Predicting Trending Elements on Web Pages Using Machine Learning
INTERNATIONAL JOURNAL OF HUMAN-COMPUTER INTERACTION(2023)
摘要
Eye-tracking data can be used to understand how users interact with web pages. Understanding the eye-movement sequences of multiple users is a challenging task because the sequence followed by each user tends to be different. Scanpath Trend Analysis (STA) brings multiple individual eye-movement sequences together and identifies a representative sequence as a trending path. However, eye-tracking data on a web page is required to determine the trending path. Our aim here is to investigate whether we can train Machine Learning (ML) algorithms to identify trending elements on a web page without collecting eye-tracking data on that web page. This article presents our experiments with different ML classification algorithms towards achieving that goal. To validate the experiments, we used two datasets from previous research, the first one included browsing and searching tasks and the second one included browsing and synthesis tasks. Our experiments show that the k-nearest neighbors algorithm (KNN) model can successfully identify the trending elements in the first dataset for both browsing (F1=approximate to 91%) and searching tasks (F1=approximate to 88%). However, the second dataset's synthesis task results were not as successful as its browsing task results. Our work here shows that a model can be created to predict the trending elements in web pages solely with web page features but the task is a critical factor in the success of prediction.
更多查看译文
关键词
machine learning,web pages,predicting
AI 理解论文
溯源树
样例
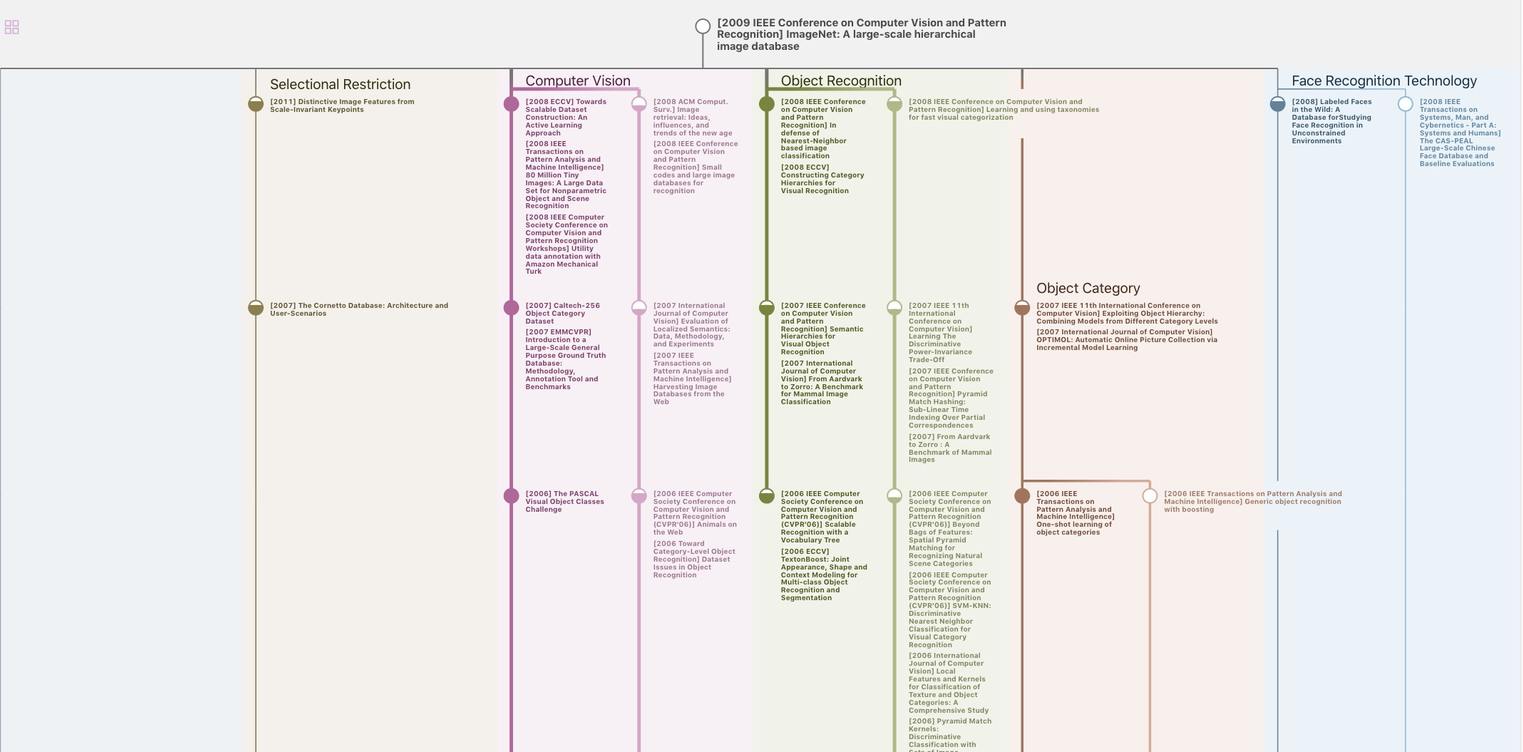
生成溯源树,研究论文发展脉络
Chat Paper
正在生成论文摘要