ShiftFormer: Spatial-Temporal Shift Operation in Video Transformer
2023 IEEE INTERNATIONAL CONFERENCE ON MULTIMEDIA AND EXPO, ICME(2023)
摘要
Transformers have achieved great success in various tasks, especially that introducing pure Transformers into video understanding shows powerful performance. However, video Transformer suffers from the problem of memory explosion: it is difficult to be deployed on hardware due to the intensive computation. To address this issue, we propose ST-shift (spatial-temporal) operation with zero computation and zero parameter. We are only shifting a small portion of the channels along the temporal and spatial dimensions. Based on this operation, we build an attention-free ShiftFormer, where ST-shift blocks substitute the attention layers in video Transformer. ShiftFormer is accurate and efficient: it can reduce 56.34% of memory usage and achieve 3.41x faster training. When both using random initialization, our model performs even better than Video Swin Transformer for video recognition on Something-Something v2.
更多查看译文
关键词
Video Classification,Transformer,Shift Operation
AI 理解论文
溯源树
样例
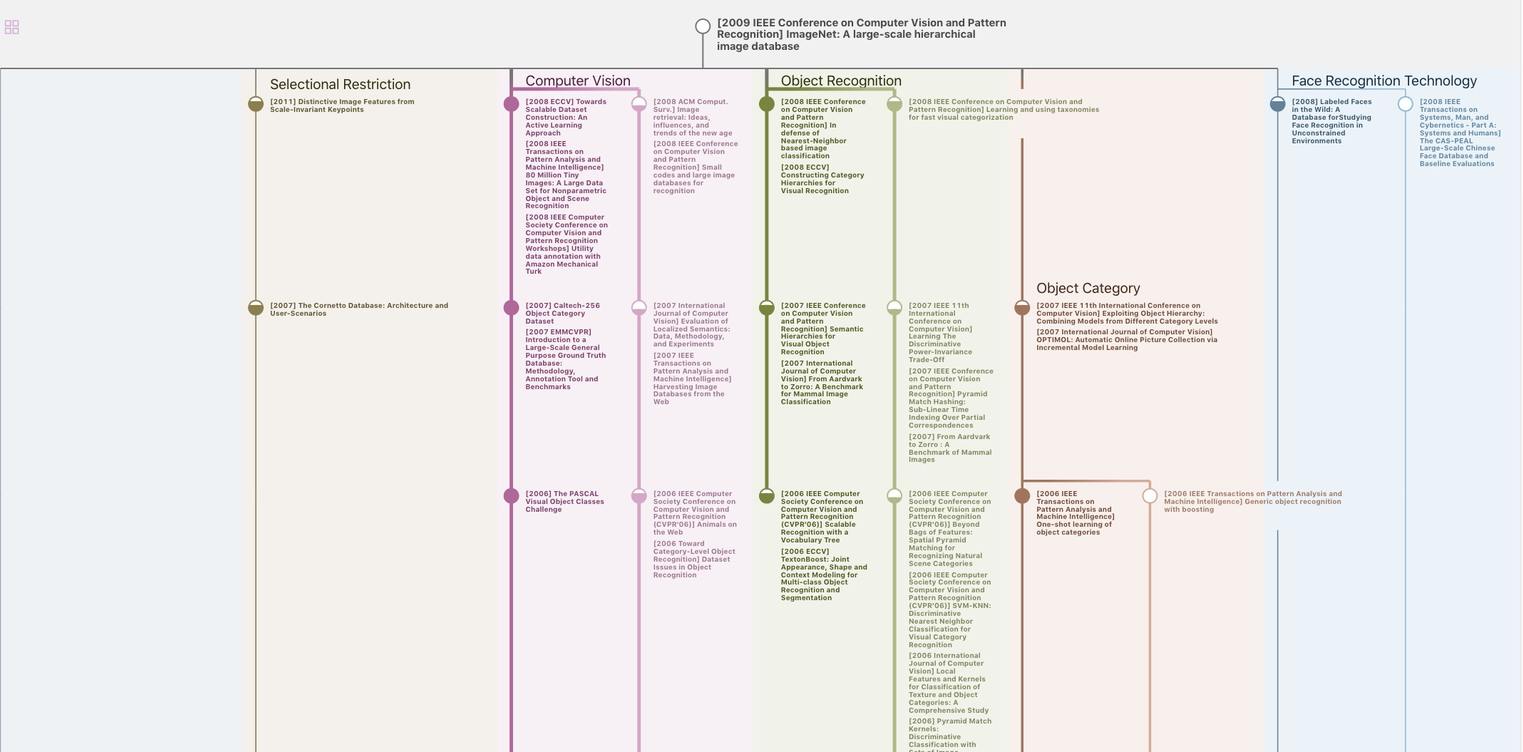
生成溯源树,研究论文发展脉络
Chat Paper
正在生成论文摘要