Optimizing proportional balance between supervised and unsupervised features for ultrasound breast lesion classification
BIOMEDICAL SIGNAL PROCESSING AND CONTROL(2024)
摘要
Computer-aided-diagnosis systems have led to transformative advancements to breast ultrasound interpretations, surmounting the challenges of manual assessments. Various methods have been explored for precisely classifying breast nodules. Notably, the channel embedding approach and the integration of characteristics from different modalities have garnered significant attention thus far. However, a serious drawback of existing methodologies lies in their reliance on mask availability or the necessity for complex preprocessing procedures to extract principal features.In this paper, we introduce a simple yet powerful lesion characterization framework that effectively addresses these shortcomings while achieving satisfactory discrimination performance. Our method simultaneously employs independent networks, trained via both supervised and unsupervised learning. Specifically, three supervised backbones are selected where the final feature volumes are progressively doubled in scale. Moreover, convolutional autoencoders are developed to perform unsupervised representation learning. By adjusting its hyperparameters, we generate multiple latent variables that align with the feature volumes of the supervised networks. Hence, our primary objective is to analyze an effective hybridization scheme driven by diverse automated representations and validate the optimized proportion of each component.Extensive ratio experiments are conducted on two public breast ultrasound datasets. The quantitative results highlight the importance of attaining a proper feature balance in hybridization. Further, consistent observations revealed the optimal ratio of 1:2 between the supervised and unsupervised volumes. We report that this condition derived a remarkable enhancement in discriminating tumor categories. To verify its robustness, our method is compared with recent competing approaches and demonstrated superiority without the need for additional specific requirements.
更多查看译文
关键词
Computer vision,Feature hybridization,Ultrasound breast lesion classification,Computer-aided-diagnosis system
AI 理解论文
溯源树
样例
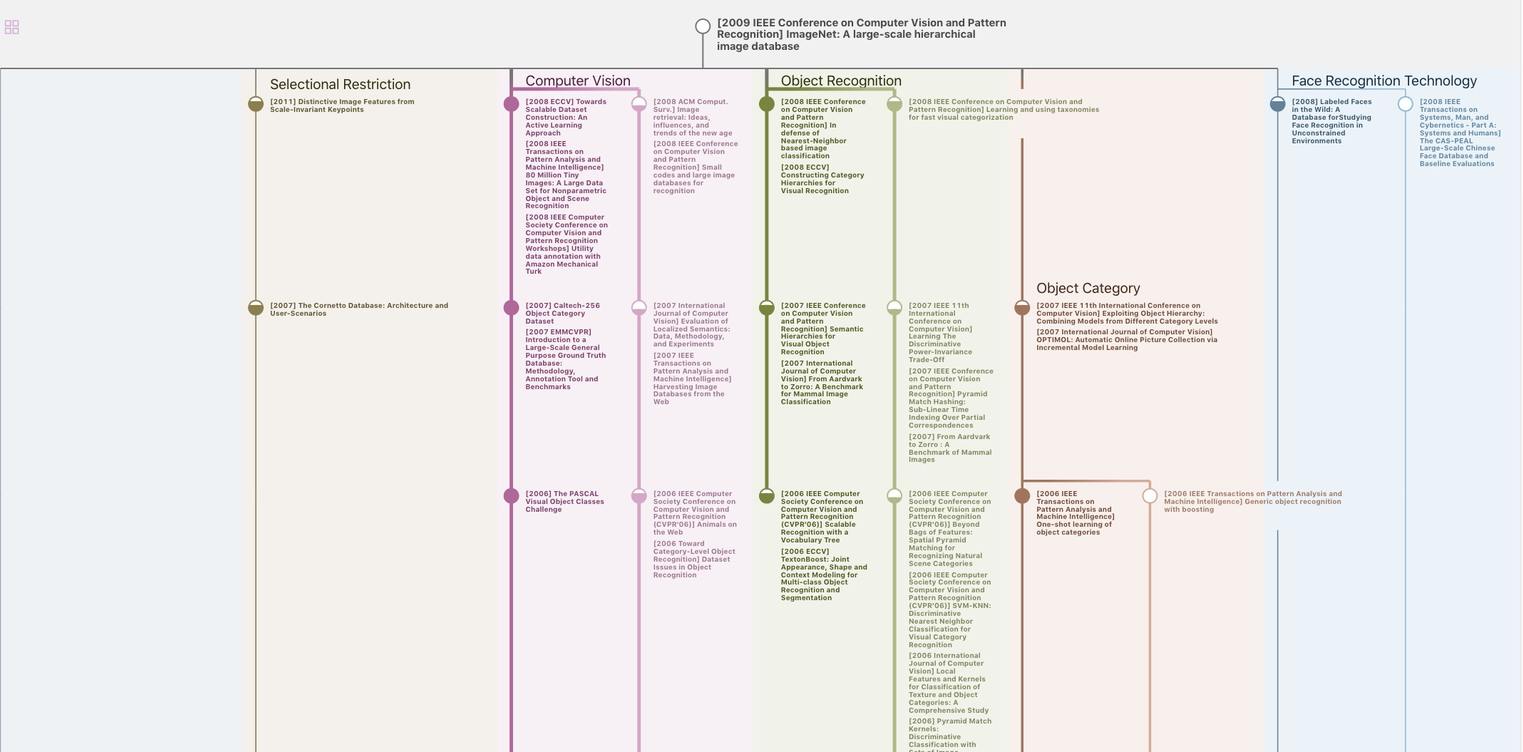
生成溯源树,研究论文发展脉络
Chat Paper
正在生成论文摘要