Application of Machine Learning Techniques to Improve Multi-Radar Mosaic Precipitation Estimates in Shanghai
ATMOSPHERE(2023)
摘要
In this study, we applied an explainable machine learning technique based on the LightGBM method, a category of gradient boosting decision tree algorithm, to conduct a quantitative radar precipitation estimation and move to understand the underlying reasons for excellent estimations. By introducing 3D grid radar reflectivity data into the LightGBM algorithm, we constructed three LightGBM models, including 2D and 3D LightGBM models. Ten groups of experiments were carried out to compare the performances of the LightGBM models with traditional Z-R relationship methods. To further assess the performances of the LightGBM models, rainfall events with 11,483 total samples during August-September of 2022 were used for statistical analysis, and two heavy rainfall events were specifically chosen for the spatial distribution evaluation. The results from both the statistical analysis and spatial distribution demonstrate that the performance of the LightGBM 3D model with nine points is the best method for quantitative precipitation estimation in this study. Through analyzing the explainability of the LightGBM models from Shapley additive explanations (SHAP) regression values, it can be inferred that the superior performance of the LightGBM 3D model is mainly attributed to its consideration of the rain gauge station attributes, diurnal variation characteristics, and the influence of spatial offset.
更多查看译文
关键词
quantitative precipitation estimation,Z-R relationship,LightGBM,radar reflectivity,SHAP
AI 理解论文
溯源树
样例
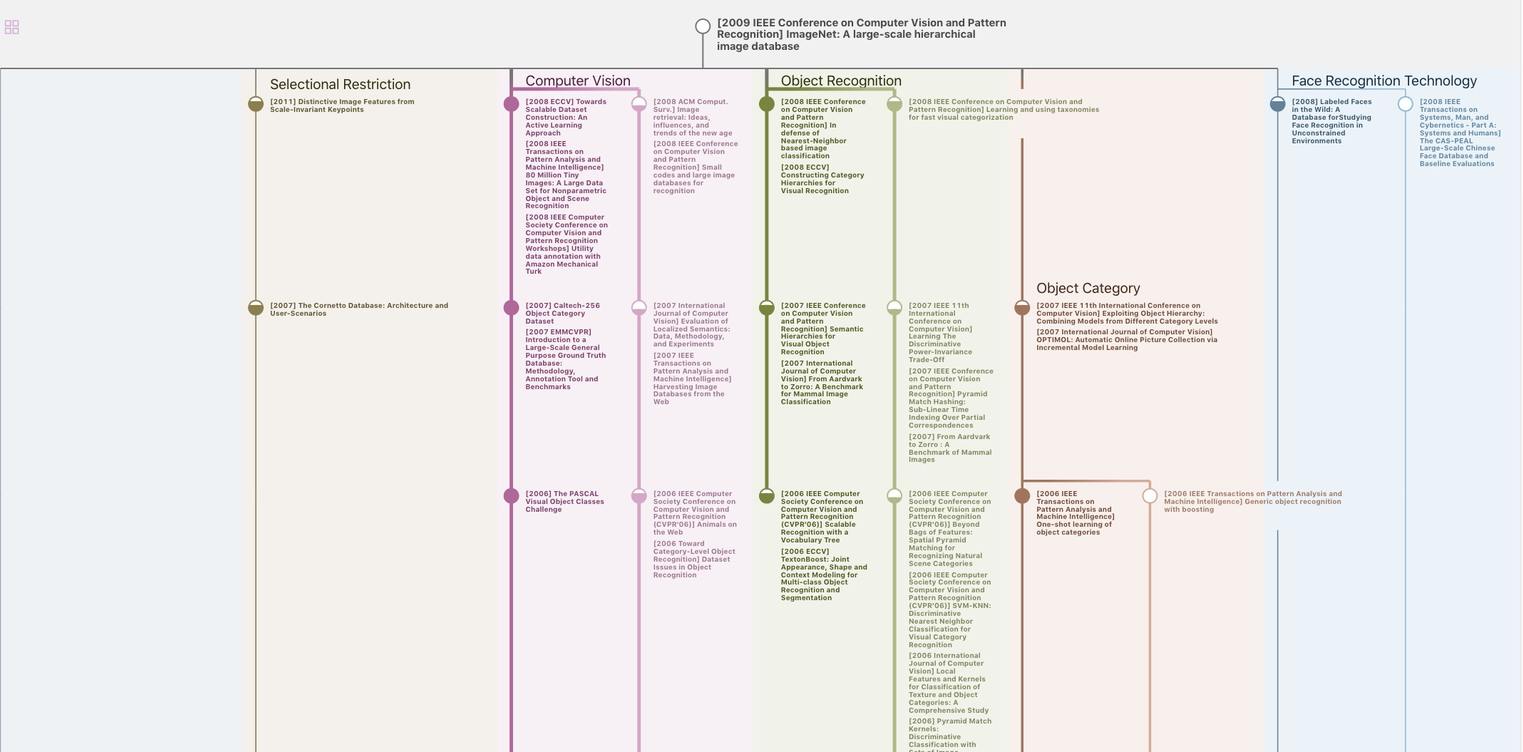
生成溯源树,研究论文发展脉络
Chat Paper
正在生成论文摘要