A Reinforcement Learning Based Large-Scale Refinery Production Scheduling Algorithm
IEEE TRANSACTIONS ON AUTOMATION SCIENCE AND ENGINEERING(2023)
摘要
Refinery production scheduling is a mixed-integer programming problem, which exists the issue of combinational explosion. Thus, solving a large-scale refinery production scheduling problem is time-consuming. This article proposes an approximate solution framework based on reinforcement learning (RL) for large-scale long-time refinery production scheduling problems to rapidly obtain a satisfactory solution. In the proposed algorithm, the Proximal Policy Optimization algorithm is used to process the continuous action. To address the cold start issue of RL in refinery scheduling problem, we present an initialization method for the actor of agent, which utilizes the operation knowledge of tractable small-scale problems to initialize the actor network, and the agent is trained in the environment of large-scale problems. Hence, the convergence of the RL algorithm is greatly accelerated. In addition, the product flowrate concept is used to express the state, making the scheduling agent scalable in terms of scheduling horizon. Experimental studies show, to large-scale refinery scheduling problems, the proposed algorithm can obtain better solutions than that of the CPLEX solver and the existing evolutionary algorithm in a much shorter solving time of the two methods.
更多查看译文
关键词
Large-scale optimization,reinforcement learning,refinery,scheduling
AI 理解论文
溯源树
样例
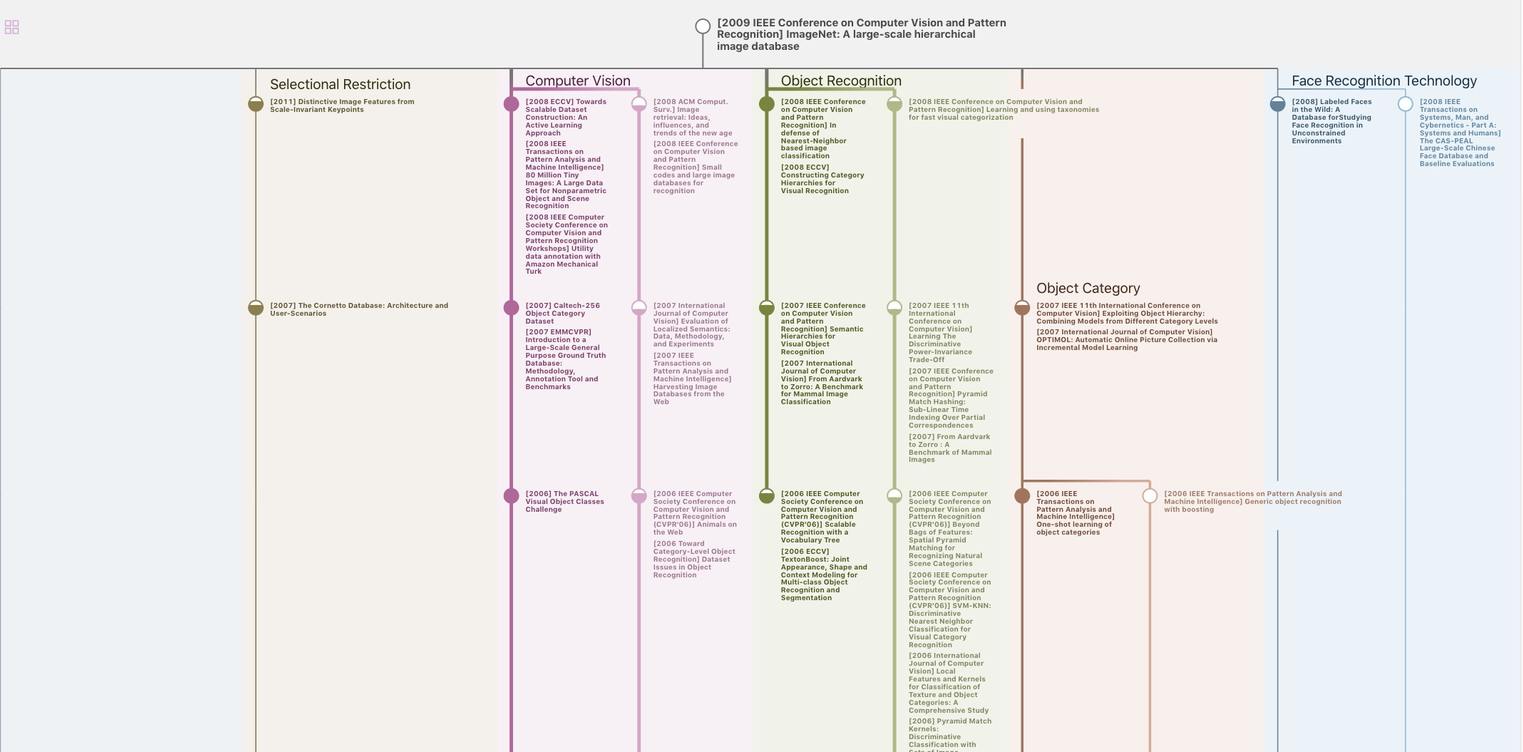
生成溯源树,研究论文发展脉络
Chat Paper
正在生成论文摘要