Mild cognitive impairment detection with optimally selected EEG channels based on variational mode decomposition and supervised machine learning
BIOMEDICAL SIGNAL PROCESSING AND CONTROL(2024)
摘要
Detecting mild cognitive impairment (MCI), which is typically the earliest stage of dementia, is essential for managing dementia. Recently, researchers have explored the use of electroencephalography (EEG) to detect MCI. EEG involves recording brain activity by placing a number of electrodes (channels) directly on the scalp. Here we present effective variational mode decomposition (VMD)-based methods for detection of MCI with optimally selected EEG channels. To achieve this, we first decompose the EEG signals from each channel into sub-signals called variational mode functions (VMFs) with different frequency bands. From each VMF, an entropy measure is used to extract a feature. Several supervised machine learning techniques are investigated to classify the resulting features and obtain full-channel classification accuracy. We assess the classification performance of the proposed methods on 19-channel EEGs recorded from 32 healthy subjects and 29 MCI patients in the resting state. In a second step, we explore the feasibility of reducing the number of EEG channels while maintaining classification accuracy by using several EEG channel selection methods. Specifically, we study the effectiveness of non dominated sorting genetic algorithm (NSGA)-based methods (multi-objective optimization methods), in addition to greedy algorithms such as back-elimination and forward-addition. Results show that selecting a few suitable channels can lead to similar or even superior accuracy compared to a classifier that utilizes all EEG channels. The results also show that the multi-objective NSGA optimization methods have a greater ability than the greedy methods to select few compatible channels. For example, 99.51% is the maximum accuracy achieved using all 19 EEG channels when processed with a combination of VMD, log energy entropy, and a K-nearest neighbor (KNN) classifier. The same accuracy is achieved with only 9 channels selected by NSGA, compared to 13 channels for greedy algorithms. With an ensemble KNN classifier, the highest accuracy, 99.81%, is achieved using 11 channels selected by an NSGA-based method. The results are encouraging, demonstrating that this brain disorder can be accurately diagnosed using a minimal number of electrodes and paving the way for its adoption in clinical practice.
更多查看译文
关键词
Channel selection,EEG,Entropy,VMD,Machine learning,MCI,Multi-objective optimization,NSGA
AI 理解论文
溯源树
样例
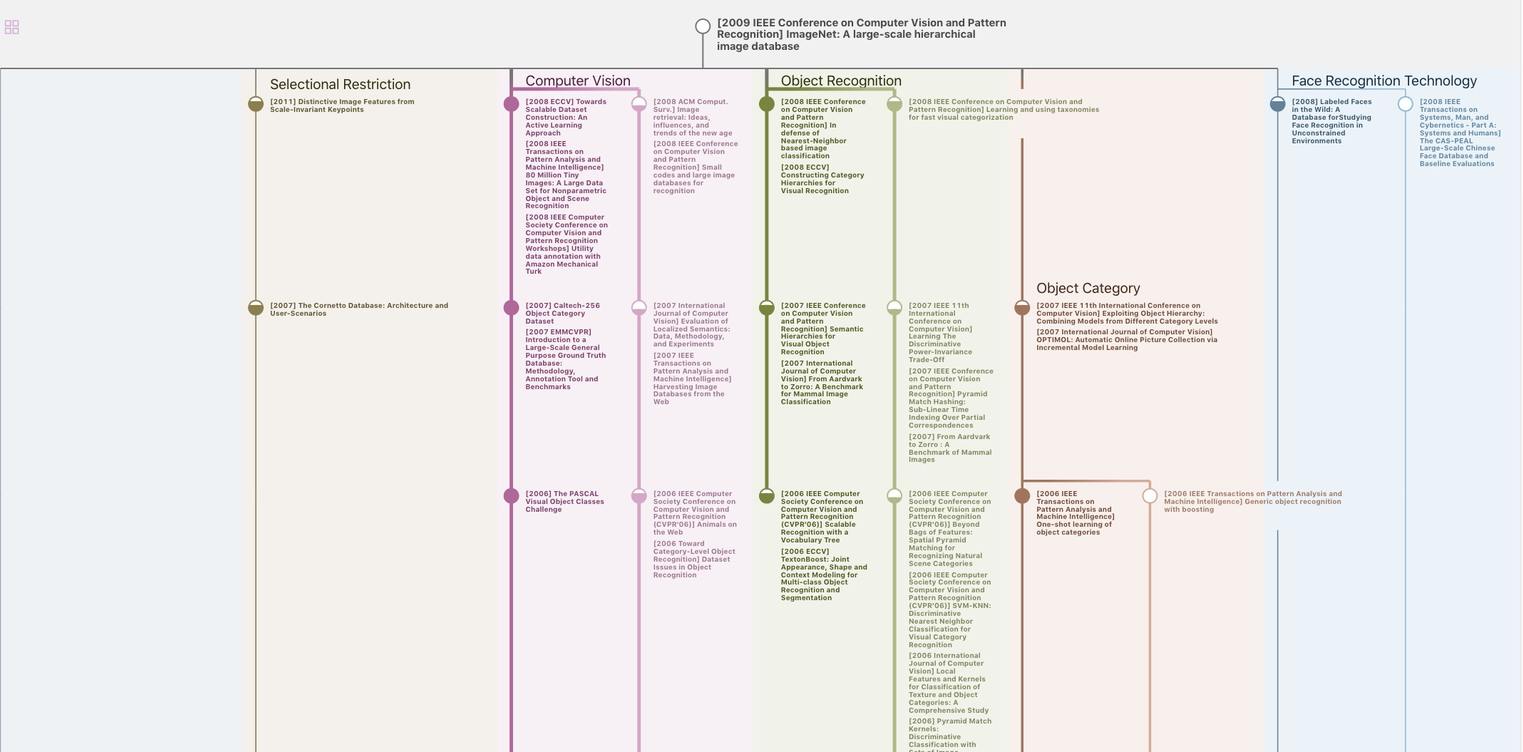
生成溯源树,研究论文发展脉络
Chat Paper
正在生成论文摘要