A multi-strategy surrogate-assisted competitive swarm optimizer for expensive optimization problems
APPLIED SOFT COMPUTING(2023)
摘要
Evolutionary computation is a powerful tool for solving nonconvex optimization problems. Generally, evolutionary algorithms take numerous fitness evaluations to obtain the potential optimal solutions. This poses a critical challenge for applying them to real-world complex engineering optimization problems. Recently, surrogate-assisted evolutionary algorithms (SAEAs) have attracted an increasing amount of research. In this paper, a surrogate-assisted competitive swarm optimizer (SACSO) is proposed to exploit the potential of evolutionary algorithms to handle expensive optimization problems. In SACSO, global search, local search and opposition-based search are implemented as three different criteria to select the appropriate particle for realistic fitness evaluation. In order to trade off global exploitation and local exploration, a dynamic adaptation strategy is also proposed in this paper. Search approaches are dynamically adjusted to select a particle or perform variations at different stages of the algorithm. The combination of generalized surrogate model (GSM) with global search and elite surrogate model (ESM) with local and opposition-based search, effectively enhances the optimal performance of SACSO. The proposed SACSO is comprehensively compared with the state-of-the-art SAEAs and well-known EAs on seven benchmark functions. Additionally, SACSO is applied to the speed reducer design optimization problem. Experimental simulation results suggest SACSO is a prospective tool for dealing with expensive optimization problems.(c) 2023 Elsevier B.V. All rights reserved.
更多查看译文
关键词
Surrogate-assisted,Competitive swarm optimizer,Radial basis function,Expensive optimization
AI 理解论文
溯源树
样例
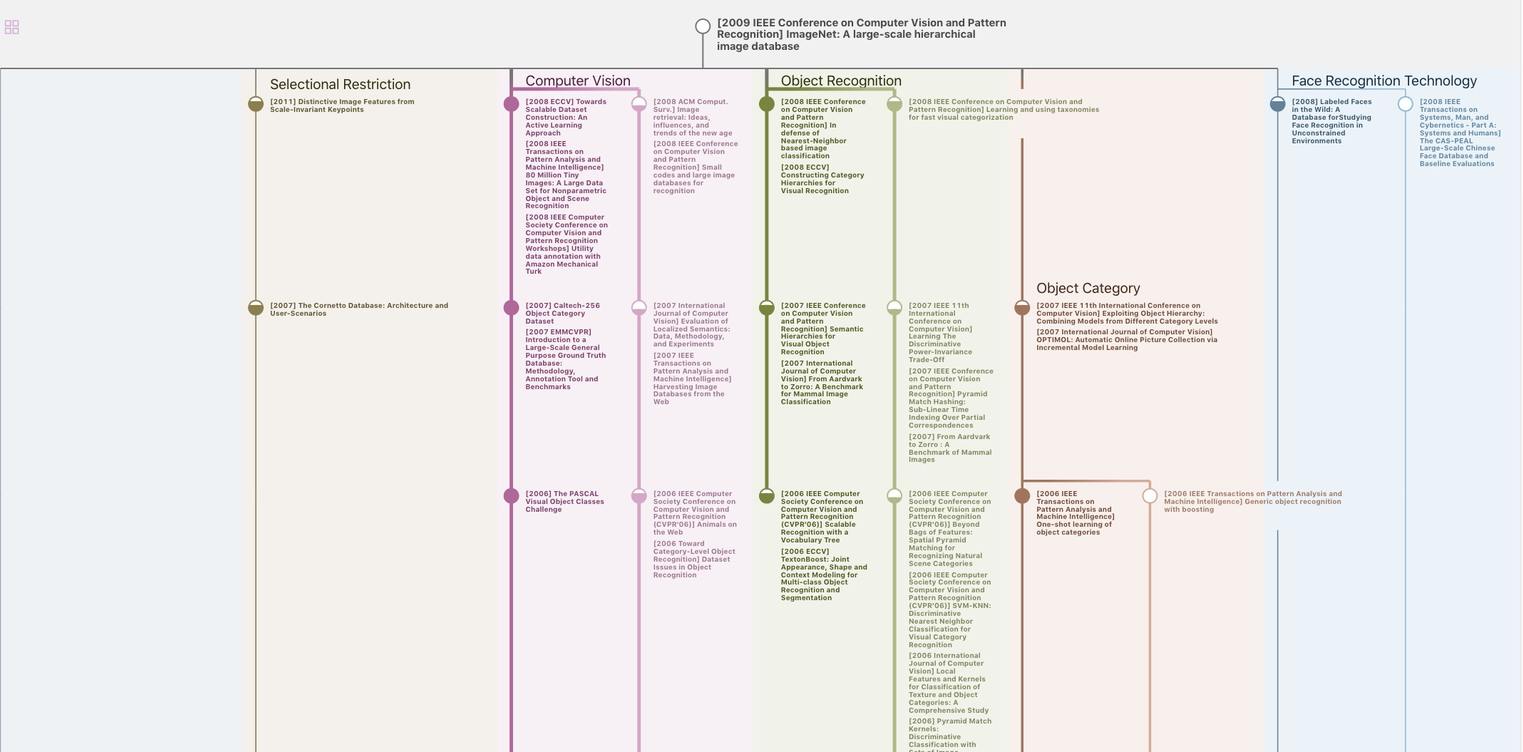
生成溯源树,研究论文发展脉络
Chat Paper
正在生成论文摘要