Indoor temperature prediction with context-aware models in residential buildings
BUILDING AND ENVIRONMENT(2023)
摘要
This paper presents a novel approach for predicting average indoor temperature in residential buildings, utilizing contextual factors of the rise of the building and geographical location. The proposed approach employs advanced deep learning architectures, such as Long Short-Term Memory (LSTM) and Transformers, to create generalized predictive models applicable to a range of residential buildings. The models are trained using historical data from 18 residential buildings over a period of 6 to 10 years, where the buildings are located in different climate zones. Testing is done on nine different data sets representing three different locations and three different types of buildings. The study demonstrates that incorporating the context of building rise significantly improves the models' predictive performance. Specifically, the transformer-based models show improvements in R2 of 4%-27% in a 6 h prediction horizon. The proposed approach explicitly using context information significantly improves the accuracy of predicting, making learnt models a good starting point for optimizing district heating distribution.
更多查看译文
关键词
Residential buildings,Indoor temperature prediction,Context-aware models,Long Short-Term Memory (LSTM),Transformer
AI 理解论文
溯源树
样例
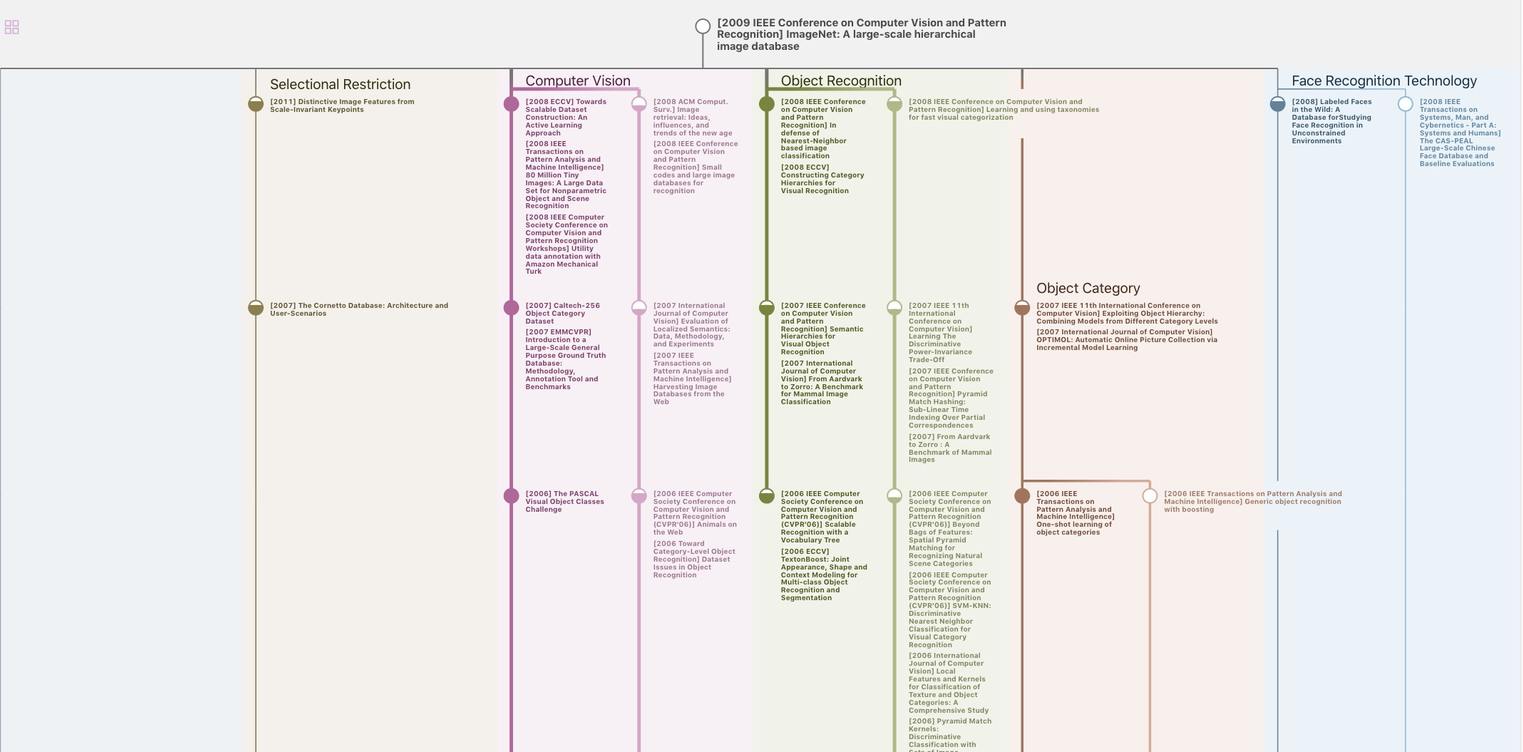
生成溯源树,研究论文发展脉络
Chat Paper
正在生成论文摘要