Geographically weighted accuracy for hard and soft land cover classifications: 5 approaches with coded illustrations
INTERNATIONAL JOURNAL OF REMOTE SENSING(2023)
摘要
This paper examines different geographically weighted (GW) approaches for calculating spatially distributed measures of accuracy / uncertainty, consolidating current approaches and proposing 2 new ones. GW frameworks use a moving window or kernel to extract and weight data subsets, from which local (ie spatially distributed) statistics or metrics are calculated. A validation dataset with hard and soft classifications is used to illustrate the approaches. It contains observed field survey data (also commonly derived from higher resolution imagery), and predicted data from a fuzzy c-means classification. The hard classes were used to estimate spatially distributed measures of overall, user's and producer's accuracies in two ways. First, by conceptualising them as probabilities to be estimated from generalised linear regression models (GLMs), extended into Geographically Weighted GLMs. Second, by constructing local GW correspondence matrices and then calculating local accuracy measures from these. The soft classes were used to calculate per-class measures of fuzzy certainty from the absolute difference between predicted and observed fuzzy memberships. Then, a novel fuzzy certainty logic is proposed and used to create fuzzy confusion matrices and per-class measures of fuzzy omission and commission error, supporting measures of fuzzy user's and producer's certainties. These were extended to the GW case to generate spatially distributed measures. Finally, the soft classifications were conceptualised as compositional data and measures of difference were estimated using Aitchison distances. In each case, the local hard and soft accuracy and certainty measures were interpolated over a 1 km grid to estimate accuracy surfaces. The context for this review is the increasing operational use of training and validation data, often with high numbers of records, containing both hard and soft classes. The data and R code used to undertake all the analyses in this paper are provided, supporting more nuanced analyses of such data.
更多查看译文
关键词
Classification accuracy, geographically weighted regression, logistic regression, fuzzy classification
AI 理解论文
溯源树
样例
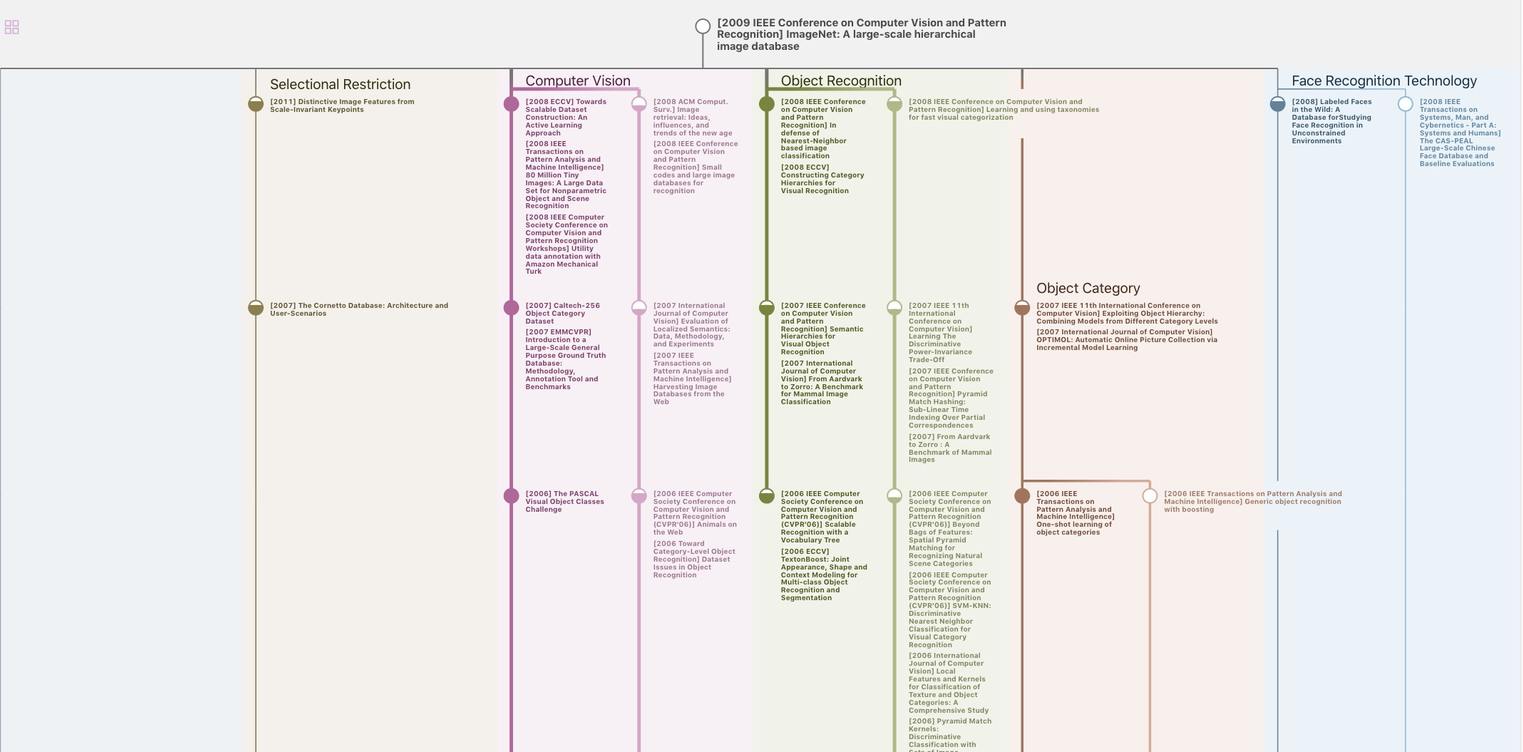
生成溯源树,研究论文发展脉络
Chat Paper
正在生成论文摘要