Lithium-ion Battery State-of-Health Estimation via Histogram Data, Principal Component Analysis, and Machine Learning
2023 IEEE TRANSPORTATION ELECTRIFICATION CONFERENCE & EXPO, ITEC(2023)
摘要
Lithium-ion batteries are widely used in electric vehicle powertrain systems. As batteries age, their state of health (SOH), indicated by their usable capacity and power capability, decreases. For reliable battery operation, accurate estimation and prediction of SOH are essential. This paper proposes an algorithm for estimating battery capacity SOH from an open-source fast charging dataset with many different charge profile types. Histogram data is created from the measured time domain data and fed into a feedforward neural network (FNN). To capture the impact of different charge profiles on aging, current and state of charge (SOC) are multiplied together to create an additional synthetic input to the estimator. To reduce the number of inputs to the FNN to only those that contain valuable information, we use principal component analysis to reduce the total number of inputs by 80%. An SOH algorithm is proposed that can estimate capacity throughout the battery's life with a 1.03% root mean square percentage error (RMSPE) and 0.68% mean absolute percentage error (MAPE).
更多查看译文
关键词
battery capacity SOH estimation,charge profiles,electric vehicle powertrain systems,feedforward neural network,FNN,histogram data,LiJk/int,LiJkJk/int,lithium-ion battery state-of-health,machine learning,MAPE,mean absolute percentage error,open-source fast charging dataset,power capability,principal component analysis,reliable battery operation,RMSPE,root mean square percentage error,SOC,SOH algorithm,state of charge,synthetic input,time domain data
AI 理解论文
溯源树
样例
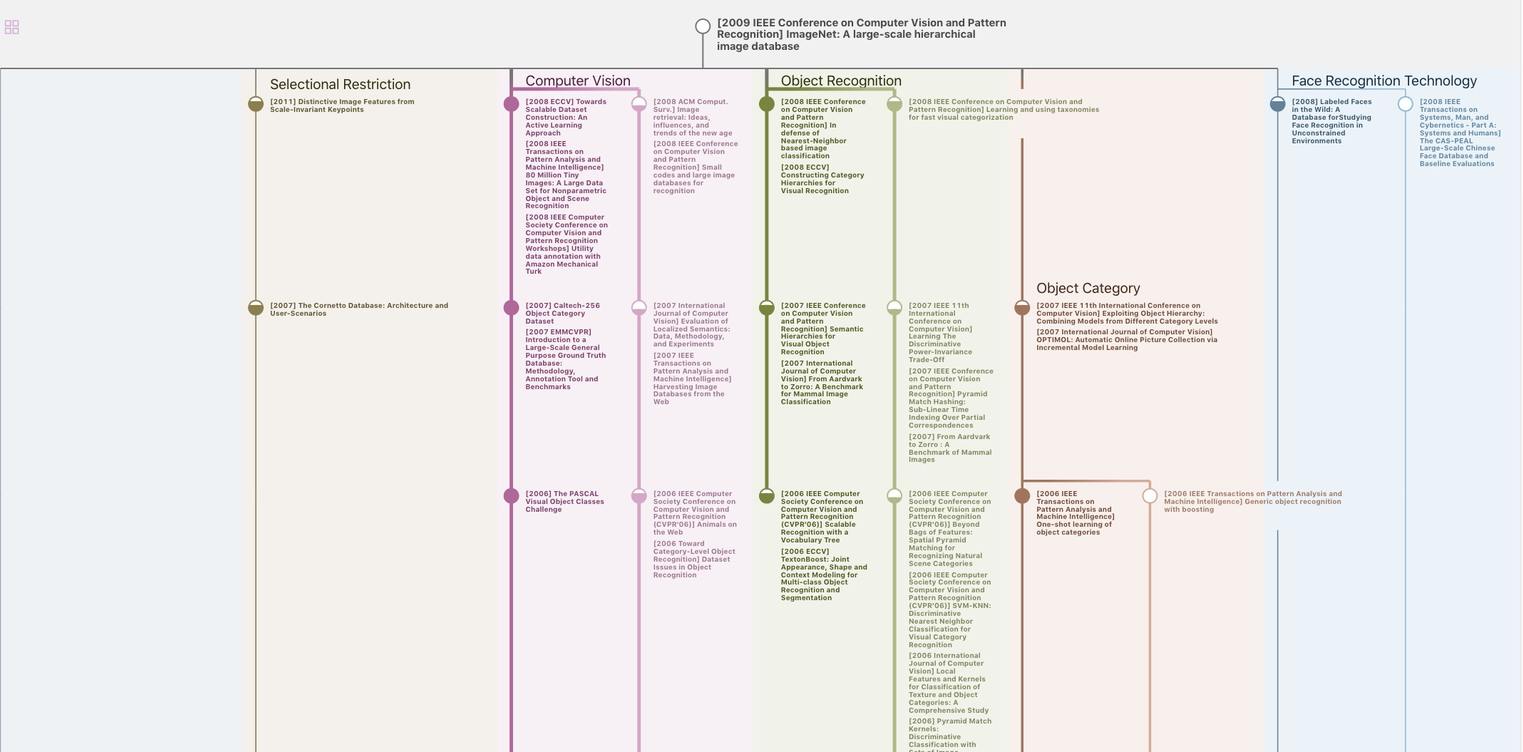
生成溯源树,研究论文发展脉络
Chat Paper
正在生成论文摘要