Asymmetric low-rank double-level cooperation for scalable discrete cross-modal hashing
EXPERT SYSTEMS WITH APPLICATIONS(2024)
摘要
As an efficient information retrieval technique, cross-modal hashing has received increasing attention. However, the remaining challenges include (1) designing effective kernelization techniques to sufficiently strengthen the connections among different samples in kernel space and improve the class separability of data; (2) learning robust compact common representations to fully extract the correlation information between different modalities; and (3) fully leveraging underlying semantic information and embedding it into optimal hash codes. To conquer these challenges, we propose a novel algorithm called Asymmetric Low-rank Double -level Cooperation Hashing (ALDCH). First, we propose a novel Nonlinear Normalized Space Kernelization submodule (NNSK) to obtain the high-quality kernelized features, which can not only capture the more powerful nonlinear structure representations but also better express the nonlinear intra-modal correlations among the original features. To learn high-quality compact representations, we further propose a novel Low-rank Double-level Cooperation Mapping submodule (LDCM) with the L21-norm constraint, which can enhance the correlation of the coefficient spaces from different modalities and enable the samples to learn constrained and compact hash representations. Besides, our proposed method fully utilizes the underlying semantic label information by introducing the Semantic Pairwise Correlation Learning submodule (SPCL). Extensive experiments conducted on benchmark datasets demonstrate the accuracy and efficiency of ALDCH, which outperforms many state-of-the-art methods.
更多查看译文
关键词
Cross-modal hashing,Compact common representations,Double-level cooperation,Nonlinear normalized space kernelization
AI 理解论文
溯源树
样例
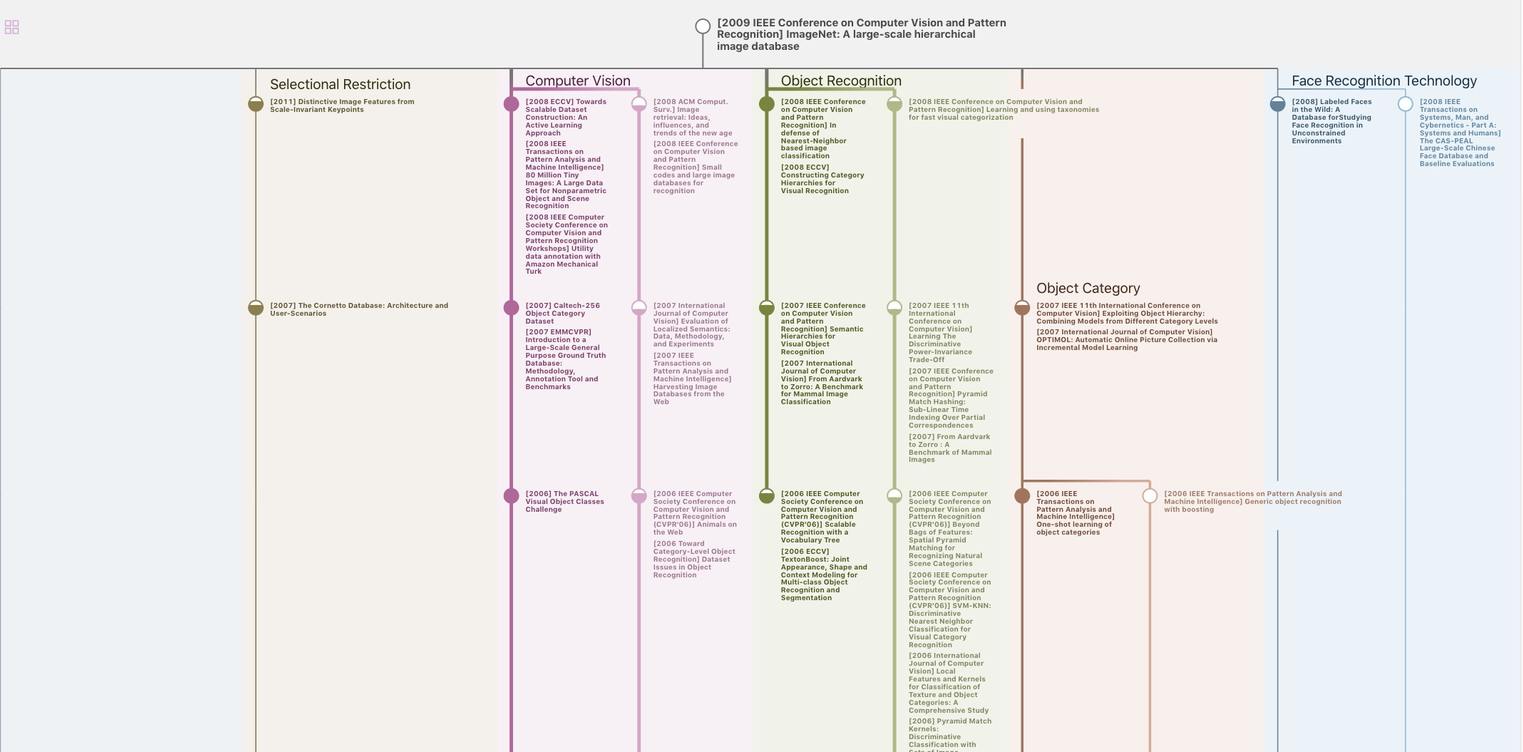
生成溯源树,研究论文发展脉络
Chat Paper
正在生成论文摘要