Capacity prediction and design optimization for laterally loaded monopiles in sandy soil using hybrid neural network and sequential quadratic programming
COMPUTERS AND GEOTECHNICS(2023)
摘要
Data driven methods have gained momentum in recent years in solving highly non-linear engineering problems that are challenging to solve using conventional methods. In this paper, we present a hybrid neural network model to predict the lateral response of large-diameter monopiles in multi-layered soil. The hybrid neural network consists of a mixture of convolutional and fully-connected layers, which capture the impacts of the soil profile, the pile geometry, and loading conditions on the lateral load response of monopiles. To train the neural network model, we produced data from high-fidelity three-dimensional (3D) finite element (FE) models that are validated against full-scale pile load tests. To ensure consistent model performance across the entire range of pile capacities considered in the dataset (ranging from approximately 100 kN to 100,000 kN), we utilize the relative error (percentage error) as the criterion for training the model. To achieve this goal, we explored six different combinations of data transformation methods (i.e., natural logarithm and root transformations) and cost functions. Among these models, the model trained with Mean Squared Error (MSE) using natural logarithm transformation yielded the most accurate and consistent predictions of the lateral capacities of monopiles. To demonstrate the strengths of the developed neural network model, it was used as a surrogate model to perform pile design optimization using sequential quadratic programming. In addition, a design example is provided to show how the developed method can be easily implemented.
更多查看译文
关键词
monopiles,sandy soil,hybrid neural network,design optimization
AI 理解论文
溯源树
样例
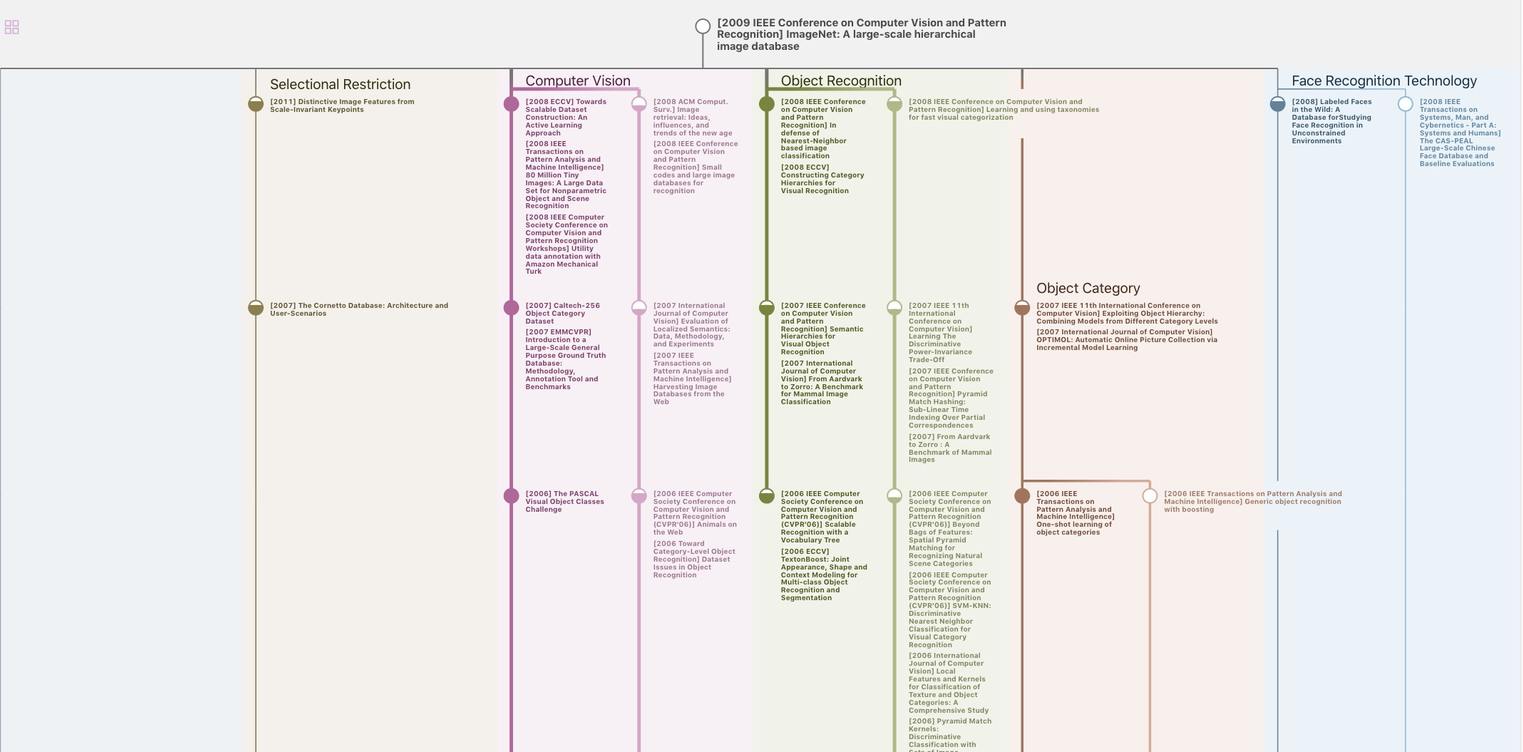
生成溯源树,研究论文发展脉络
Chat Paper
正在生成论文摘要