Initial condition based real time classification of power quality disturbance using deep convolution neural network with bidirectional long short-term memory
IET GENERATION TRANSMISSION & DISTRIBUTION(2023)
摘要
The accurate classification of power quality disturbances (PQDs) is crucial for advancing real-time monitoring and classification systems within the modern power grid. The proposed system must ensure dependable, safeguarded, and stable operating conditions amidst diverse power quality issues. This paper presents an approach to classifying power quality disturbances using a deep learning model that synergizes deep convolutional neural networks (DCNN) and Bidirectional Long Short-Term Memory (BiLSTM). This amalgamation effectively extracts and classifies disturbance signals in real time, grounded on noise levels. The initial feature extraction from the signal is accomplished through a time-frequency matrix. Subsequently, secondary extraction employs the BiLSTM layer to intricately and significantly classify disturbances in the power signal. This aids in transforming high-dimensional matrices into a reduced set for enhanced performance. The detailed classification is facilitated by the softmax layer. The simulation results support the power quality evaluations under varied constraints and underscore the substantial classification of power quality disturbances through the DCNN-BiLSTM algorithm, in comparison to alternative classification algorithms in terms of computational speed and accuracy.
更多查看译文
关键词
power quality disturbance,real time classification,deep convolution,neural network
AI 理解论文
溯源树
样例
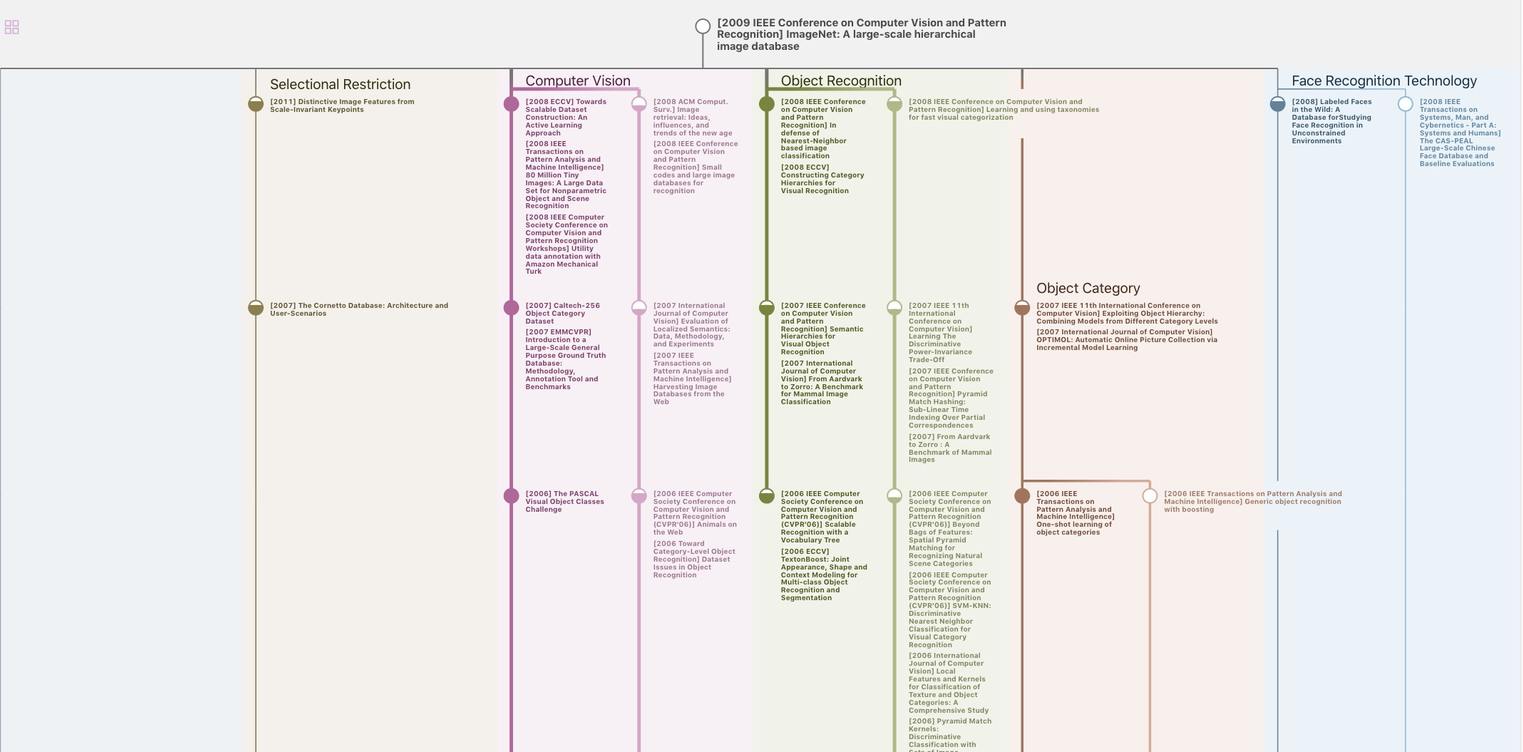
生成溯源树,研究论文发展脉络
Chat Paper
正在生成论文摘要