Threshold effect of data amount and grid size on urban land use type identification using multi-source data fusion
SUSTAINABLE CITIES AND SOCIETY(2023)
摘要
Reliable urban land use maps are important for sustainable development and planning. Currently, the effects of different data source combinations and grid sizes on mapping results have rarely been studied. To reduce subjectivity in data selection, 10 collected multi-source spatial data were combined by traversal to create 1013 simulated combination schemes. Considering the size range of these data sources, 10 fusion grid sizes were selected. Then, a multi-source data learning model for urban land use classification (ULUC) was established by combining convolutional neural networks and long short-term memory. By taking Jinshui District (Zhengzhou, China) as an example, 10130 ULUC mappings were obtained. The maximum accuracy (82.9%) was achieved in the combination scheme D1D2D3D5D6D7D8D9D10 at a grid size of 30 m. The optimal solution among simulation 10130 schemes had an accuracy of 82.9%, a 14.7% improvement compared to the average accuracy of 67.6%. It is found that (1) The maximum accuracy showed a tendency to increase and then decrease with the increase in the variety of multi-source data combinations;(2) As the grid size decreases, the maximum accuracy also exhibited a tendency to increase and then decrease; (3) There was a significant threshold effect for both data combination types and grid sizes.
更多查看译文
关键词
City functional area,Variable grid size,Variable data combination,Deep learning,Sustainable land development policies
AI 理解论文
溯源树
样例
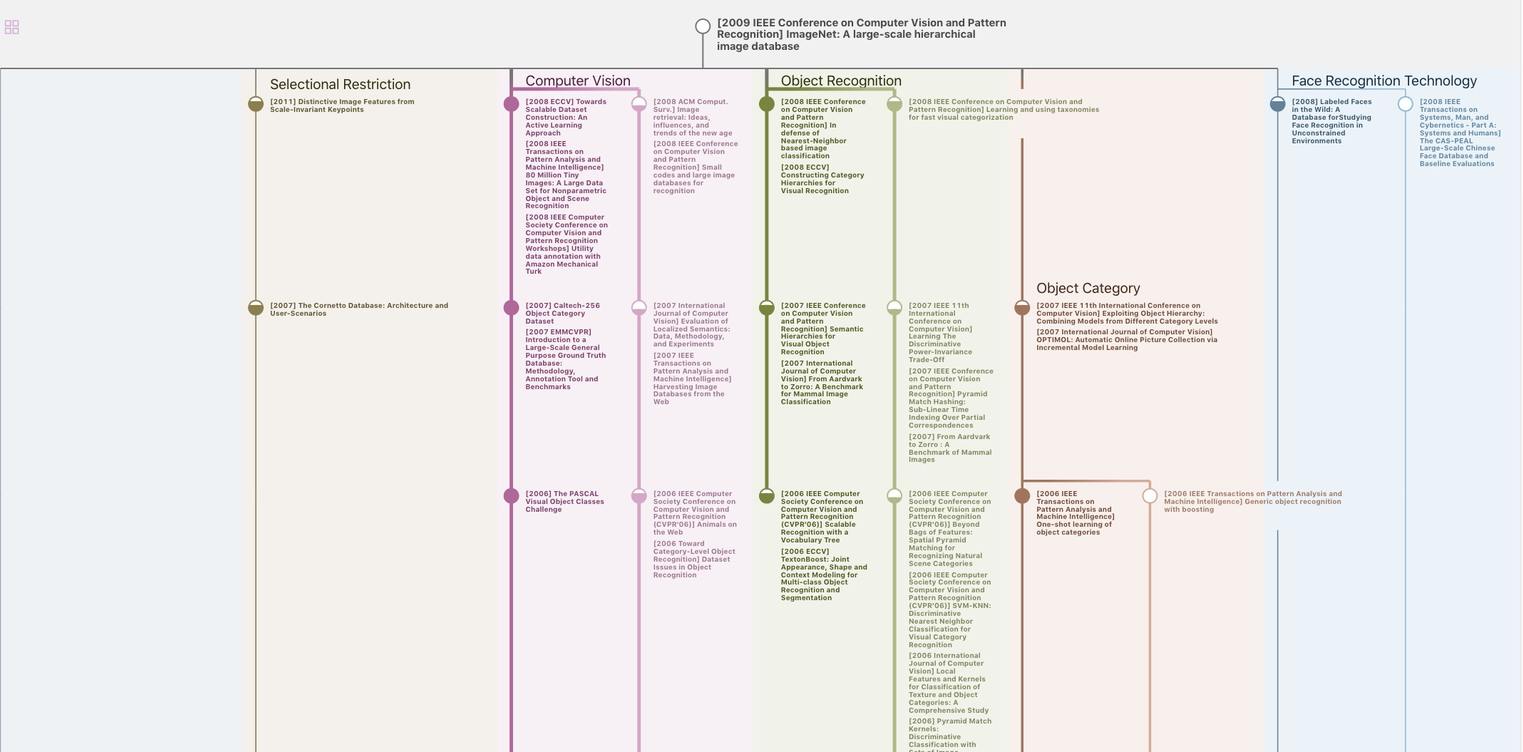
生成溯源树,研究论文发展脉络
Chat Paper
正在生成论文摘要