Bayesian denoising of structured sources and its implications on learning-based denoising
INFORMATION AND INFERENCE-A JOURNAL OF THE IMA(2023)
摘要
Denoising a stationary process (X-i)(i is an element of)Z corrupted by additive white Gaussian noise (Z(i))(i is an element of)Z is a classic, well-studied and fundamental problem in information theory and statistical signal processing. However, finding theoretically founded computationally efficient denoising methods applicable to general sources is still an open problem. In the Bayesian set-up where the source distribution is known, a minimum mean square error (MMSE) denoiser estimates X-n from noisy measurements Y-n as X-<^>(n)=E[X-n|Y-n]. However, for general sources, computing E[X-n|Y-n] is computationally very challenging, if not infeasible. In this paper, starting from a Bayesian set-up, a novel denoising method, namely, quantized maximum a posteriori (Q-MAP) denoiser is proposed and its asymptotic performance is analysed. Both for memoryless sources, and for structured first-order Markov sources, it is shown that, asymptotically, as sigma(2)(z) (noise variance) converges to zero, 1/sigma E-2(z)[(X-i - X-<^>QMAP)(2)] converges to the information dimension of the source. For the studied memoryless sources, this limit is known to be optimal. A key advantage of the Q-MAP denoiser, unlike an MMSE denoiser, is that it highlights the key properties of the source distribution that are to be used in its denoising. This key property leads to a new learning-based denoising approach that is applicable to generic structured sources. Using ImageNet database for training, initial simulation results exploring the performance of such a learning-based denoiser in image denoising are presented.
更多查看译文
关键词
bayesian denoising,structured sources,learning-based
AI 理解论文
溯源树
样例
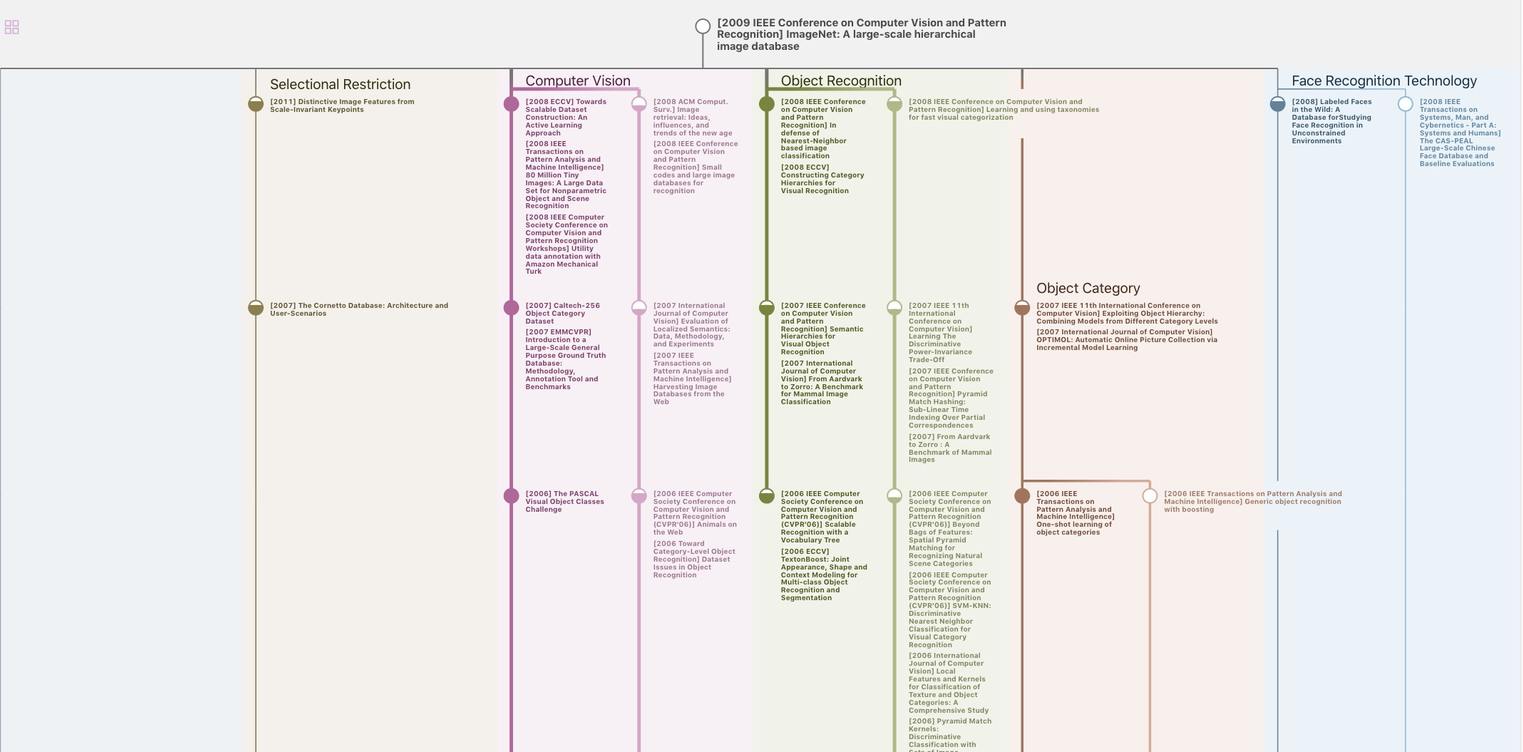
生成溯源树,研究论文发展脉络
Chat Paper
正在生成论文摘要