The Ear Unwrapper: A Maize Ear Image Acquisition Pipeline for Disease Severity Phenotyping
AGRIENGINEERING(2023)
摘要
Fusarium ear rot (FER) is a common disease in maize caused by the pathogen Fusarium verticillioides. Because of the quantitative nature of the disease, scoring disease severity is difficult and nuanced, relying on various ways to quantify the damage caused by the pathogen. Towards the goal of designing a system with greater objectivity, reproducibility, and accuracy than subjective scores or estimations of the infected area, a system of semi-automated image acquisition and subsequent image analysis was designed. The tool created for image acquisition, "The Ear Unwrapper", successfully obtained images of the full exterior of maize ears. A set of images produced from The Ear Unwrapper was then used as an example of how machine learning could be used to estimate disease severity from unannotated images. A high correlation (0.74) was found between the methods estimating the area of disease, but low correlations (0.47 and 0.28) were found between the number of infected kernels and the area of disease, indicating how different methods can result in contrasting severity scores. This study provides an example of how a simplified image acquisition tool can be built and incorporated into a machine learning pipeline to measure phenotypes of interest. We also present how the use of machine learning in image analysis can be adapted from open-source software to estimate complex phenotypes such as Fusarium ear rot.
更多查看译文
关键词
ear unwrapper,disease severity phenotyping,maize,disease severity
AI 理解论文
溯源树
样例
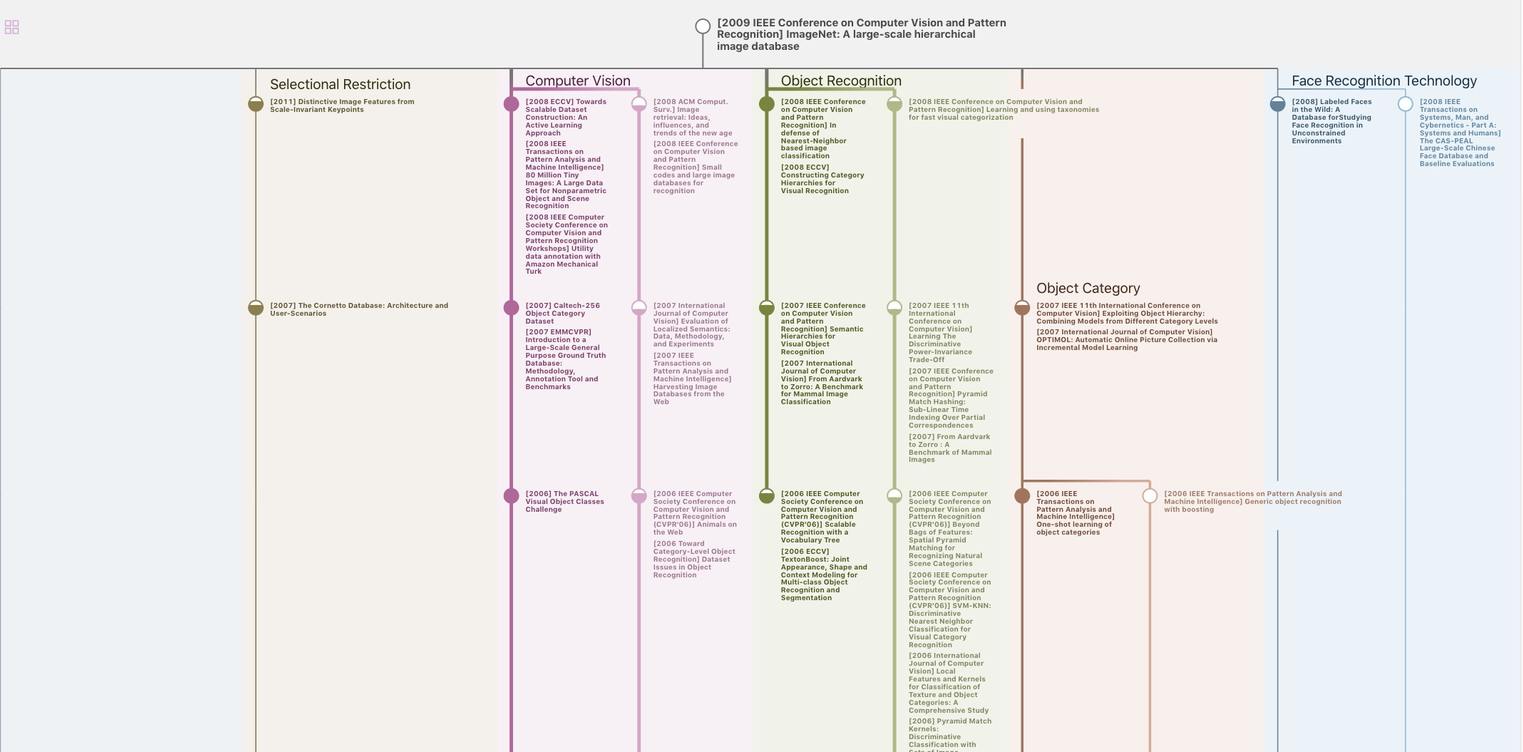
生成溯源树,研究论文发展脉络
Chat Paper
正在生成论文摘要