Rough-Fuzzy Graph Learning Domain Adaptation for Fake News Detection
IEEE TRANSACTIONS ON COMPUTATIONAL SOCIAL SYSTEMS(2023)
摘要
The widespread dissemination of fake news across the internet has profound detrimental consequences for society, governments, and citizens. To address this pressing issue, numerous machine learning-based models have been developed for detecting fake news. However, the challenge of acquiring sufficient labeled news data in a new domain, coupled with the presence of inconsistent data distribution, necessitates the integration of unsupervised domain adaptation (DA) methods to enhance the reliability of cross-domain fake news detection. In this article, a rough-fuzzy graph learning DA for fake news detection is proposed. First, a rough-fuzzy graph learning method is proposed to effectively handle the representation of cross-domain sample uncertainty structural information, thereby learning a more discriminative subspace. Second, a rough-fuzzy region division strategy is designed to perform different analysis on target domain samples, thus achieving a more accurate description of the relationships between cross-domain samples. Furthermore, considering that domain private features may negatively affect the knowledge transfer process, a sparse structure preserving strategy is proposed to better capture shared general features across domains. Experimental evaluations conducted on three news datasets demonstrate the efficacy of the proposed method in cross-domain fake news detection.
更多查看译文
关键词
Domain adaptation (DA),fake news detection,fuzzy sets,graph learning,rough sets,sparse structure
AI 理解论文
溯源树
样例
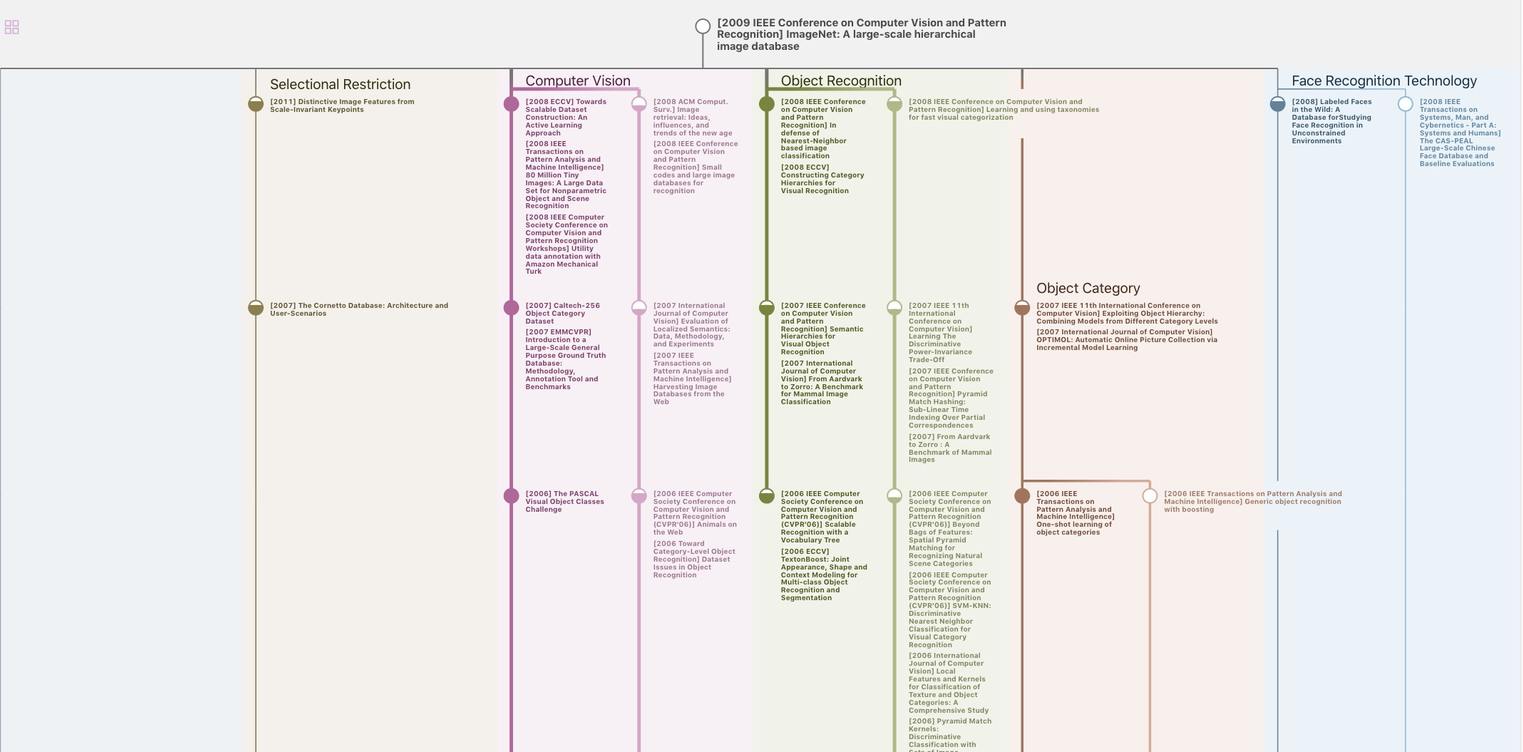
生成溯源树,研究论文发展脉络
Chat Paper
正在生成论文摘要