Identifying salient features of cooling energy usage of commercial buildings using explainable artificial intelligence
ADVANCES IN BUILDING ENERGY RESEARCH(2023)
摘要
Electrical energy usage of commercial buildings (over 60% in the USA) is of growing concern due to its impact on the environment. To reduce it, it is necessary to identify the factors contributing to commercial building energy usage. Towards this, we studied the importance of various building features and their contributions on cooling energy, using two datasets of Commercial Building Energy Consumption Survey (CBECS) 2018 and 2012. For this, Shapash, an explainable artificial intelligence technique, was used, with Random Forest, to predict the cooling energy usage intensity (EUI). The two most important features for 2018 are cooling degree days and principal building activity, while cooling degree days and cooling percentage were for 2012 with 54.79% and 33.46% contributions of the total consumption respectively. The comparison of feature importance indicated that cooling degree days are the topmost important feature impacting cooling EUI and have a more significant contribution of 34.29% for 2018 and 19.68% for 2012. Overall, the most important features impacting cooling EUI were cooling degree days, principle building activity, cooling percentage and total hours open per week. The results from this study provide insights into the most significant factors influencing energy consumption and help in developing strategies for reducing it.
更多查看译文
关键词
explainable artificial intelligence,energy usage,commercial buildings
AI 理解论文
溯源树
样例
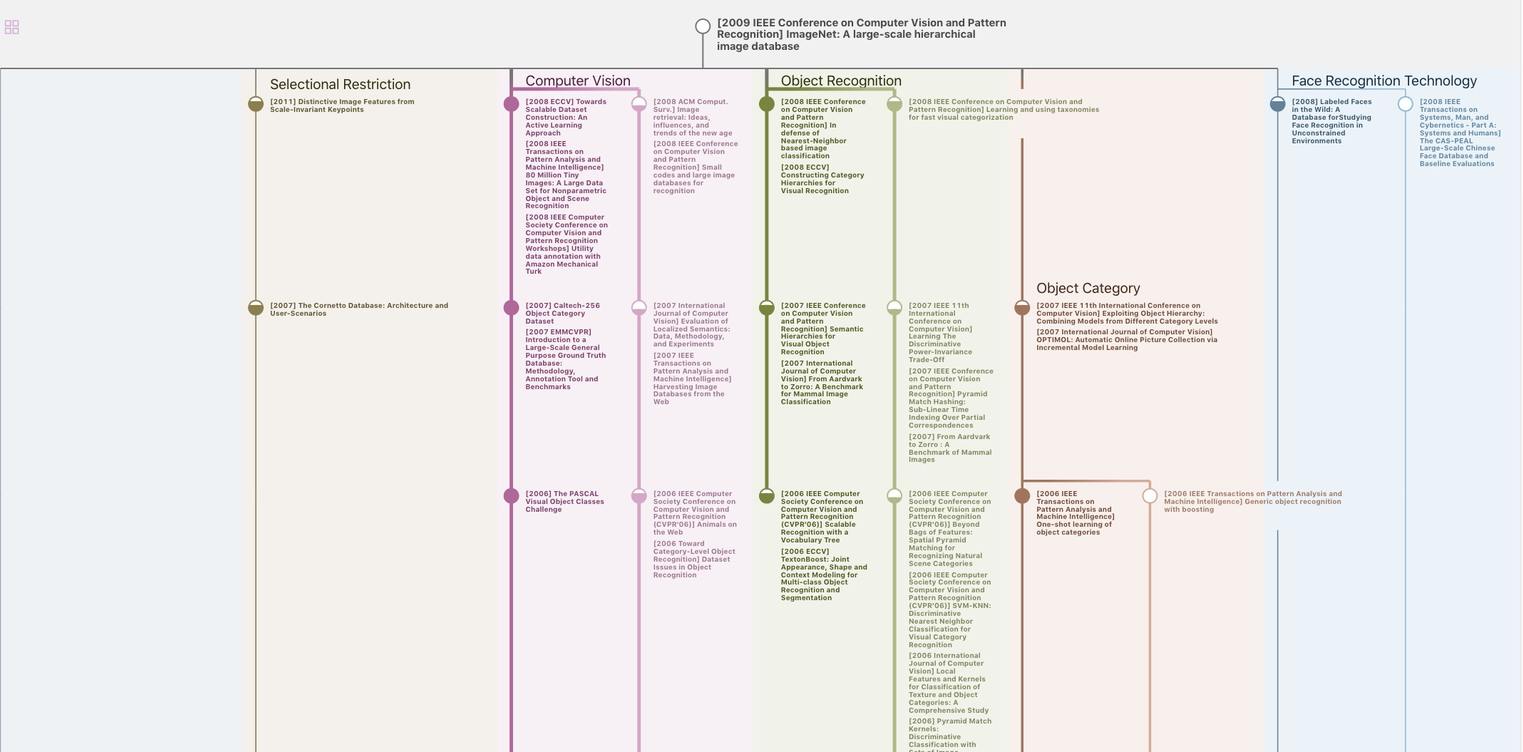
生成溯源树,研究论文发展脉络
Chat Paper
正在生成论文摘要