Specular Removal of Industrial Metal Objects Without Changing Lighting Configuration
IEEE TRANSACTIONS ON INDUSTRIAL INFORMATICS(2024)
摘要
In an industrial environment, measuring and reconstructing metal objects using computer vision methods can be affected by surface highlight reflections, leading to inaccurate results. In this article, we propose a novel network with broad applicability for removing highlight reflections based on dynamic highlight masks, which is suitable for industrial metal highlight images where a baseline image with no highlight reflections is not available. First, we use a pretrained model to learn the highlight features of the metal surface, and then construct an adaptive dynamic highlight soft mask that allows texture features in highlight regions to be preserved while removing highlights. Second, we adjust the shape and shift of convolution operations using an adaptive highlight mask to better match the metal surface structure and avoid unnatural transitions at the edges of highlights in partially convolutional networks. We conducted quantitative evaluations on synthetic and real-world highlight datasets to demonstrate the effectiveness of our method. Compared with the state-of-the-art methods, our method shows improvements of over 0.4 in terms of correlation and Bhattacharyya distance of the histogram curves for synthesized highlight datasets. In terms of nonhighlight region invariance of real industrial highlight datasets, our method shows improvements of over 8, 0.1, and 23 points in terms of peak signal-to-noise ratio, structural similarity, and mean squared error, respectively.
更多查看译文
关键词
Highlight detection,highlight removal,in-dustrial scene,metal objects,specular reflection
AI 理解论文
溯源树
样例
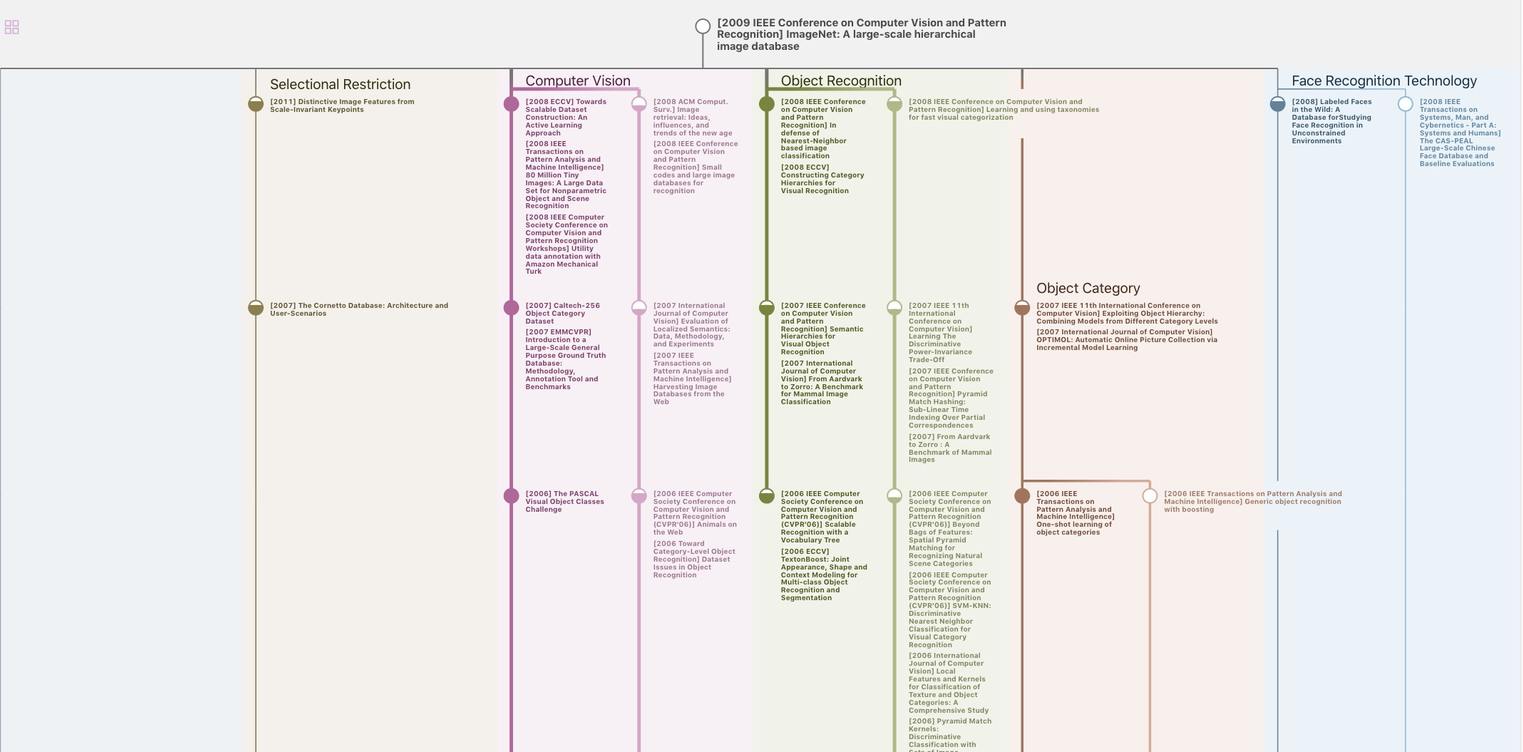
生成溯源树,研究论文发展脉络
Chat Paper
正在生成论文摘要