Novel automated detection of sports activities using shadow videos
MULTIMEDIA TOOLS AND APPLICATIONS(2023)
摘要
The aim of this work is to investigate the use of shadow videos for daily sports activity detection and to contribute to the emerging field of shadow-based classification in machine learning. A novel deep feature engineering model is proposed, and a new shadow video dataset is collected to validate the proposed model. Furthermore, the use of shadow videos ensures privacy protection for individuals. To evaluate the proposed system, a dataset comprising five sports activities (i.e., squat, steady run, standing butterfly, overhead side bend, and knee lift) recorded from 33 participants is used. The proposed model works in the following way: (i) videos are divided into frames and aggregated into non-overlapping blocks of six frames to create images, (ii) deep features are extracted from three fully connected layers of the pre-trained AlexNet, resulting in 4096, 4096, and 1000 features from fc6, fc7, and fc8 layers, respectively. These three feature vectors are then merged to generate a final feature vector with a length of 9192 (= 4096 + 4096 + 1000). (iii) The Chi2 selector is employed to select the most distinctive 1000 features in the feature selection phase, and (iv) the support vector machine (SVM) with leave-one-subject-out (LOSO) validation is used to classify the five sports activities. The proposed deep features coupled with Chi2 based model achieved a classification accuracy of 88.49% using the SVM classifier with LOSO cross-validation (CV) on our collected dataset.
更多查看译文
关键词
sports activities,shadow videos,detection
AI 理解论文
溯源树
样例
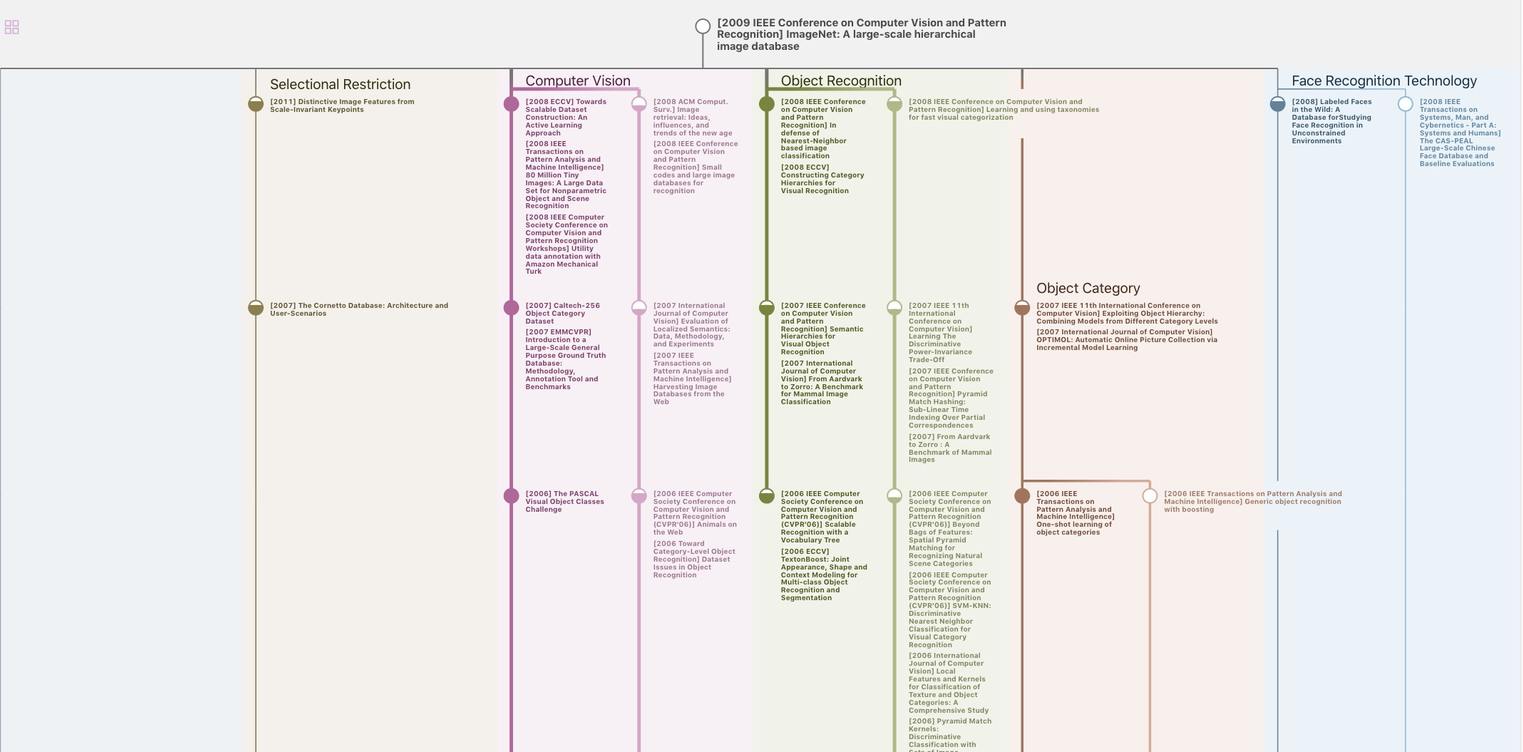
生成溯源树,研究论文发展脉络
Chat Paper
正在生成论文摘要