A Visually Interpretable Convolutional-Transformer Model for Assessing Depression from Facial Images
2023 IEEE INTERNATIONAL CONFERENCE ON MULTIMEDIA AND EXPO, ICME(2023)
摘要
The accuracy and availability are the most critical and challenging problems for major depressive disorder (MDD) diagnosis. Limited receptive field and inaccurate visual interpretation always weaken the clinical application of deep learning-based depression recognition model. Thus, we propose a visually interpretable depression monitoring model termed Transformer and Convolutional with slot-attention (TC-slot) to assess depression from facial images. Specifically, this approach stands upon the intersection of convolution and transformer, combines self-attention mechanism and deep convolution, and uses a well-designed stem structure to explore the global and local relationships. Moreover, in TC-slot, a classifier built on slot-attention mechanism directly involved in the decision-making process further localizes salient regions of facial depression patterns and provides precise and meaningful explanations. The results indicate that the proposed approach effectively improves the classification and recognition performance compared with other state-of-the-art approaches, with guaranteed favorable visual interpretability, providing clinical insights into the assessment of the assessing depression.
更多查看译文
关键词
Depression, Convolutional neural networks, Transformer, Visual interpretability
AI 理解论文
溯源树
样例
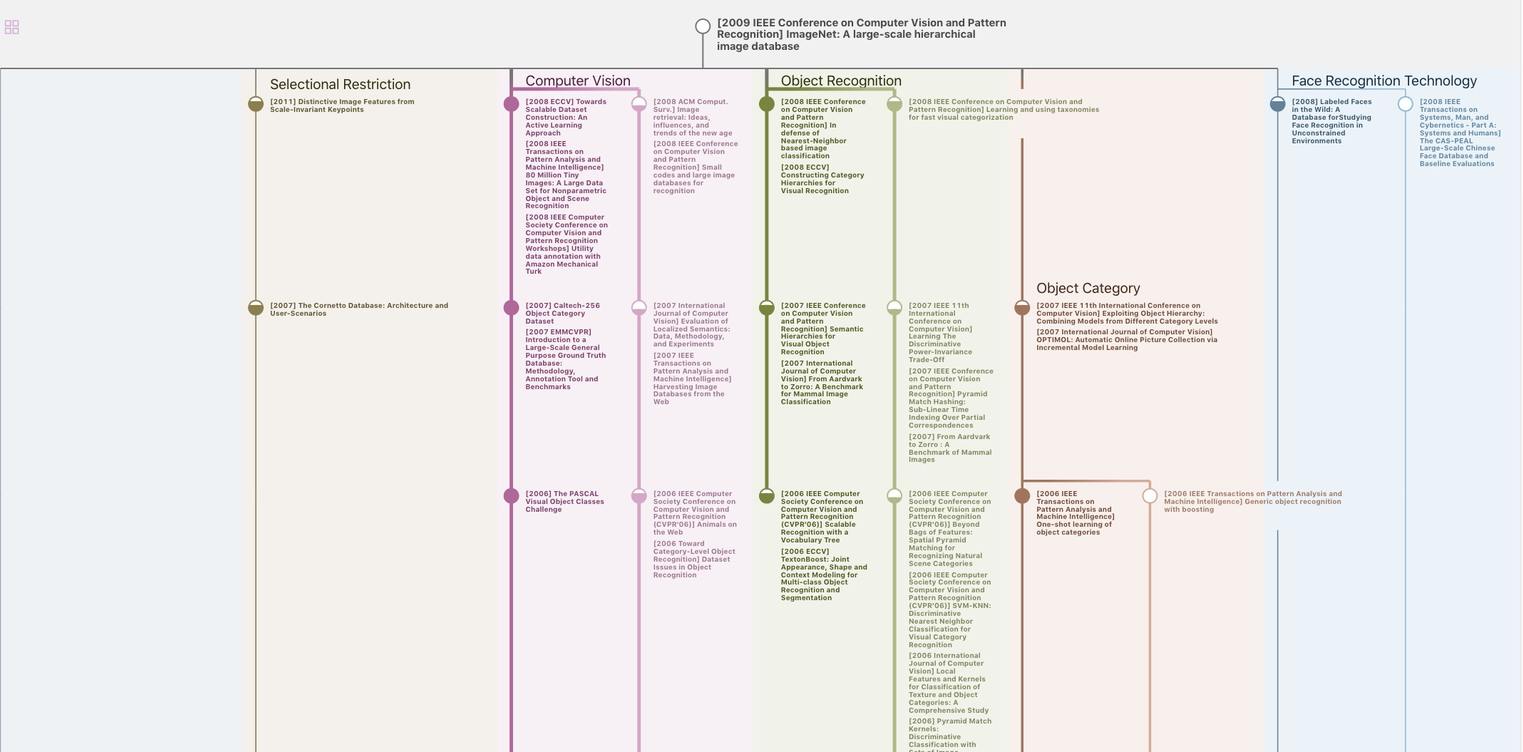
生成溯源树,研究论文发展脉络
Chat Paper
正在生成论文摘要