Saliency-Aware Dual Embedded Attention Network for Multivariate Time-Series Forecasting in Information Technology Operations
IEEE TRANSACTIONS ON INDUSTRIAL INFORMATICS(2023)
摘要
In the field of artificial intelligence for information technology operations, operational data are often modeled as aperiodic multivariate time series, which contain rich multidimensional and nonlinear patterns. However, the existing approaches are unable to effectively acquire knowledge and recognize patterns due to their reliance on processing and modeling periodic patterns. To address this issue, this article proposes a novel deep-saliency-aware dual embedded attention network for aperiodic multivariate time-series forecasting. Our network consists of three main components: 1) a convolutional-neural-network- and transformer-based component for saliency representation of the aperiodic patterns; 2) a lightweight recurrent neural network component for capturing long-term dependence features; and 3) an attention mechanism for fusing the latent representations from the former components. Extensive empirical studies are conducted on a real-world dataset and five other public datasets to evaluate the proposed network against four state-of-the-art models. The results show that our method achieves impressive high performance on most evaluation metrics. Furthermore, the data and code used in this study are publicly available, which can facilitate progress in the community.
更多查看译文
关键词
Attention mechanism,deep neural network (DNN),feature fusion,multivariate time series,time-series prediction
AI 理解论文
溯源树
样例
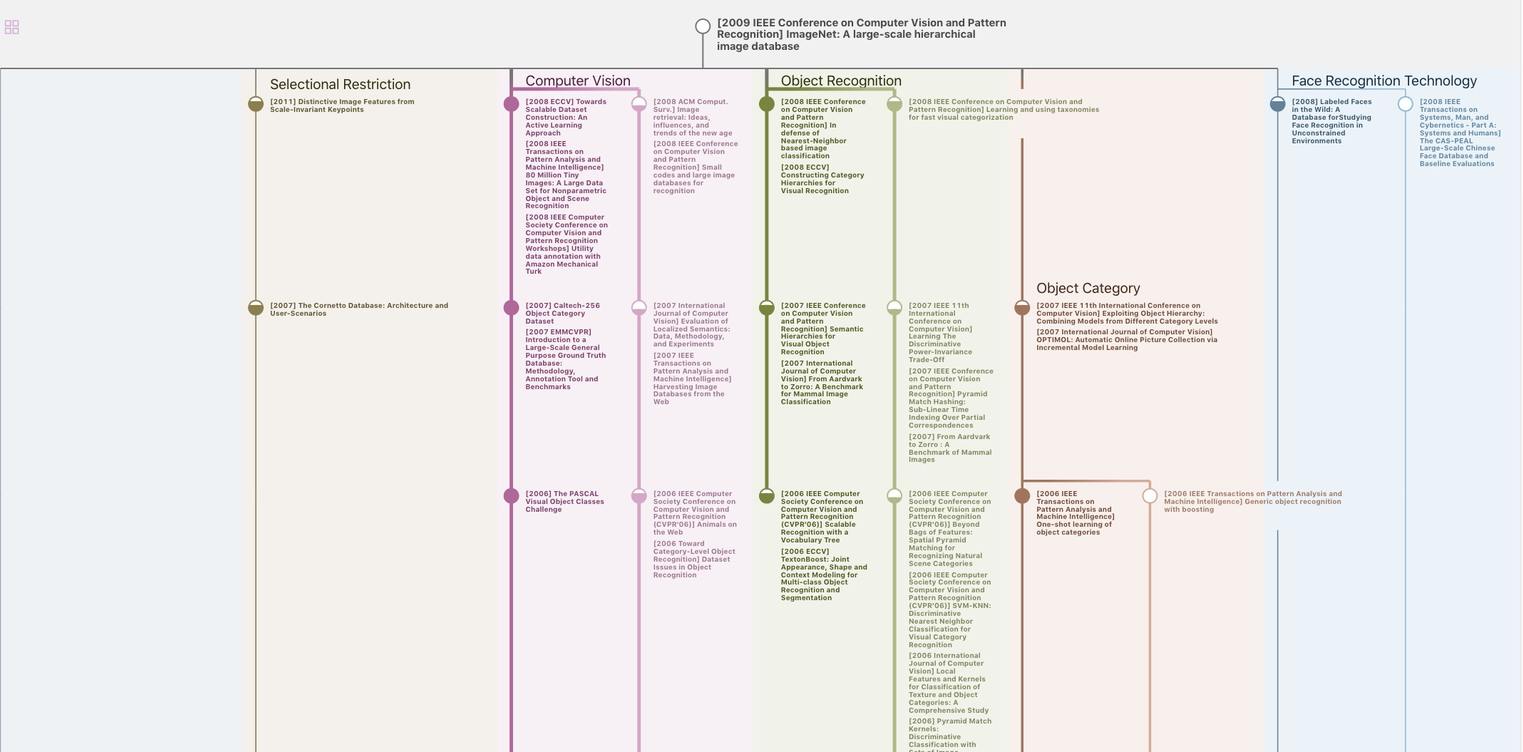
生成溯源树,研究论文发展脉络
Chat Paper
正在生成论文摘要