A Deep Convolution Multi-Adversarial adaptation network with Correlation Alignment for fault diagnosis of rotating machinery under different working conditions
ENGINEERING APPLICATIONS OF ARTIFICIAL INTELLIGENCE(2023)
摘要
Domain adaptation (DA) approaches have been extensively applied to the diagnosis of rotating machinery faults under different working conditions. However, most DA-based methods perform poorly in practical situations since they generally only consider the global distribution or subdomain distribution of the source and target domains. Thus, we propose a novel Deep Convolution Multi-Adversarial adaptation network with Correlation Alignment (DCMACA). DCMACA consists of an improved deep convolutional feature extractor, a domain adaptation module, and a label classifier. The improved deep convolutional feature extractor comprises ordinary convolutional layers, depthwise convolution layers, Squeeze and Excitation modules, skip connection operations, an average pooling layer, and a fully connected layer. The domain adaptation module introduces multiple domain discriminators and Coral distance to align the subdomain distribution and global distribution of features extracted by the feature extractor, respectively. The softmax function is employed as the label classifier. Based on DCMACA, we presented a new approach for identifying faults in rotating machinery under different operating conditions. First, the original vibration signals are converted into the time-frequency maps of size 64 × 64 via the continuous wavelet transform and bilinear interpolation technologies. Subsequently, the time-frequency maps are input to DCMACA to complete the extraction of transferable features and fault identification. The proposed DCMACA fault identification approach was evaluated through two experiments, where it achieved an average accuracy of 98.84% in 18 migration diagnostic tasks. The comprehensive results reveal that the presented approach can realize higher diagnostic accuracies, robustness, and superior generalization capability compared to the existing mainstream DA approaches.
更多查看译文
关键词
Fault diagnosis, Subdomain adaptation, Global adaptation, Multiple domain discriminators, Correlation alignment
AI 理解论文
溯源树
样例
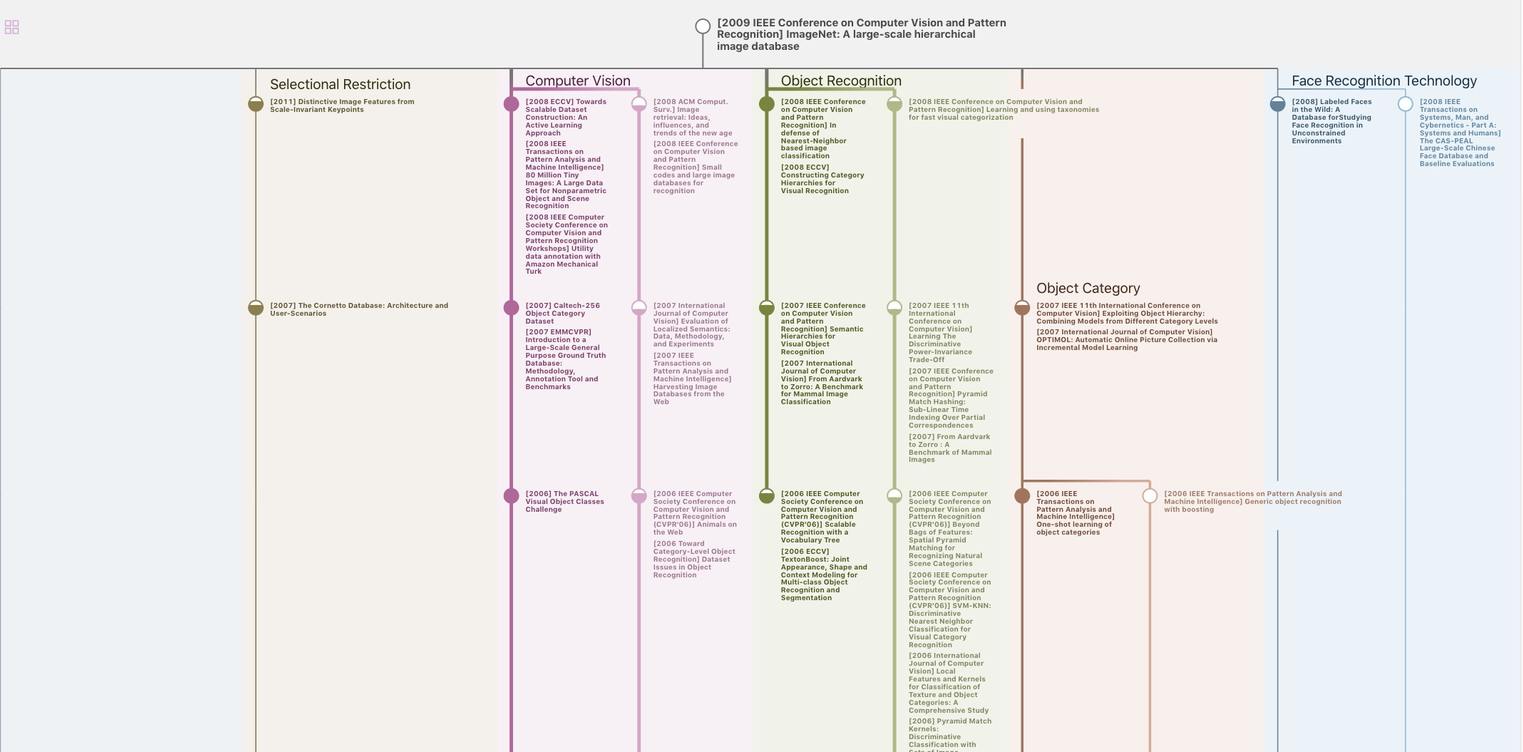
生成溯源树,研究论文发展脉络
Chat Paper
正在生成论文摘要