ClimateFiller: A Python framework for climate time series gap-filling and based on artificial and multi-source data
SOFTWARE IMPACTS(2023)
摘要
Clean weather time series is the primary ingredient for the successful modeling of any process in the soil plant-atmosphere continuum. However, measured meteorological data are often associated with gaps due to various reasons, such as eventual sensor malfunctioning, power outages, and data transmission errors. Thus, meteorological data needs to be quality-controlled prior to any further processing. To this end, we developed a Python framework that automatically detects missing values and uses ERA5-Land reanalysis data and machine learning to fill in the gaps. The resulting RMSE values indicate a good consistency between the estimated and real in-situ data (0.73 degrees C, 26.07 W m2, and 11.57%, for air temperature, global solar radiation, and air relative humidity, respectively.). Additionally, the framework implements different data-driven methods to detect and fill in outliers and apply physics constraints to the data.
更多查看译文
关键词
Missing data, Data quality control, Weather time series gap-filling, Machine learning, ERA5-Land, Python
AI 理解论文
溯源树
样例
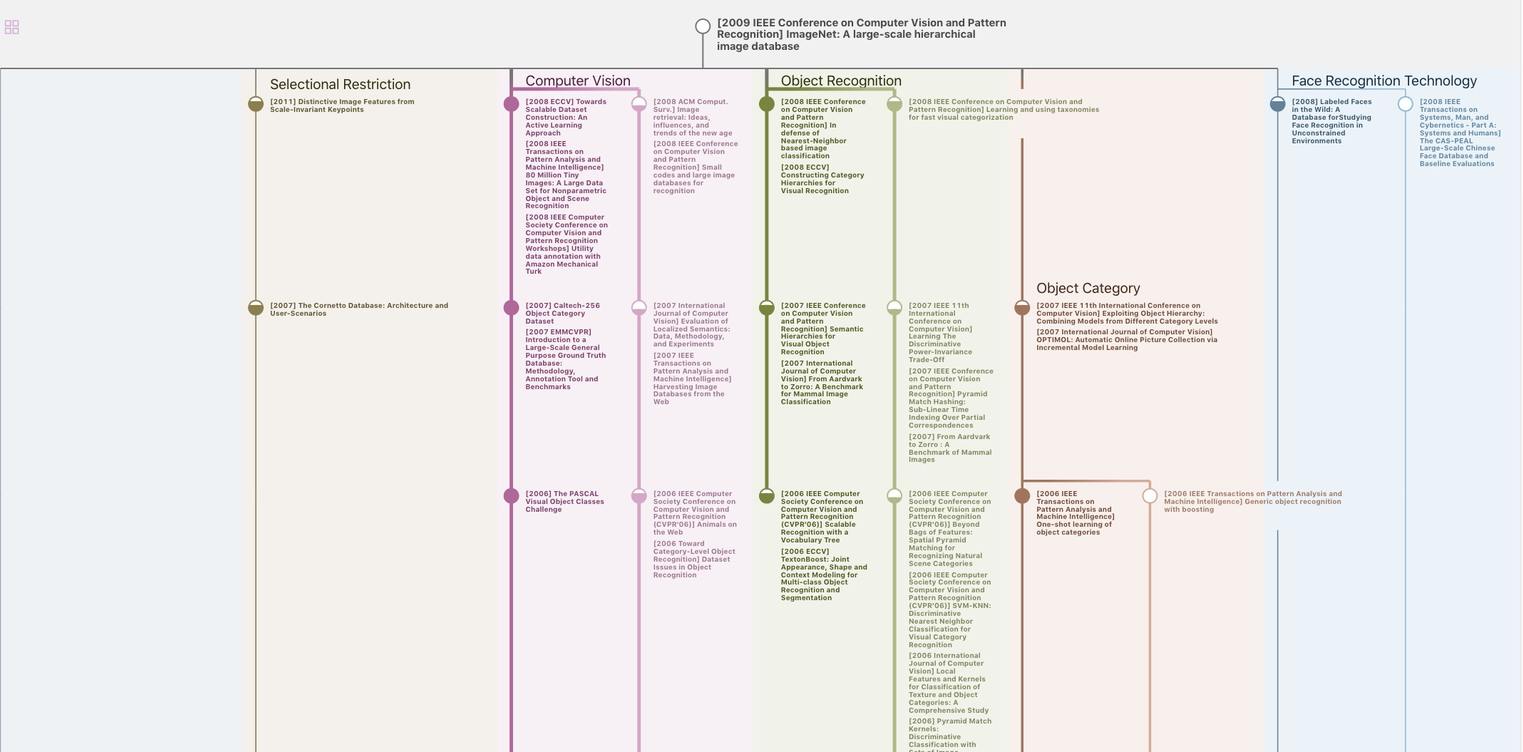
生成溯源树,研究论文发展脉络
Chat Paper
正在生成论文摘要