME(2023)
摘要
Clustering-based unsupervised domain adaptation (UDA) for person re-identification aims to learn in the unlabeled target domain. However, the noise problem of clustering-based generated pseudo labels remains under-explored, and these wrong labels can mislead the feature learning process. In this paper, we propose a consistent and intensive pseudo label refinement method in which pseudo labels generated in two different feature spaces, local and global, refine each other to improve the pseudo label quality of the final clusters. Then we utilize a quantitative criterion to measure label inaccuracy and fine-tune the target domain to reduce the noise by an inaccuracy-guided pseudo label optimization scheme. On a strong benchmark, we demonstrate the superiority of the method with extensive experiments. Specifically, our method outperforms the baseline by 7.2% mAP on the Duke2Market task and 0.5% mAP on the Market2MSMT task over the state-of-the-art.
更多查看译文
关键词
person re-identification, domain adaptation, mutual refinement, label accuracy optimization
AI 理解论文
溯源树
样例
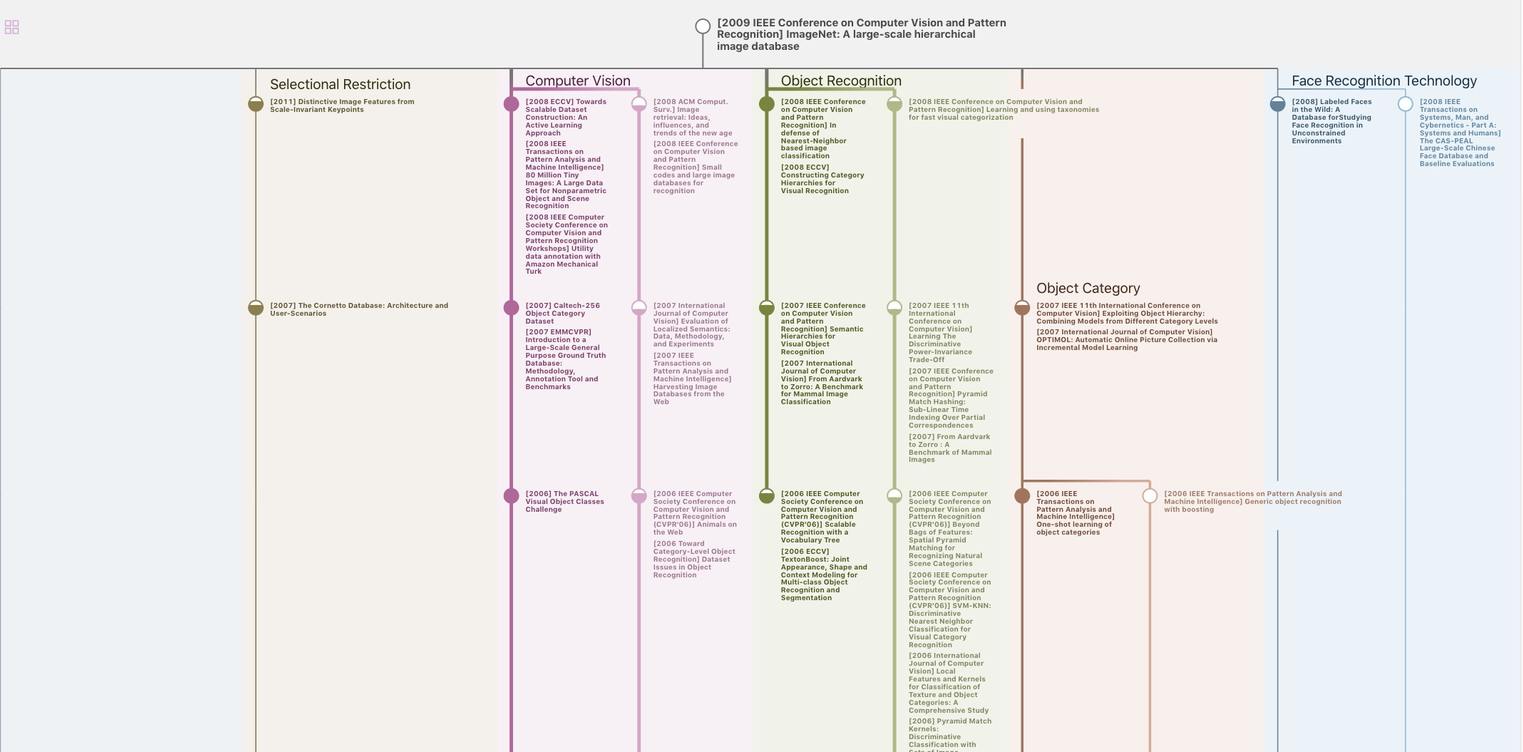
生成溯源树,研究论文发展脉络
Chat Paper
正在生成论文摘要