Application of advanced machine learning algorithms and geospatial techniques for groundwater potential zone mapping in Gambela Plain, Ethiopia
HYDROLOGY RESEARCH(2023)
摘要
Groundwater availability is one of the key anxieties in most semi-arid regions of Ethiopia. The purpose of this study was to investigate the groundwater potential zone map of the alluvial plain of Gambela. The study applied analytic hierarchy process (AHP) models with four different machine learning algorithms: random forest classifier (RFC), gradient boosting classifier (GBC), decision tree classifier (DTC), and K-neighbor classifier (KNC). The features that are used as predictors include geology, geomorphology, slope, soil, lineament density, drainage density, land use and land cover (LULC), normalized difference vegetation index (NDVI), topographic wetness index (TWI), topographic roughness index (TRI), and rainfall. The final output of the groundwater potential zone was classified as low, moderate, high, and very high potential zones. The authentication through receiver operating curve (ROC) shows 78.2, 93.4, 92.5, 72.4, and 87.7% values of area under the curve (AUC) for AHP, RFC, GBC, DTC, and KNC, respectively. The results show that RFC and GBC are the best GWPZ map estimator. The study also shows that rainfall and geomorphology are the primary factors influencing the GWPZ. The outcome might promote improved management alternatives in other areas of the country with a comparable climate.
更多查看译文
关键词
Gambela Plain, groundwater potential, machine learning, random forest, remote sensing, ROC
AI 理解论文
溯源树
样例
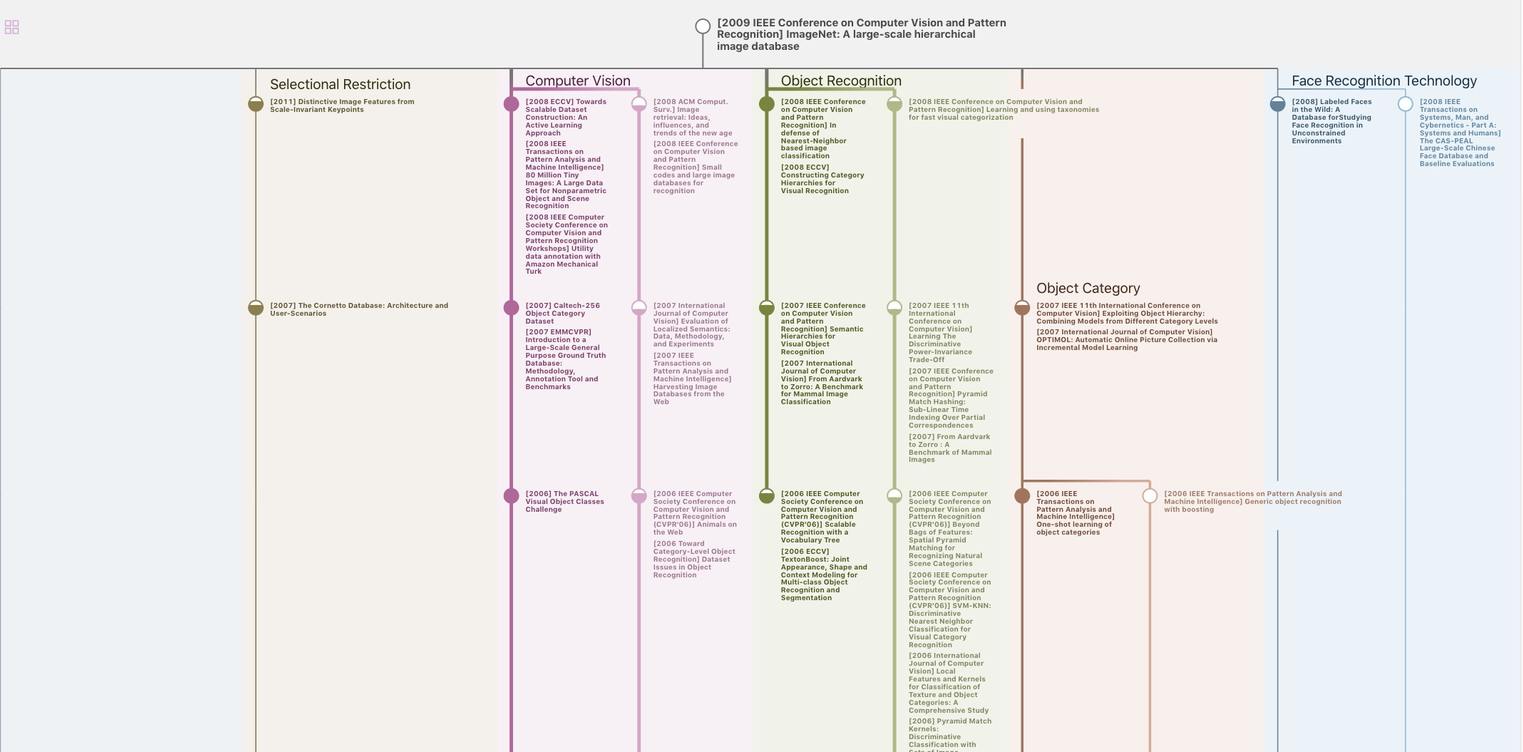
生成溯源树,研究论文发展脉络
Chat Paper
正在生成论文摘要