Dynamic Mobile Sink Path Planning for Unsynchronized Data Collection in Heterogeneous Wireless Sensor Networks
IEEE SENSORS JOURNAL(2023)
摘要
In heterogeneous wireless sensor networks (HWSNs), heterogeneous sensors may follow various data-generating distributions, which makes data collection a very challenging task. Although mobile sinks (MSs) are widely used to collect data from wireless sensors, existing MS-assisted approaches are often based on the assumption of synchronized data, for example, all sensors generate data at the same time, in which data are considered delay-tolerant and -sensitive data according to delay limits, while the generation time of data is ignored. This article focuses on unsynchronized data collection for HWSNs, in which unsynchronized data generation of sensors is allowed as real as actual monitoring applications. First, to reflect the timeliness of data, we use a rigid collection window to represent the lifetime of sensing data to refine the visiting time of the MS. Second, a graph attention network (GAT) structure is adopted to describe node locations, accessible paths, and data with collection windows for path planning. Third, a new deep reinforcement learning (DRL)-based MS path planning (MSPP) framework is proposed to tackle the path of the MS by minimizing total energy cost while satisfying data lifetime constraints. MSPP first uses the Target Selector module to plan the moving targets and adopts the MS Controller module to control MS mobility for achieving fast convergence and better optimality. Finally, extensive simulations show that our scheme provides explicit data collection guarantees and minimum energy consumption.
更多查看译文
关键词
Deep reinforcement learning (DRL),heterogeneous wireless sensor networks (HWSNs),mobile sink (MS),path planning,unsynchronized data collection
AI 理解论文
溯源树
样例
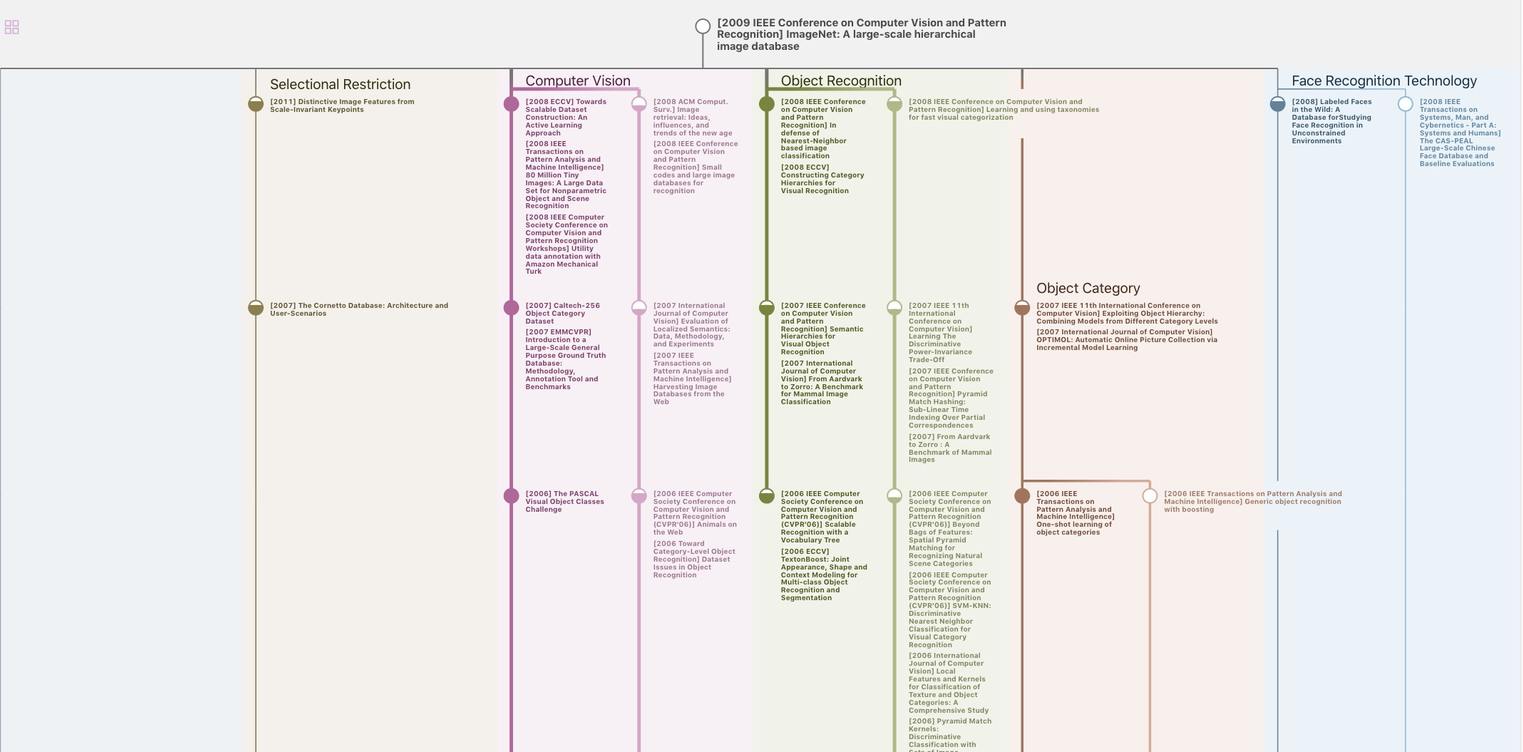
生成溯源树,研究论文发展脉络
Chat Paper
正在生成论文摘要