An Analysis of Temporal Features in Multivariate Time Series to Forecast Network Events
APPLIED SCIENCES-BASEL(2023)
摘要
Analyzing network traffic over time is crucial for understanding the changes in network activity. To properly examine network traffic patterns over time, multiple network events in each timestamp need to be converted to time series data. In this study, we propose a new approach to transform network traffic data into time series formats by extracting temporal features to analyze normal/attack patterns. The normal patterns indicate network traffic occurred without any intrusion-related activities, whereas the attack patterns denote potential threats that deviate from the normal patterns. To evaluate the features, long short-term memory (LSTM) is applied to forecast multi-step network normal and attack events. Visual analysis is also performed to enhance the understanding of key features in the network. We compared the performance differences using time scales of 60 and 120 s. Upon evaluation, we found that the temporal features extracted with the 60 s time scale exhibited better performance in forecasting future network events.
更多查看译文
关键词
multivariate time series,temporal features,forecast,events,network
AI 理解论文
溯源树
样例
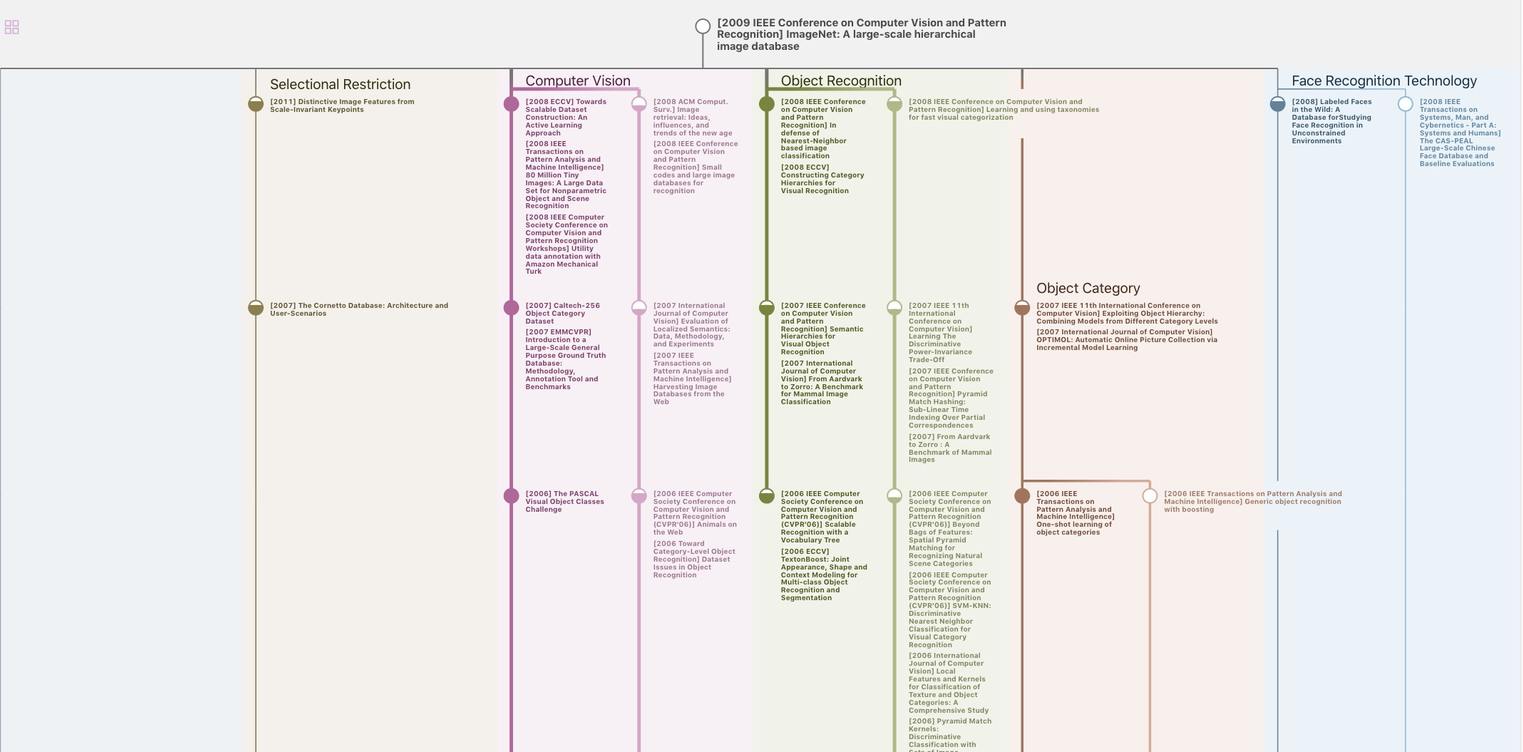
生成溯源树,研究论文发展脉络
Chat Paper
正在生成论文摘要