Unveiling the Unobservable: Causal Inference on Multiple Derived Outcomes
JOURNAL OF THE AMERICAN STATISTICAL ASSOCIATION(2023)
摘要
In many applications, the interest is in treatment effects on random quantities of subjects, where those random quantities are not directly observable but can be estimated based on data from each subject. In this article, we propose a general framework for conducting causal inference in a hierarchical data generation setting. The identifiability of causal parameters of interest is shown under a condition on the biasedness of subject level estimates and an ignorability condition on the treatment assignment. Estimation of the treatment effects is constructed by inverse propensity score weighting on the estimated subject level parameters. A multiple testing procedure able to control the false discovery proportion is proposed to identify the nonzero treatment effects. Theoretical results are developed to investigate the proposed procedure, and numerical simulations are carried out to evaluate its empirical performance. A case study of medication effects on brain functional connectivity of patients with Autism spectrum disorder (ASD) using fMRI data is conducted to demonstrate the utility of the proposed method. Supplementary materials for this article are available online.
更多查看译文
关键词
causal inference,unobservable,outcomes
AI 理解论文
溯源树
样例
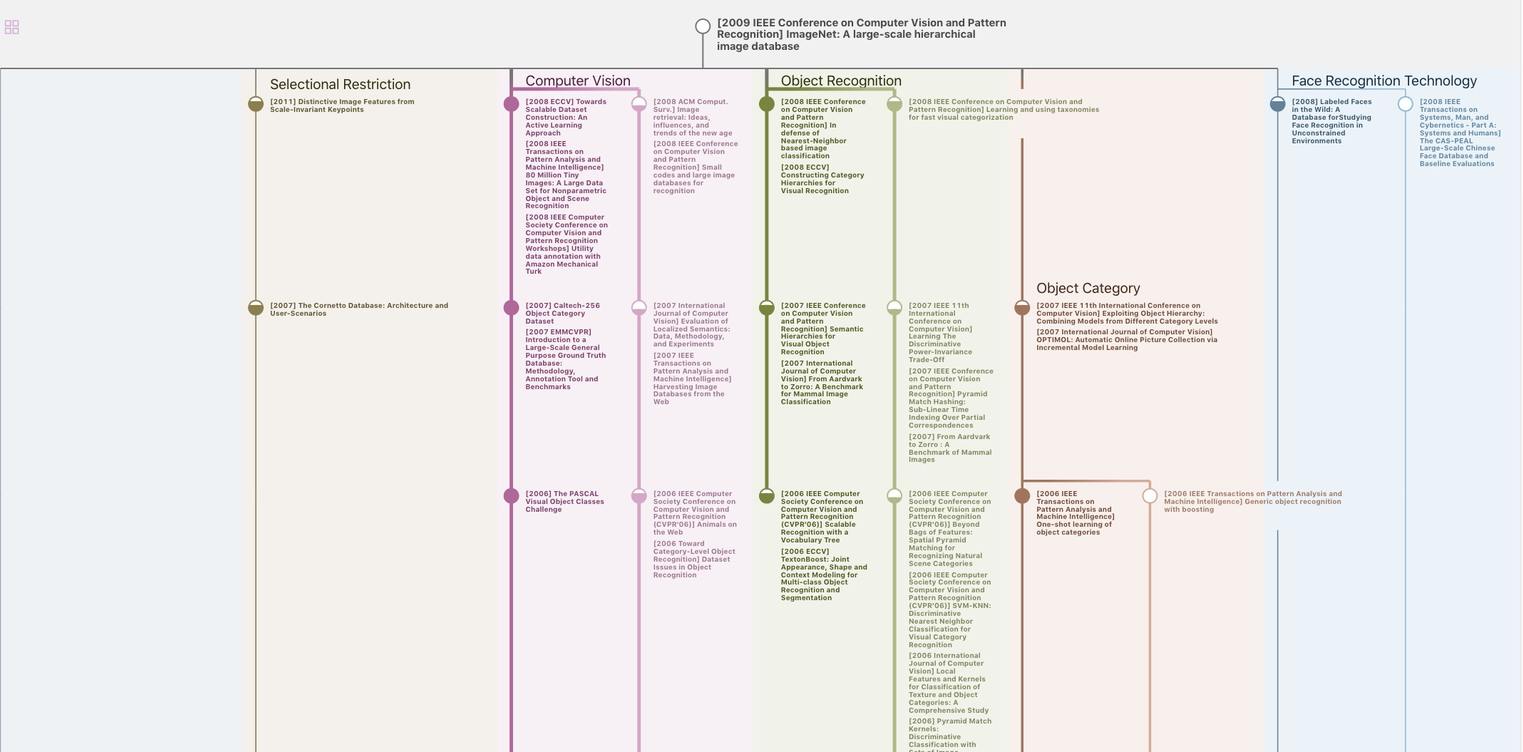
生成溯源树,研究论文发展脉络
Chat Paper
正在生成论文摘要