SWATM: Contribution-Aware Adaptive Federated Learning Framework Based on Augmented Shapley Values
2023 IEEE INTERNATIONAL CONFERENCE ON MULTIMEDIA AND EXPO, ICME(2023)
摘要
The vanilla federated learning (FedAvg) takes a weighted aggregation of uploaded models based on the amount of data from each participant. By addressing varying data quality and heterogeneous data distributions across participants, the existing work calculates the contribution of participants based on Shapley Value (SV) and attempt to guide model aggregation under SV. Our empirical study found that vanilla SV exhibits low sensitivity and instability issues. To address these issues, we propose a Shapley Value Weighted FL aggregation method based on Adaptive Temperature augmentation and Momentum update (SWATM). The adaptive temperature moderately augments the sensitivity of SV according to aggregation performance, while the momentum update slows down the fluctuation and improves stability. Experiments on CIFAR-10 show that, our approach can significantly improve stability compared to the state-of-the-art, and experiments on household safety inspection show that our method accelerate the convergence of the global model. Finally, we deploy SWATM for predictive maintenance of equipment with a combined F1-score improvement of 2.57%.
更多查看译文
关键词
Federated learning, contribution evaluation, Shapley value, adaptive temperature
AI 理解论文
溯源树
样例
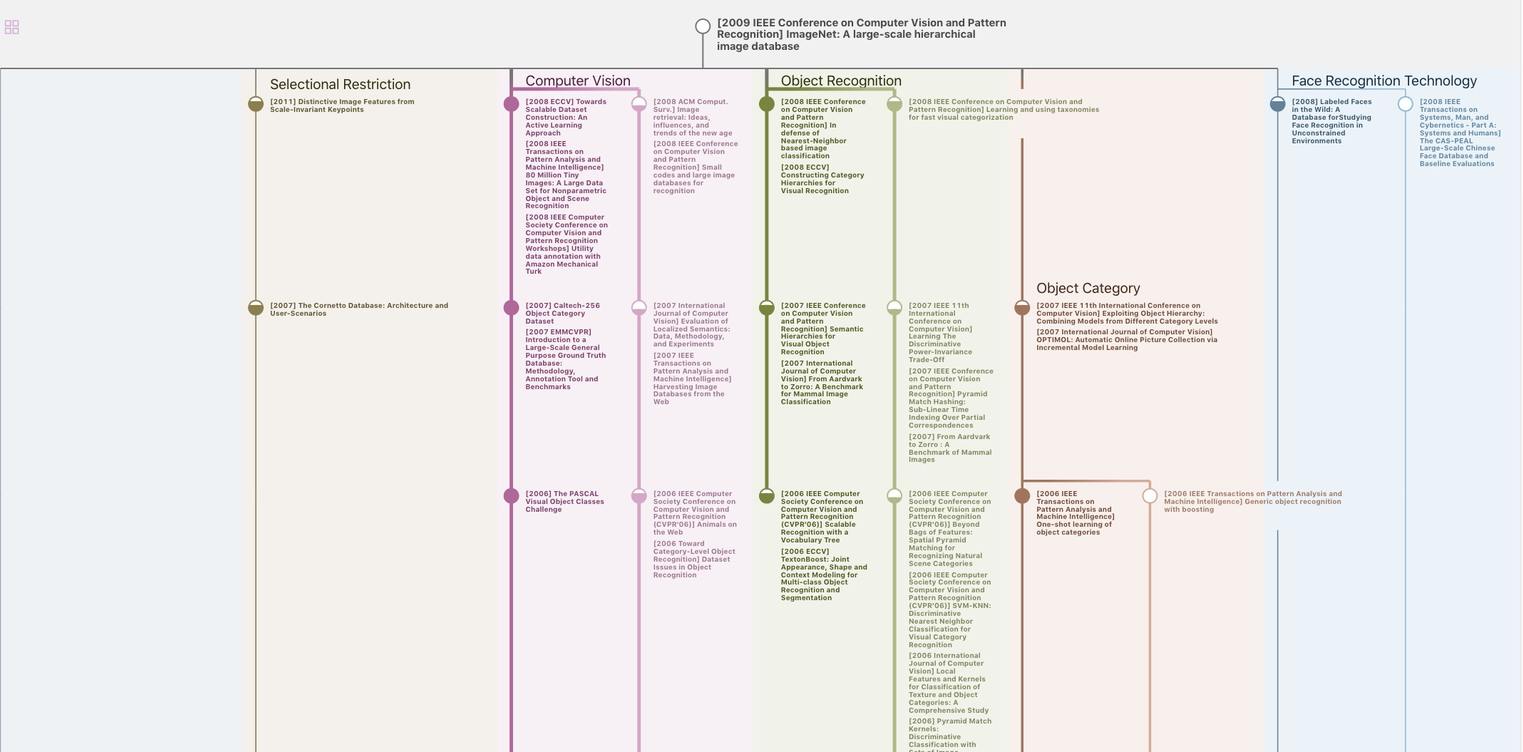
生成溯源树,研究论文发展脉络
Chat Paper
正在生成论文摘要