Time-series forecasting of road distress parameters using dynamic Bayesian belief networks
CONSTRUCTION INNOVATION-ENGLAND(2024)
摘要
PurposeTo proactively draw efficient maintenance plans, road agencies should be able to forecast main road distress parameters, such as cracking, rutting, deflection and International Roughness Index (IRI). Nonetheless, the behavior of those parameters throughout pavement life cycles is associated with high uncertainty, resulting from various interrelated factors that fluctuate over time. This study aims to propose the use of dynamic Bayesian belief networks for the development of time-series prediction models to probabilistically forecast road distress parameters.Design/methodology/approachWhile Bayesian belief network (BBN) has the merit of capturing uncertainty associated with variables in a domain, dynamic BBNs, in particular, are deemed ideal for forecasting road distress over time due to its Markovian and invariant transition probability properties. Four dynamic BBN models are developed to represent rutting, deflection, cracking and IRI, using pavement data collected from 32 major road sections in the United Arab Emirates between 2013 and 2019. Those models are based on several factors affecting pavement deterioration, which are classified into three categories traffic factors, environmental factors and road-specific factors.FindingsThe four developed performance prediction models achieved an overall precision and reliability rate of over 80%.Originality/valueThe proposed approach provides flexibility to illustrate road conditions under various scenarios, which is beneficial for pavement maintainers in obtaining a realistic representation of expected future road conditions, where maintenance efforts could be prioritized and optimized.
更多查看译文
关键词
Road distress parameters,Dynamic Bayesian belief networks,Time-series data,Performance prediction modeling
AI 理解论文
溯源树
样例
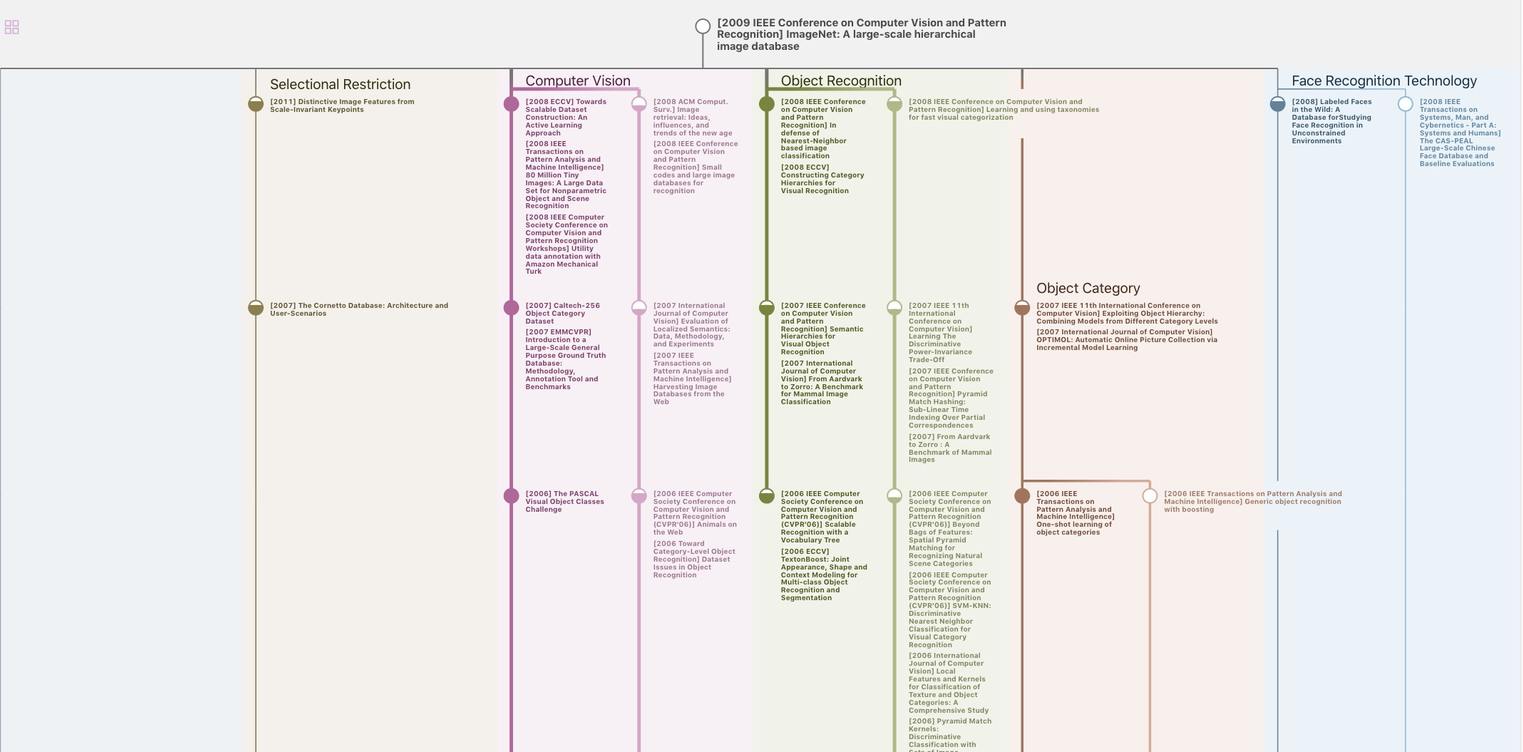
生成溯源树,研究论文发展脉络
Chat Paper
正在生成论文摘要