Assessing the Suitability of Different Machine Learning Approaches for Smart Traffic Mobility
2023 IEEE TRANSPORTATION ELECTRIFICATION CONFERENCE & EXPO, ITEC(2023)
摘要
One of the most critical traffic management issues is congestion in modern and big smart cities. The first task is to accurately forecast traffic patterns to reduce congestion and accidents due to rapid economic development and rising number of vehicles. It is essential for Intelligent Transportation Systems to accurately anticipate future traffic circumstances (such as traffic flow, speed, and traffic time) so that administrators may take proper preventative actions against congestion and travelers can make better-informed judgments. Better trip planning, more efficient traffic operations, lower carbon emissions, and less congestion are all possible outcomes of this forecast. This paper explores different deep-learning time-series forecasting methods such as LSTM, BiLSTM, Prophet, and Transformer models for making short-term predictions regarding traffic flows to ensure smart mobility. The next step is to analyze traffic patterns to provide convenient transportation mobility. Then, we evaluated several performance matrices and computational loads of the proposed methods in this paper.
更多查看译文
关键词
Traffic flow prediction, Traffic congestion, Deep learning, Smart mobility, LSTM, Prophet, Transformer
AI 理解论文
溯源树
样例
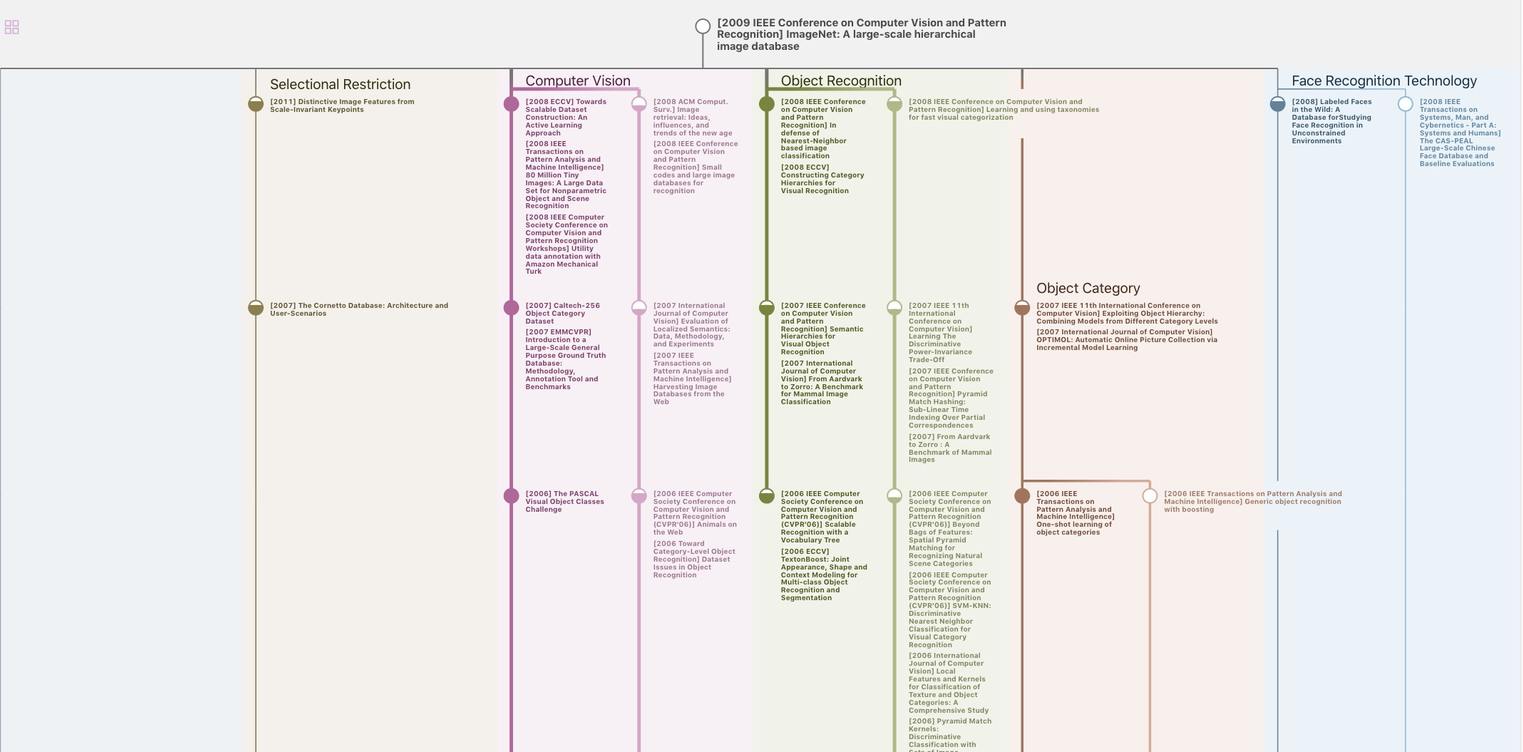
生成溯源树,研究论文发展脉络
Chat Paper
正在生成论文摘要