RLGAT: Retweet prediction in social networks using representation learning and GATs
MULTIMEDIA TOOLS AND APPLICATIONS(2023)
摘要
With the exponential growth of social media platforms, retweet behavior has become a crucial factor in various social network applications like message diffusion, business intelligence, and E-commerce recommendations. The primary objective of this paper is to predict whether a user will retweet a tweet posted by followees. However, the existing prediction methods cannot model the complex interaction between users. Moreover, some complex and implicit features (e.g. content semantic and structural information) are difficult to be represented and fused reasonably and comprehensively. To address the above issues, we propose a novel framework named RLGAT by using R epresentation L earning and G raph A ttention N etworks (GATs) for retweet prediction. RLGAT combines content, structure and social attributes to predict retweet behavior. XLNet-CNN and E-SDNE are employed to generate content and structural representations, respectively. Based on the extracted features of content, structure and social attributes, the AE-GATs model for prediction can further incorporate the correlation of nodes into the generation of node representations. The two real-world datasets are extracted from Sina Microblog and Twitter. The results demonstrate the effectiveness of XLNet-CNN, E-SDNE, AE-GATs, and RLGAT. Notably, RLGAT surpasses state-of-the-art methods, achieving an F1 score of 0.8078 and 0.8017 on Sina and Twitter, respectively. RLGAT is not only effective in predicting user’s retweet behavior, but also beneficial for predicting information diffusion.
更多查看译文
关键词
Retweet prediction,Social network,Graph Attention Networks,Representation learning,XLNet
AI 理解论文
溯源树
样例
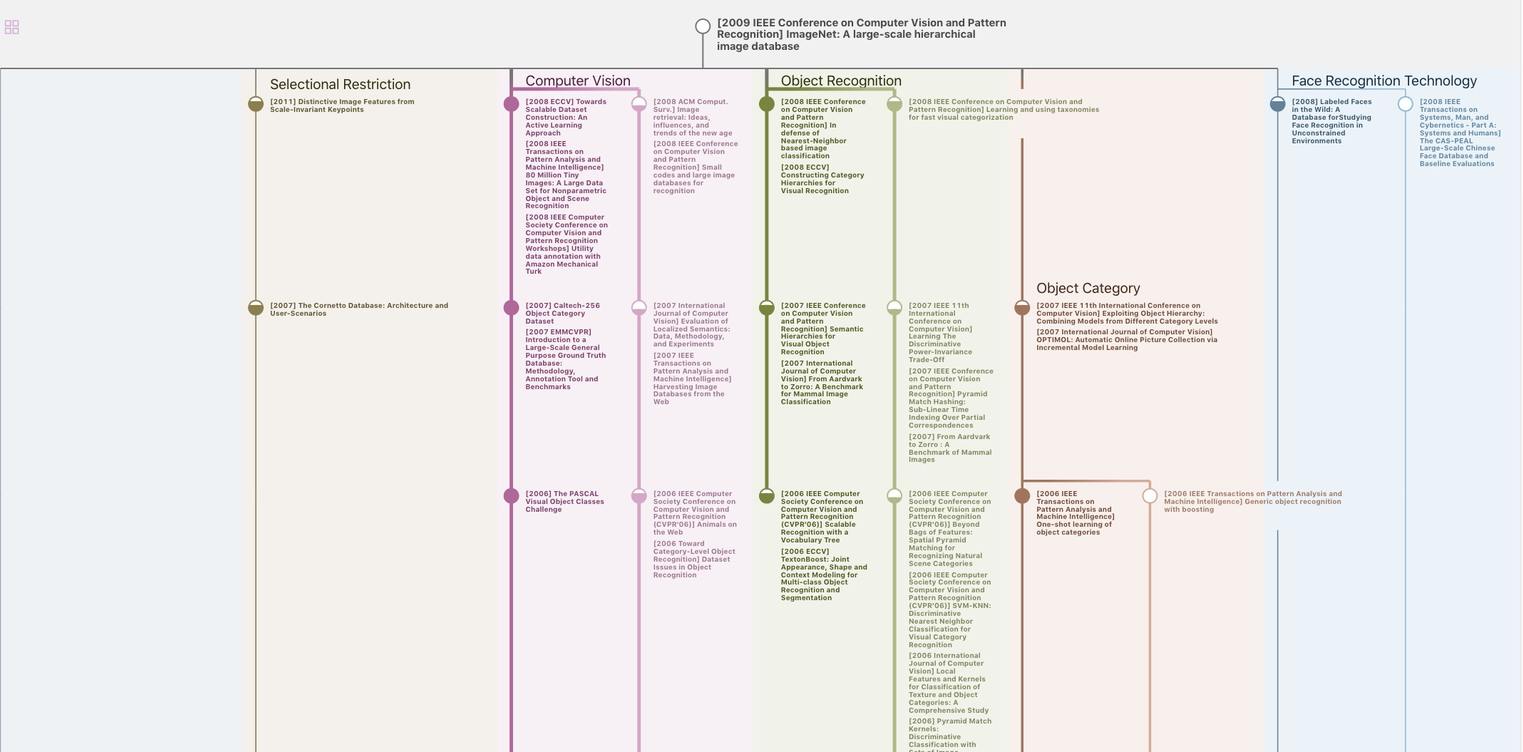
生成溯源树,研究论文发展脉络
Chat Paper
正在生成论文摘要