Natural gas pipeline leak detection based on acoustic signal analysis and feature reconstruction
APPLIED ENERGY(2023)
摘要
The natural gas pipeline leakage detection task based on acoustic signal has some problems such as background noise coverage, lack of effective features, and low fault identification accuracy caused by small sample data. However, only one of these problems was usually studied in previous technologies. Almost no one has attempted to challenge multiple issues at the same time. In this study, a natural gas pipeline leak detection model that integrates acoustic feature processing techniques and feature reconstruction is proposed to resolve the above problems collaboratively. This model consists of two components. The first component is a feature processing technique of the acoustic signal that integrates frequency domain vector denoising and time domain associative function feature enhancement. The second component is a one-dimensional convolutional neural network with an expanded structural feature encoder (FAE) for feature reconstruction (FAE-1D-CNN). In the feature processing stage of the acoustic signal, firstly, the acoustic signal collected by the acoustic sensor is discretized into a digital signal. Secondly, the energy modal function is used to perform high/low energy modal clustering of digital signal features. The feature validity is enhanced by adding association factors to the low-energy modal features matrix. A low-pass filtering method is then used in the high-energy modal features to remove the background noise coverage of the high-frequency components. In the fault feature extraction stage, a feature encoder (FAE) is introduced in the 1D-CNN network to extract effective fault features while performing secondary reconstruction of local spatial features, addressing the problem of small sample leakage signals with few effective fault characteristics. The global average pooling layer is used instead of the fully connected layer, and the Softmax function is adopted as the classifier for fault discrimination. The performance of the proposed method was evaluated on the GPLA-12 dataset, and the fault identification accuracy is up to 95.17%. Compared with other competing methods, the method in this paper exhibits optimal performance and has broad application prospects.
更多查看译文
关键词
acoustic signal analysis,gas pipeline
AI 理解论文
溯源树
样例
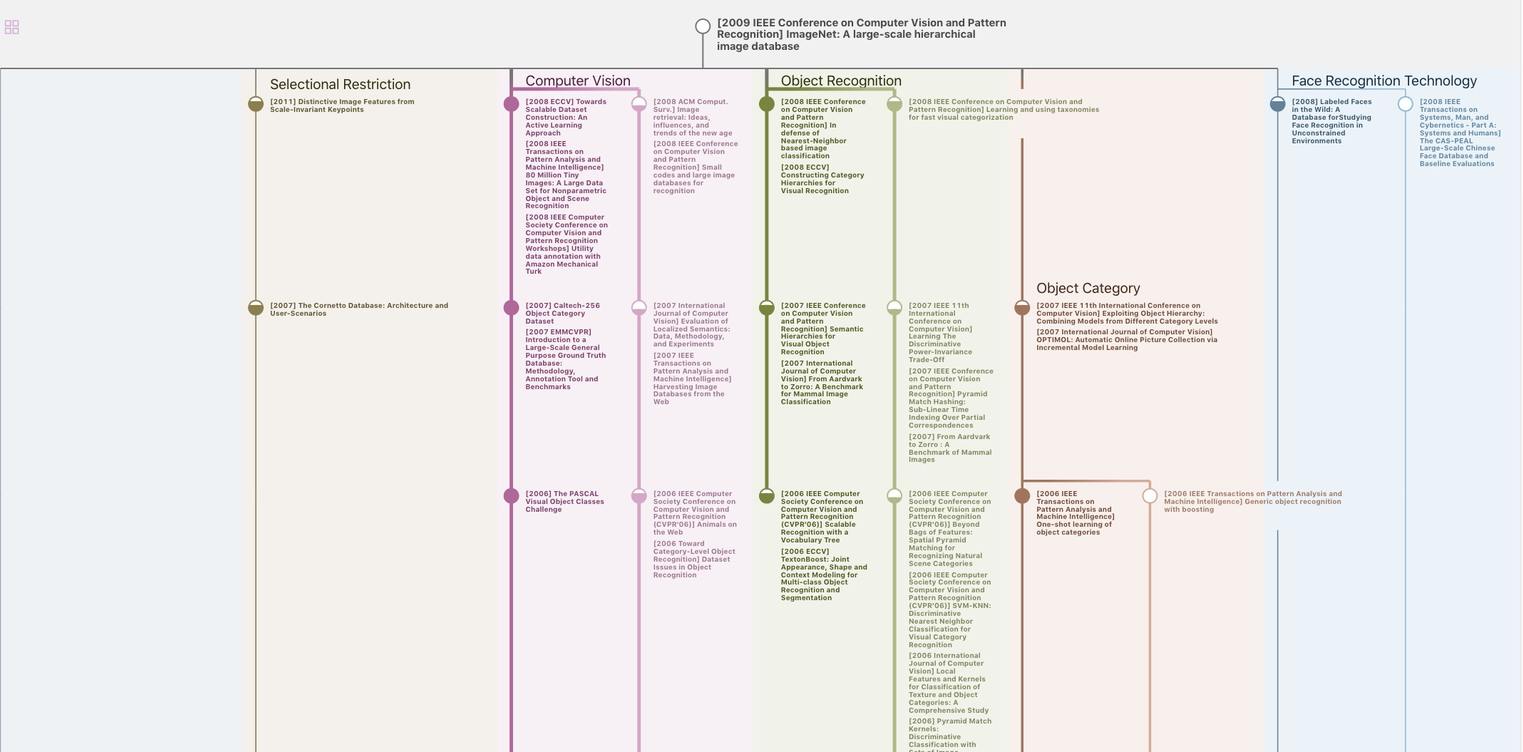
生成溯源树,研究论文发展脉络
Chat Paper
正在生成论文摘要