Online Self-Learning Stochastic Configuration Networks for Nonstationary Data Stream Analysis
IEEE TRANSACTIONS ON INDUSTRIAL INFORMATICS(2024)
摘要
Stochastic configuration networks (SCNs) have been widely used as predictive models to model complex nonlinear systems due to their advantages in terms of easy-to-implement, fast learning speed, and universal approximation property. In many fields, however, the data generated by nonlinear systems are often characterized by dynamic time series and nonstationary, which result in the learner model with poor generalization performance. This article presents an online self-learning stochastic configuration network to improve the continuous learning ability of SCNs to model nonstationary data streams. The method can autonomously adjust the parameters and structure of the network according to the real-time arriving data streams. Specifically, we use a recursive learning mechanism to update the network parameters online based on the data acquired in real time. In addition, the structure of the SCNs is dynamically adjusted by sensitivity analysis and stochastic configuration algorithm to improve the adaptive and continuous learning capability of the network. A series of comparisons are carried out over two benchmark datasets and one practical industrial case from the wastewater treatment process to verify the effectiveness of the proposed method. Experimental results demonstrate that the proposed method has good potential for nonstationary data stream analysis.
更多查看译文
关键词
Nonstationary data streams,online sequential learning,self-learning,stochastic configuration networks (SCNs),wastewater treatment process
AI 理解论文
溯源树
样例
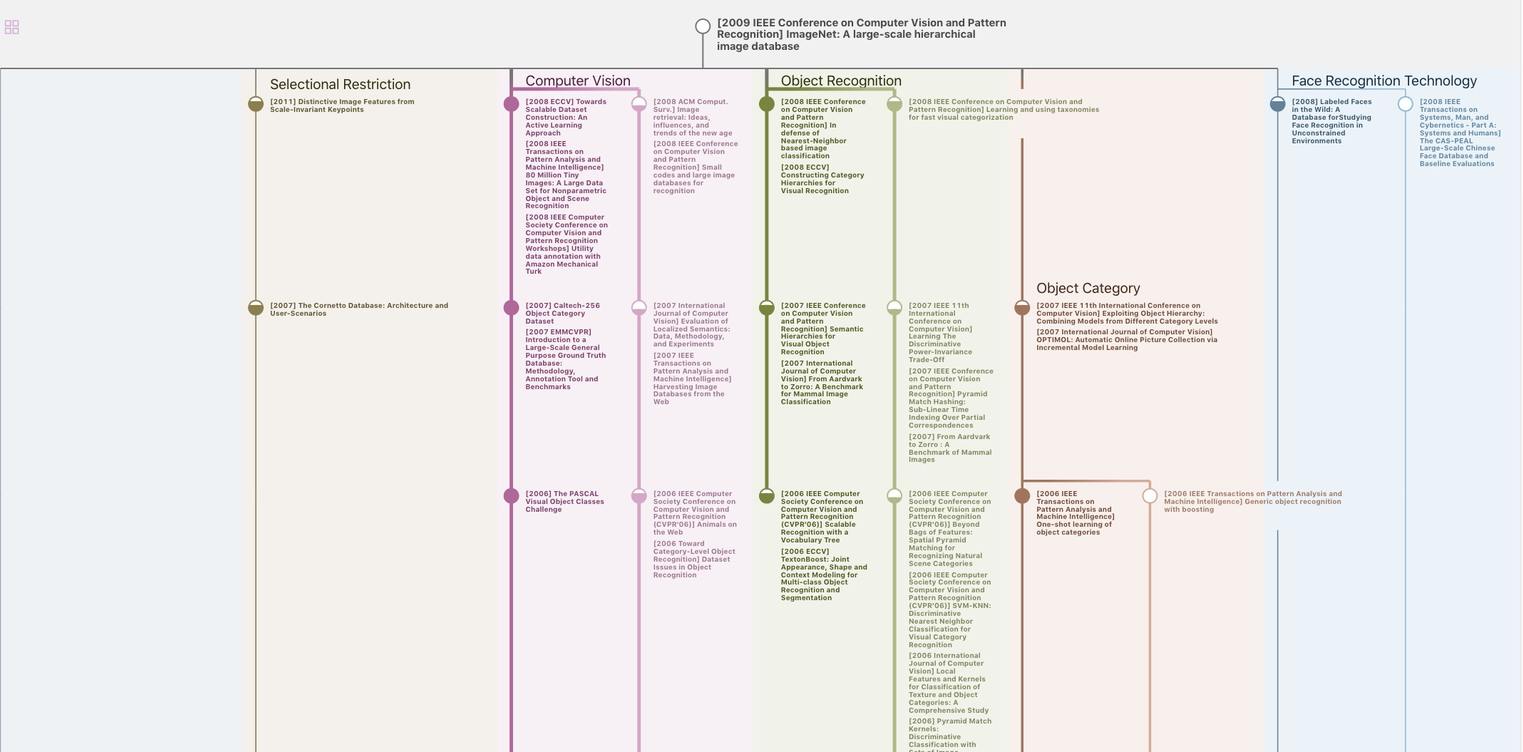
生成溯源树,研究论文发展脉络
Chat Paper
正在生成论文摘要