Exact Decoding of a Sequentially Markov Coalescent Model in Genetics
JOURNAL OF THE AMERICAN STATISTICAL ASSOCIATION(2023)
摘要
In statistical genetics, the sequentially Markov coalescent (SMC) is an important family of models for approximating the distribution of genetic variation data under complex evolutionary models. Methods based on SMC are widely used in genetics and evolutionary biology, with significant applications to genotype phasing and imputation, recombination rate estimation, and inferring population history. SMC allows for likelihood-based inference using hidden Markov models (HMMs), where the latent variable represents a genealogy. Because genealogies are continuous, while HMMs are discrete, SMC requires discretizing the space of trees in a way that is awkward and creates bias. In this work, we propose a method that circumvents this requirement, enabling SMC-based inference to be performed in the natural setting of a continuous state space. We derive fast, exact procedures for frequentist and Bayesian inference using SMC. Compared to existing methods, ours requires minimal user intervention or parameter tuning, no numerical optimization or E-M, and is faster and more accurate. Supplementary materials for this article are available online.
更多查看译文
关键词
sequentially markov coalescent model,exact decoding,genetics
AI 理解论文
溯源树
样例
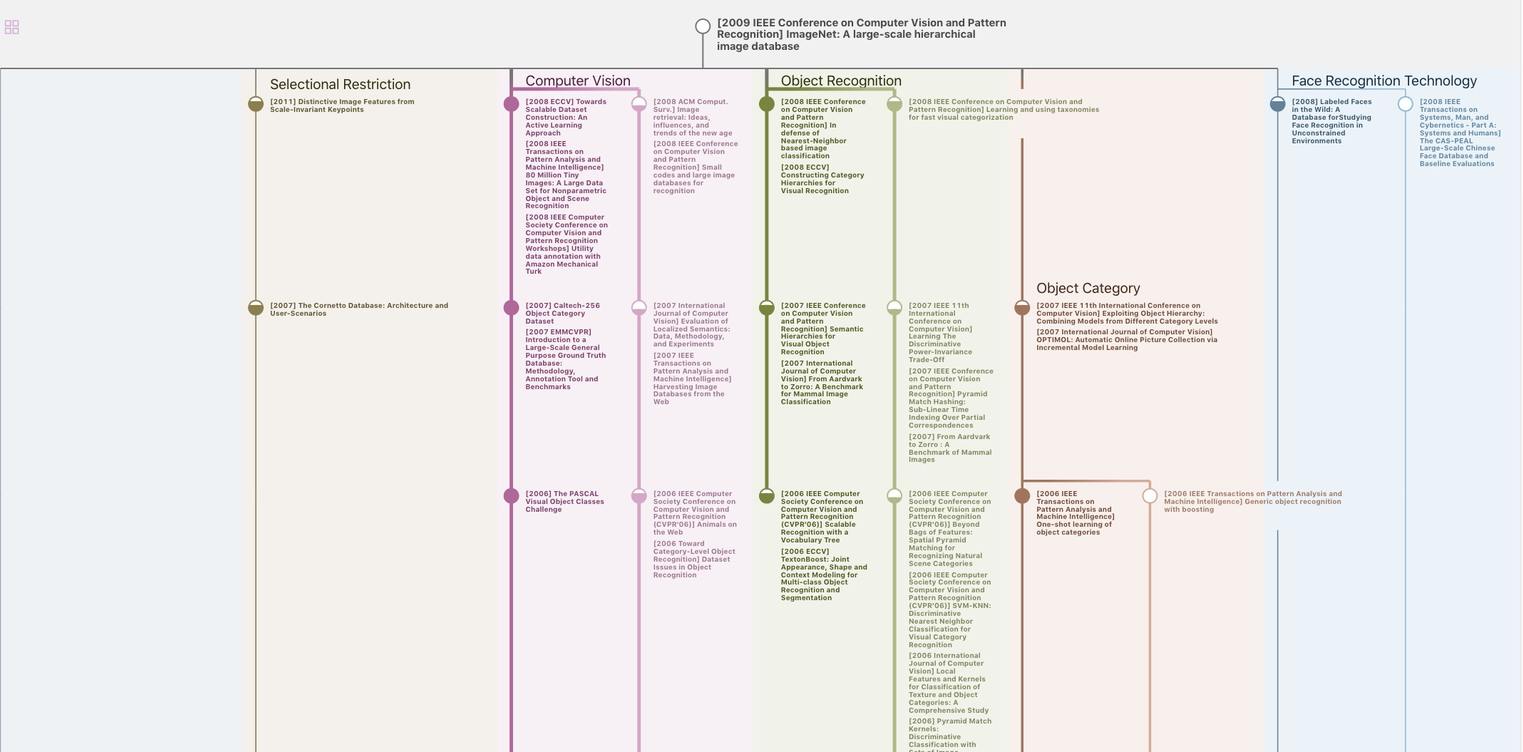
生成溯源树,研究论文发展脉络
Chat Paper
正在生成论文摘要