Deep sample clustering domain adaptation for breast histopathology image classification
BIOMEDICAL SIGNAL PROCESSING AND CONTROL(2024)
摘要
Deep learning has been widely applied to the diagnosis of breast cancer. Most deep learning models require a large number of labeled samples for training, but a large number of datasets with detailed labels is impractical. Therefore, exploring the methods to accurately classify unlabeled data remains a crucial area of research. In this paper, a deep sample clustering unsupervised domain adaptation method based on deep fusion feature is pro-posed for breast histopathology image classification. Deep multi-level fusion feature has a strong ability to capture image information, allowing for accurate characterization of the structural features of cells, thus improving the clustering performance. Next, based on the criterion of adaptive clustering of samples, the sim-ilarity between different clustering centers in the source and target domains is adjusted jointly. Finally, the classification results were achieved by the nearest neighbor relationship between the clustering centers and samples in the target domain. The proposed method was tested on three different breast cancer datasets. The prediction results of all patches were aggregated to obtain the classification accuracy of the histopathological image. The experimental results demonstrate that the proposed method achieves high classification accuracy and has the potential for application in clinical settings.
更多查看译文
关键词
Unsupervised domain adaptation,Breast histopathology image,Samples clustering,Fusion feature
AI 理解论文
溯源树
样例
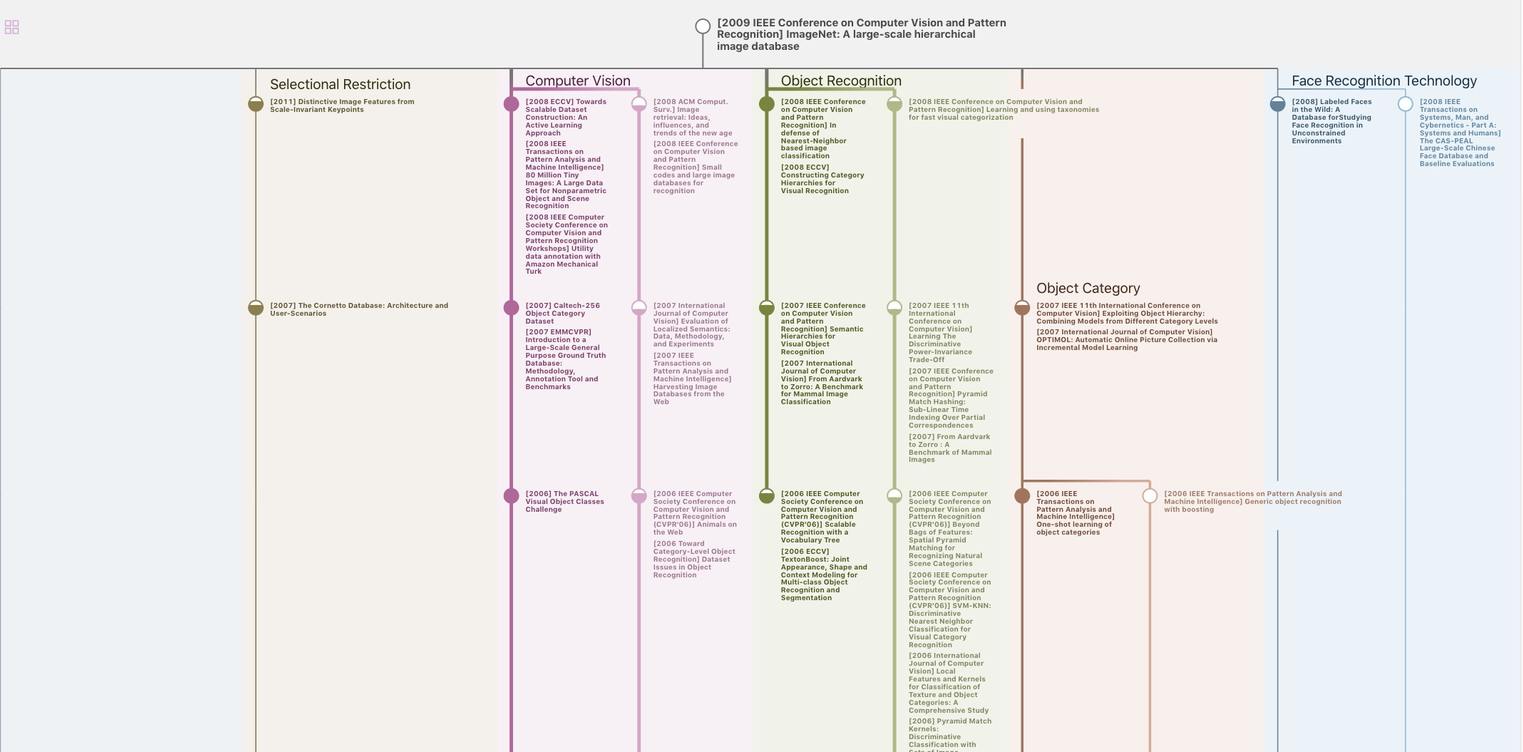
生成溯源树,研究论文发展脉络
Chat Paper
正在生成论文摘要