Efficient channel attention residual learning for the time-series fault diagnosis of wind turbine gearboxes
MEASUREMENT SCIENCE AND TECHNOLOGY(2024)
摘要
Due to harsh and variable working conditions, the wind turbine gearbox may be damaged during the operation, resulting in an extended downtime with reduced productivity and economic loss. This calls for efficient fault diagnostics for the wind turbine gearboxes. Commonly-used diagnostics based on classical deep learning networks cannot guarantee good performance with time series signals due to the weakness of feature extraction. For this reason, an efficient channel attention residual learning approach is proposed to enhance the feature extraction and fault diagnosis for wind turbine gearboxes, leading to the development of a channel attention residual network (CAResNet). The collected time series signals are directly employed as the input. The efficient channel attention is embedded into the residual network to capture features and improve fault diagnosis capability. Experiments are carried out on a real wind turbine gearbox. The results showed that the average diagnostic accuracy of the CAResNet model was 94.41%. CAResNet has better diagnostic accuracy than other methods proposed in this paper.
更多查看译文
关键词
wind turbine,gearbox,channel attention residual network,time series,fault diagnosis
AI 理解论文
溯源树
样例
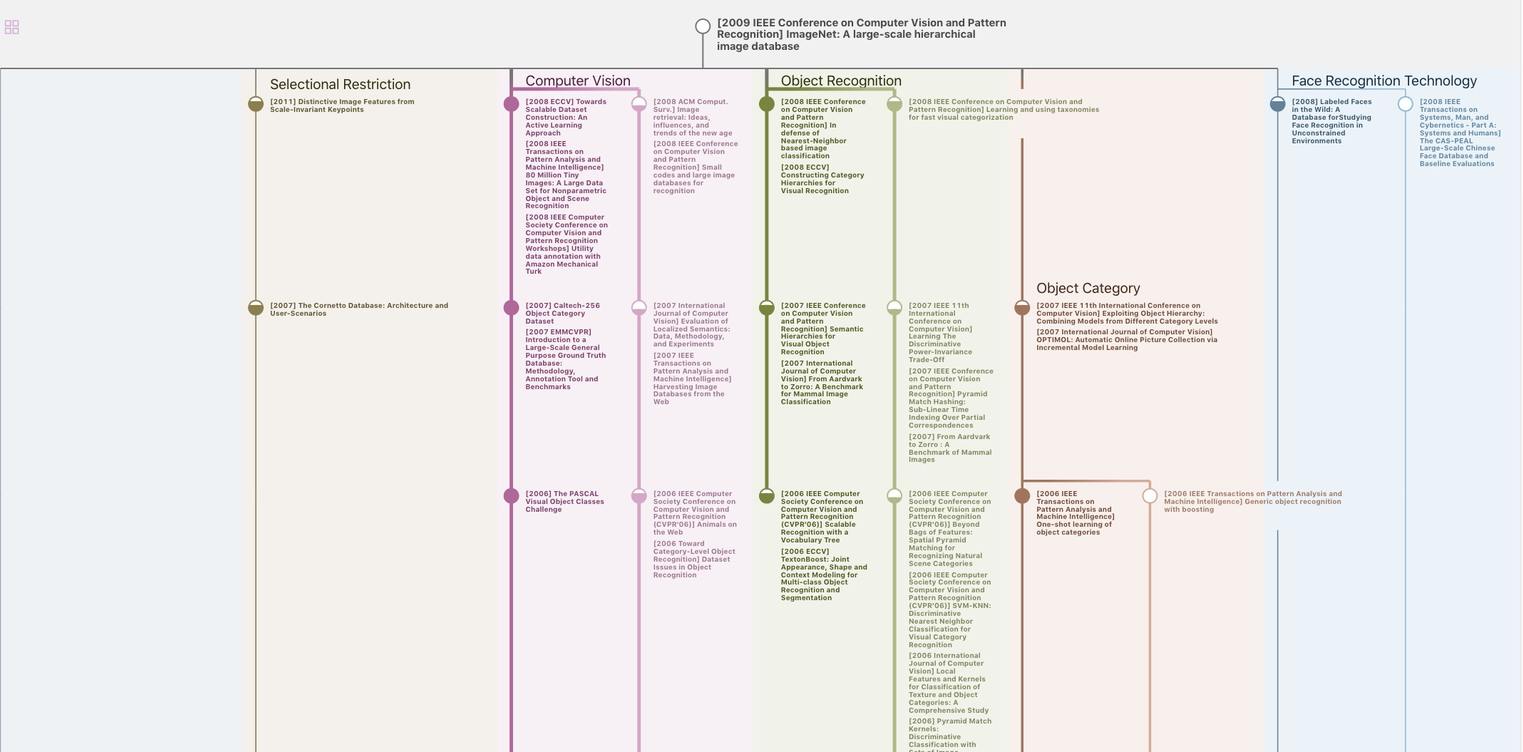
生成溯源树,研究论文发展脉络
Chat Paper
正在生成论文摘要