Comparison of multi-task approaches on molecular property prediction
CHINESE JOURNAL OF CHEMICAL PHYSICS(2023)
摘要
With the bloom of deep learning algorithms, various models have been widely utilized in quantum chemistry calculation to design new molecules and explore molecular properties. However, limited studies focus on multi-task molecular property prediction, which offers more efficient ways to simultaneously learn different but related properties by leveraging the inter-task relationship. In this work, we apply the hard parameter sharing framework and advanced loss weighting methods to multi-task molecular property prediction. Based on the performance comparison between single-task baseline and multi-task models on several task sets, we find that the prediction accuracy largely depends on the inter-task relationship, and hard parameter sharing improves the performance when the correlation becomes complex. In addition, we show that proper loss weighting methods help achieve more balanced multi-task optimization and enhance the prediction accuracy. Our additional experiments on varying amount of training data further validate the multi-task advantages and show that multi-task models with proper loss weighting methods can achieve more accurate prediction of molecular properties with much less computational cost.
更多查看译文
关键词
molecular property prediction,multi-task
AI 理解论文
溯源树
样例
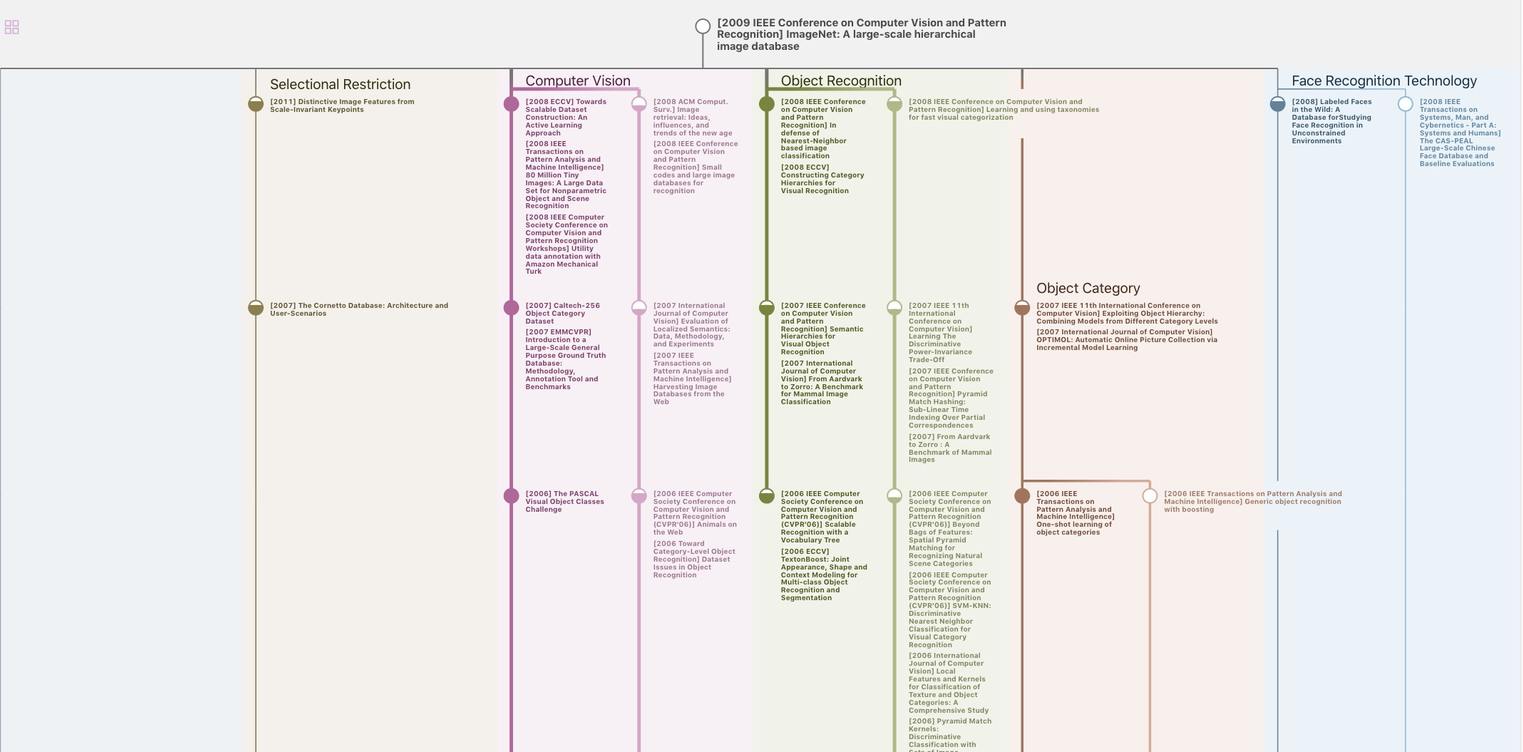
生成溯源树,研究论文发展脉络
Chat Paper
正在生成论文摘要