Fast halftoning policy network with error feedback
JOURNAL OF ELECTRONIC IMAGING(2023)
摘要
Deep learning-based halftoning methods have demonstrated their abilities in dithering images with desired blue-noise properties. However, tons of parameters and computations come along with the 2D convolutional neural networks (CNNs), which hinder their practical usage, particularly in the cost-sensitive printing imaging system equipped with low-end computing devices. We devise a fast halftoning policy network to alleviate the problem. Motivated by classic error diffusion algorithms, we introduce an error feedback mechanism to model the dependencies between halftone lines. Consequently, the problem collapses to 1D deep halftoning within each line, which simplifies the learning task. Furthermore, a very simple convolutional layer is applied to capture the texture details from the continuous-tone image. We find that the proposed halftoning model can stably converge under the reinforcement learning-based framework. Experiments show that our model achieves a 10.6x speedup with only 1.7% parameters compared to a previous 2D CNN-based work while maintaining similar halftone quality. We have also conducted experiments on a digital signal processor to demonstrate how the proposed model saves expensive off-chip memory accesses and improves processing throughput in real scenarios. (c) 2023 SPIE and IS&T
更多查看译文
关键词
halftones,printing,real-time imaging,image processing
AI 理解论文
溯源树
样例
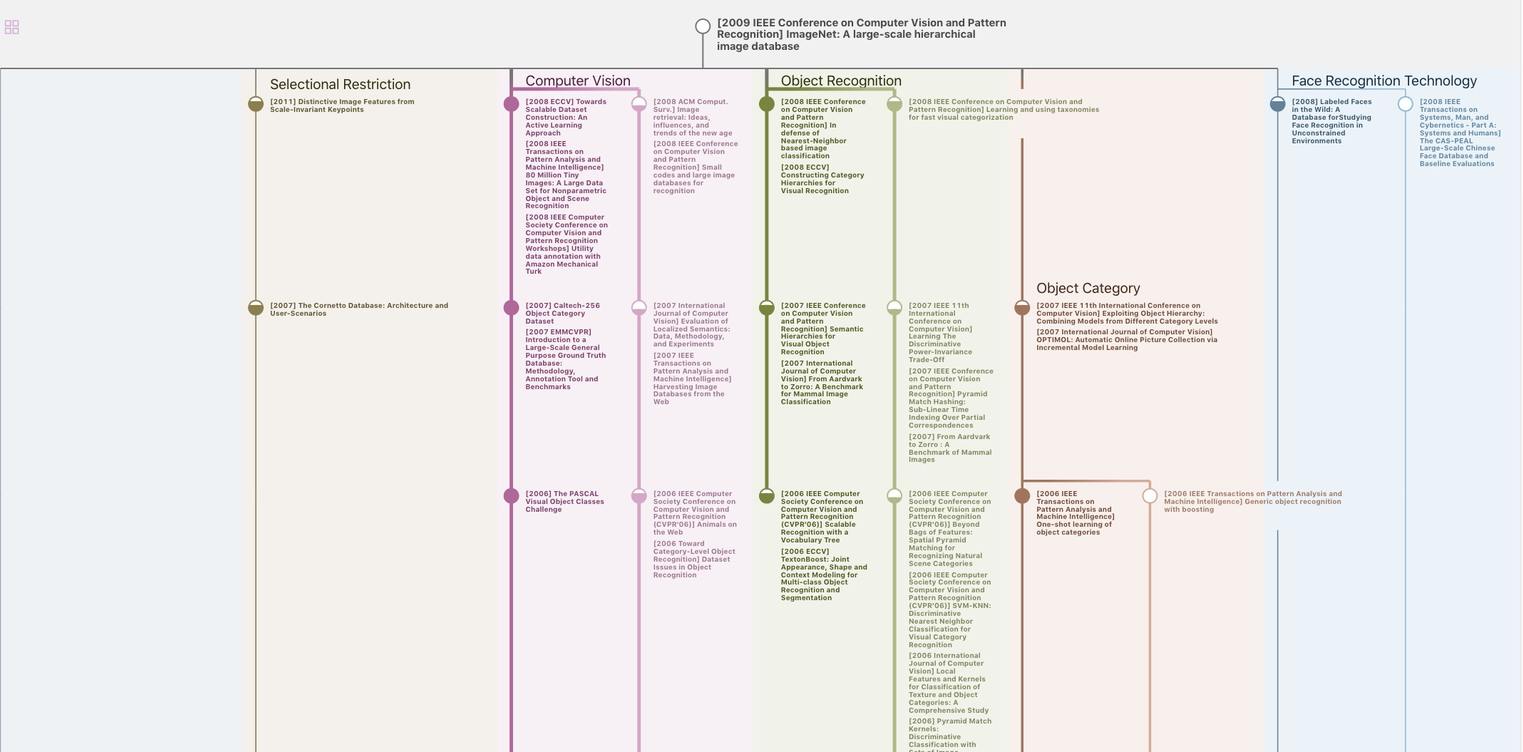
生成溯源树,研究论文发展脉络
Chat Paper
正在生成论文摘要