Application of a Pre-Trained CNN Model for Fault Interpretation in the Structurally Complex Browse Basin, Australia
APPLIED SCIENCES-BASEL(2023)
摘要
Fault detection is an important step in subsurface interpretation and reservoir characterization from 3D seismic images. Due to the numerous and complex fault structures in seismic images, manual seismic interpretation is time-consuming and requires intensive work. We applied a pre-trained CNN model to predict faults from the 3D seismic volume of the Poseidon field in the Browse Basin, Australia. This field is highly structured with complex normal faulting throughout the targeted Plover Formations. Our motivation for this work is to compare machine-learning-based fault prediction to user-interpreted fault identification supported by seismic variance attributes. We found reasonably satisfactory results using CNN with an improved fault probability volume that outperforms variance technology. Therefore, we propose that this workflow could reduce time and be able to predict faults quite accurately in most structurally complex areas.
更多查看译文
关键词
fault detection, reservoir characterization, seismic images, convolutional neural network, variance attribute
AI 理解论文
溯源树
样例
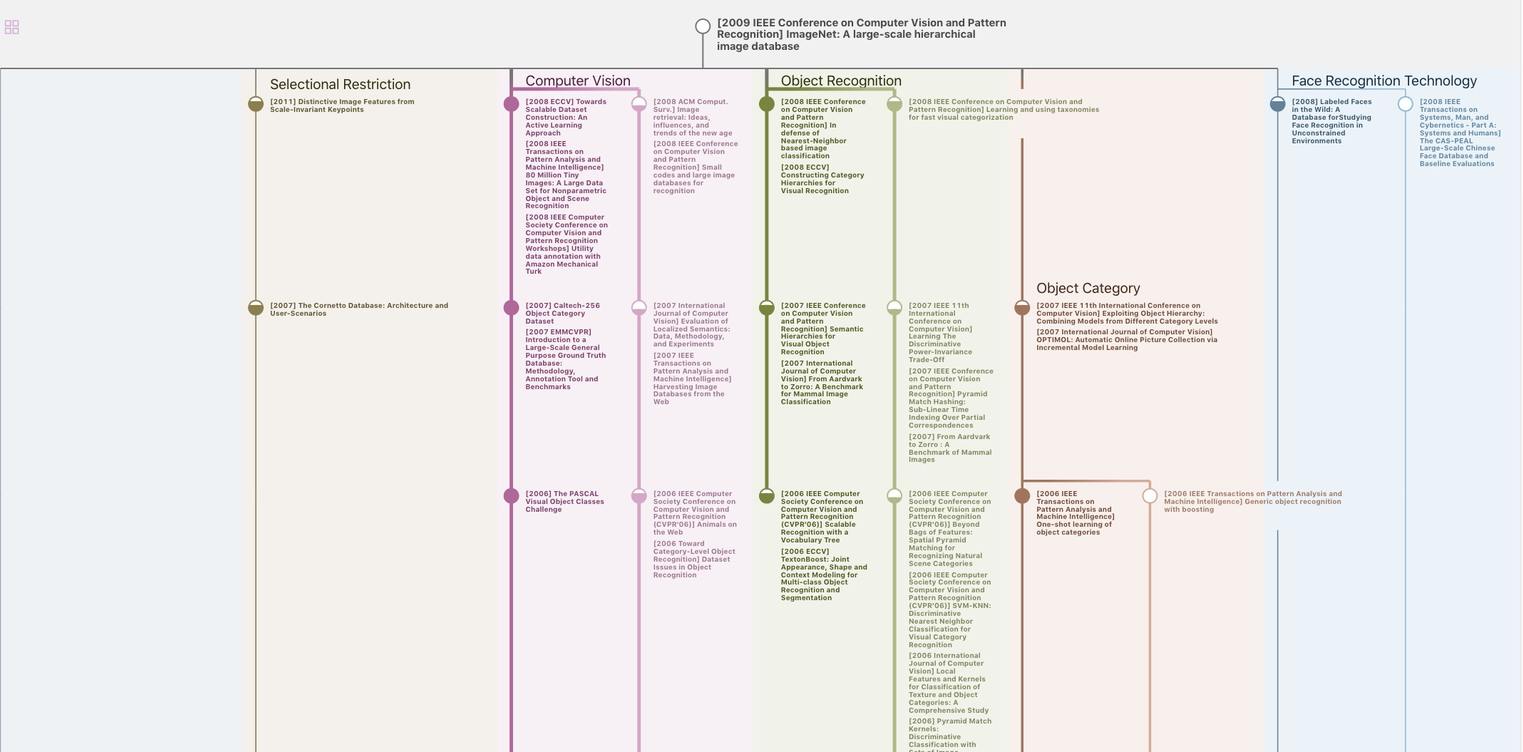
生成溯源树,研究论文发展脉络
Chat Paper
正在生成论文摘要